In recent years, vision-language models (VLMs) have advanced significantly in bridging image, video, and textual modalities. Yet, a persistent limitation remains: the inability to effectively process long-context multimodal data such as high-resolution imagery or extended video sequences. Many existing VLMs are optimized for short-context scenarios and struggle with performance degradation, inefficient memory usage, or loss of semantic detail when scaled to handle longer inputs. Addressing these limitations requires not only architectural flexibility but also dedicated strategies for data sampling, training, and evaluation.
Eagle 2.5: A Generalist Framework for Long-Context Learning
NVIDIA introduces Eagle 2.5, a family of vision-language models designed for long-context multimodal learning. Unlike models that simply accommodate more input tokens, Eagle 2.5 demonstrates measurable and consistent performance improvements as input length increases. The system is developed with a focus on both video and image understanding at scale, targeting tasks where the richness of long-form content is critical.
Eagle 2.5 operates with a relatively compact 8B parameter count and yet achieves strong results across established benchmarks. On Video-MME (with 512-frame input), the model scores 72.4%, approaching or matching results from significantly larger models such as Qwen2.5-VL-72B and InternVL2.5-78B. Notably, these gains are achieved without relying on task-specific compression modules, reflecting the model’s generalist design philosophy.
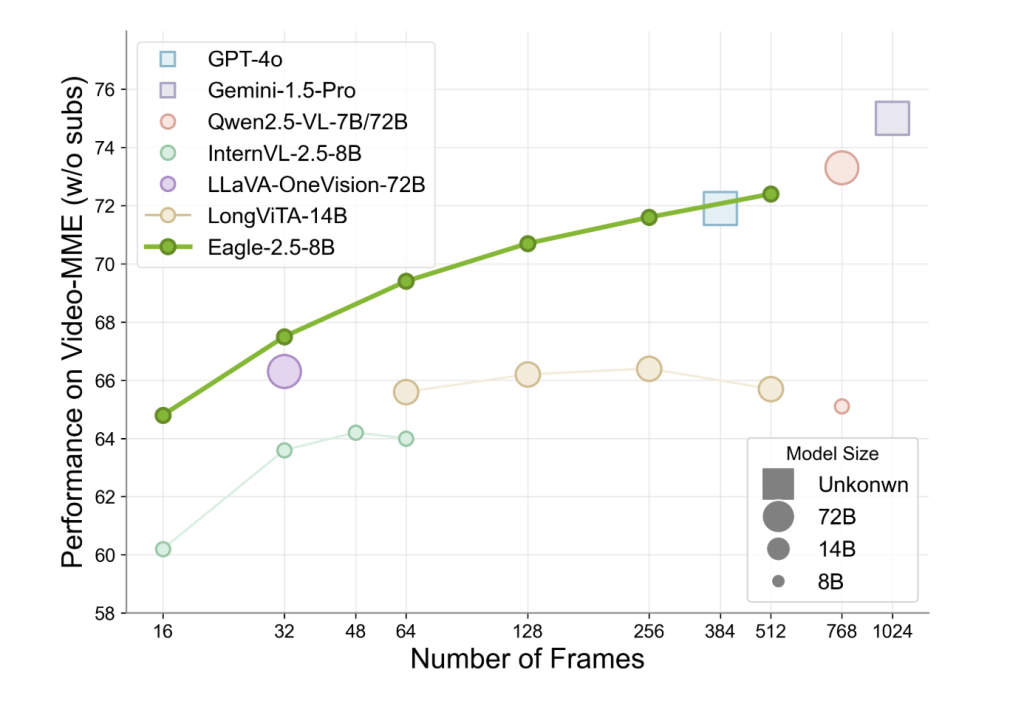
Training Strategy: Context-Aware Optimization
The effectiveness of Eagle 2.5 stems from two complementary training strategies: information-first sampling and progressive post-training.
- Information-First Sampling prioritizes retention of critical visual and semantic content. It introduces Image Area Preservation (IAP), a tiling scheme that maintains over 60% of the original image area while minimizing aspect ratio distortion. Additionally, Automatic Degradation Sampling (ADS) dynamically balances visual and textual inputs based on context length constraints, preserving full textual sequences and adaptively optimizing visual granularity.Progressive Post-Training incrementally increases the model’s context window—moving through 32K, 64K, and 128K token stages. This gradual exposure allows the model to develop consistent capabilities across input lengths. The method avoids overfitting to any single context range and helps maintain stable performance in diverse inference scenarios.
These approaches are underpinned by an architecture based on SigLIP for vision encoding and MLP projection layers for alignment with the language model backbone. The system forgoes domain-specific compression components to retain flexibility across varied task types.

Eagle-Video-110K: Structured Data for Extended Video Comprehension
A key component of Eagle 2.5 is its training data pipeline, which integrates both open-source resources and a custom-curated dataset: Eagle-Video-110K. This dataset is constructed to support long-form video understanding and adopts a dual annotation scheme:
- A top-down approach introduces story-level segmentation using human-annotated chapter metadata and GPT-4-generated dense captions and question-answer pairs.A bottom-up method generates QA pairs for short clips using GPT-4o, augmented with time and textual context anchors to capture spatiotemporal detail.
The dataset collection emphasizes diversity over redundancy. A cosine similarity-based selection process filters novel content from sources such as InternVid, Shot2Story, and VidChapters. This results in a corpus with both narrative coherence and granular annotations, enabling models to capture hierarchical information across time.

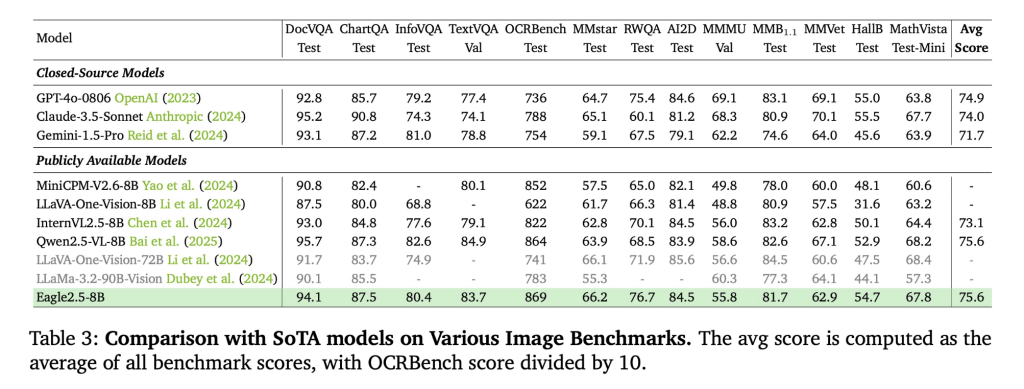
Performance and Benchmarking
Eagle 2.5-8B exhibits robust performance across multiple video and image understanding tasks. On video benchmarks, it scores 74.8 on MVBench, 77.6 on MLVU, and 66