Today's digital landscape offers a vast sea of information, encompassing not only text, but also images and videos. Traditional enterprise search engines were primarily designed for text-based queries, and often fall short when it comes to analyzing visual content. However, with a combination of natural language processing (NLP) and multimodal embeddings, a new era of search is emerging that lets your customers search for an image or video — or information within it — in the same way they would with text-based content.
In this blog, we showcase a demo for performing text search on images, videos, or both using a powerful multimodal embedding model that’s specifically designed for cross-modal semantic search scenarios such as searching images using text, or finding text in images based on a given query. Multimodal embedding is the key to accomplishing these tasks.
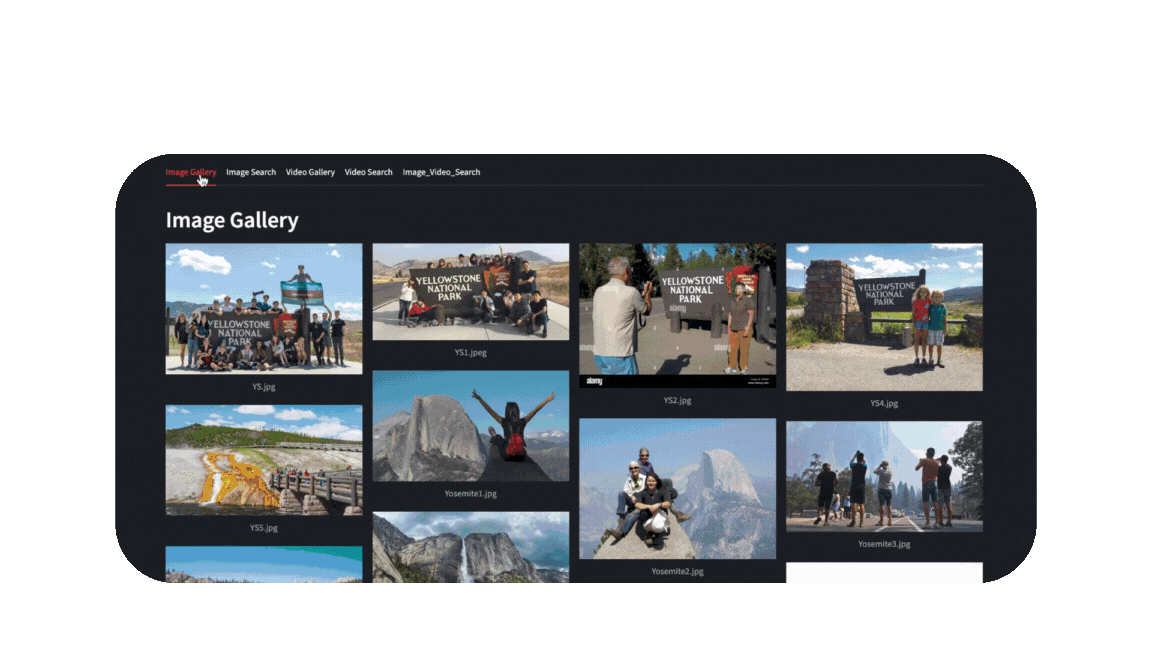
Our demo performs text to image search, text to video search, text to image and video combined search
Let's see how this works!
A solution for converged image, video, and text search
The architecture leverages Google Cloud Storage for storing media files, with BigQuery object tables referencing these files. A multimodal embedding model generates semantic embeddings for the images and videos, which are then indexed in BigQuery for efficient similarity search, enabling seamless cross-modal search experiences.
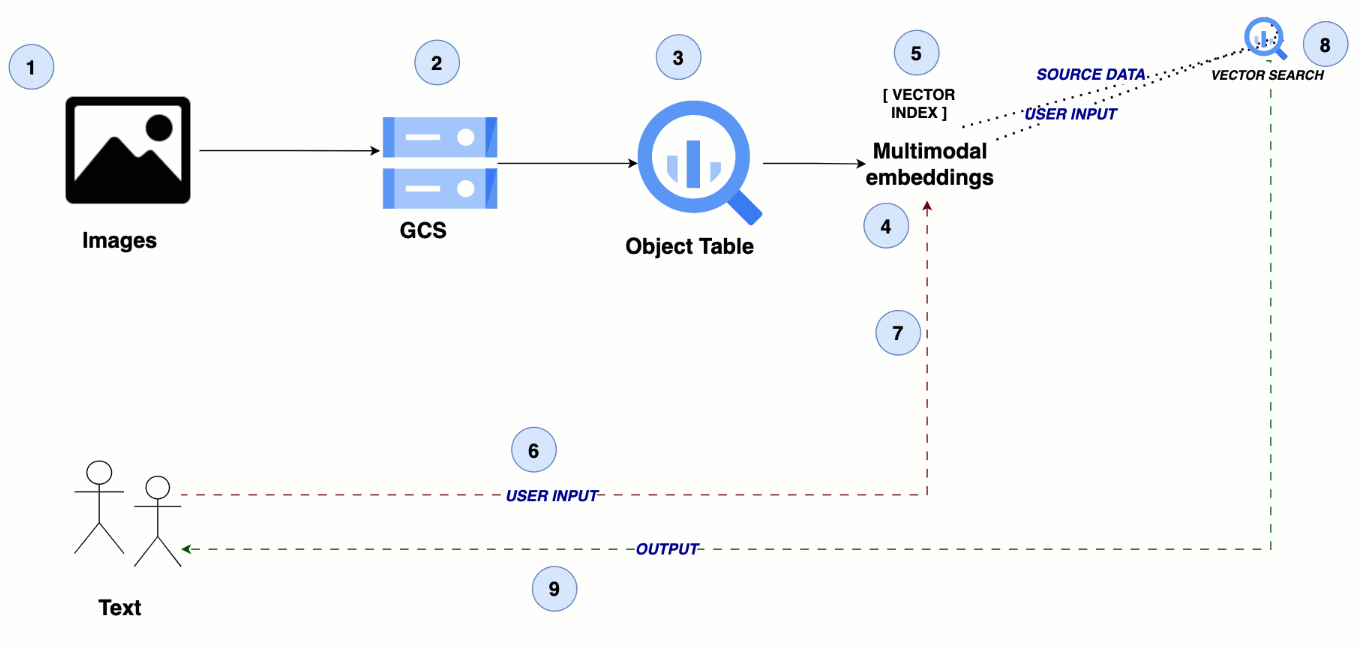
From text to visuals: Multimodal search for images and videos
To implement a similar solution, follow the steps below.
Steps 1 - 2: Upload image and video data to Cloud Storage
Upload all image and video files to a Cloud Storage bucket. For the demo, we’ve downloaded some images and videos from Google Search that are available on GitHub. Be sure to remove the README.md file before uploading them to your Cloud Storage bucket.
Prepare your media files:
Using your own data, collect all the images and video files you plan to work with.
Ensure the files are organized and named appropriately for easy management and access.
Upload data to Cloud Storage:
Create a Cloud Storage bucket, if you haven't already.
Upload your media files into the bucket. You can use the Google Cloud console, the
gsutil
command-line tool, or the Cloud Storage API.Verify that the files are uploaded correctly and note the bucket's name and path where the files are stored (e.g.,
gs://your-bucket-name/your-files
).
Step 3: Create an object table in BigQuery
Create an Object table in BigQuery to point to your source image and video files in the Cloud Storage bucket. Object tables are read-only tables over unstructured data objects that reside in Cloud Storage. You can learn about other use cases for BigQuery object tables here.
Before you create the object table, establish a connection, as described here. Ensure that the connection's principal has the ‘Vertex AI User’ role and that the Vertex AI API is enabled for your project.
Create remote connection
- code_block
- <ListValue: [StructValue([('code', "CREATE OR REPLACE MODEL `dataset_name.model_name`\r\n REMOTE WITH CONNECTION `us.connection_name`\r\n OPTIONS (ENDPOINT = 'multimodalembedding@001');"), ('language', ''), ('caption', <wagtail.rich_text.RichText object at 0x3eea46f2f9d0>)])]>
Create object table
- code_block
- <ListValue: [StructValue([('code', "CREATE OR REPLACE EXTERNAL TABLE `dataset_name.table_name`\r\nWITH CONNECTION `us.connection_name`\r\nOPTIONS\r\n ( object_metadata = 'SIMPLE',\r\n uris = ['gs://bucket_name/*']\r\n );"), ('language', ''), ('caption', <wagtail.rich_text.RichText object at 0x3eea46f2fca0>)])]>
Step 4: Create your multimodal embeddings
We generate embeddings (numerical representations) for your media data using a pre-trained multimodal embedding model. These embeddings capture the semantic information of the content, enabling efficient similarity searches.
- code_block
- <ListValue: [StructValue([('code', "CREATE OR REPLACE TABLE `dataset_name.table_name`\r\nAS\r\nSELECT *\r\nFROM\r\n ML.GENERATE_EMBEDDING(\r\n MODEL `dataset_name.model_name`,\r\n (SELECT * FROM `dataset_name.table_name`))\r\nCheck for any errors during the embedding generation process. Errors may arise due to file format issues or problems with the source file.\r\nSELECT DISTINCT(ml_generate_embedding_status),\r\n COUNT(uri) AS num_rows\r\nFROM `dataset_name.table_name`\r\nGROUP BY 1;\r\nIf an error occurs, we can remove the problematic entry from the embedding table.\r\nDELETE FROM `dataset_name.table_name`\r\nWHERE ml_generate_embedding_status like '%INVALID_ARGUMENT:%';"), ('language', ''), ('caption', <wagtail.rich_text.RichText object at 0x3eea46f2f280>)])]>
Step 5: Create a vector index in BigQuery
Create a VECTOR INDEX in BigQuery for the embeddings to efficiently store and query the embeddings generated from your image and video data. This index is essential for performing similarity searches later.
- code_block
- <ListValue: [StructValue([('code', "CREATE OR REPLACE\r\n VECTOR INDEX `index_name`\r\nON\r\n dataset_name.table_name(ml_generate_embedding_result)\r\n OPTIONS (\r\n index_type = 'IVF', distance_type = 'COSINE');"), ('language', ''), ('caption', <wagtail.rich_text.RichText object at 0x3eea46f2f4c0>)])]>
Step 6: Send the user’s query as text input
A user’s query is sent as text input in simple natural language like “elephant eating grass”. When a user submits a query, the system converts this textual input into an embedding, similar to how it processed the media data.
Step 7: Create a text embedding for the user query
You can create a text embedding for the user query using the same multimodal embedding model. To compare the user query with the stored embeddings, first generate an embedding for the query itself using the same multimodal embedding model.
- code_block
- <ListValue: [StructValue([('code', 'CREATE OR REPLACE TABLE `dataset_name.table_name`\r\nAS\r\nSELECT * FROM ML.GENERATE_EMBEDDING(\r\n MODEL `dataset_name.model_name`,\r\n (\r\n SELECT ‘elephant eating grass’ AS content\r\n )\r\n);'), ('language', ''), ('caption', <wagtail.rich_text.RichText object at 0x3eea46f2ff40>)])]>
Step 8: Perform similarity search
Similarity search is performed between the user query and the source data containing images and videos using VECTOR SEARCH. Using the vector index created in Step 4, perform a similarity search to find the most similar media items to the user query. This search compares the user query's embedding with the embeddings of the media data.
- code_block
- <ListValue: [StructValue([('code', "SELECT base.uri AS gcs_uri, distance\r\n FROM\r\n VECTOR_SEARCH(\r\n TABLE `XXXXX.XXXXXX`,\r\n 'ml_generate_embedding_result',\r\n TABLE `XXXXX.XXXXXX`,\r\n 'ml_generate_embedding_result',\r\n top_k => 5,\r\n distance_type => 'COSINE',\r\n ORDER BY distance;"), ('language', ''), ('caption', <wagtail.rich_text.RichText object at 0x3eea46f2ff70>)])]>
Step 9: Return the search results for images and videos to the user
Finally, the results from the similarity search are presented to the user. The results include the URIs of the most similar images and videos stored in the Cloud Storage bucket, along with their similarity scores (distances). This allows the user to view or download the media items related to their query.
Multimodal embeddings powers a new level of search
Because multimodal embeddings can handle both image and video modalities, building a powerful search experience across your visual content is just a few steps away. No matter if your use case is image search, video search, or image and video search combined, get ready to unlock a new level of search enhancing your users’ experiences and streamlining content discovery.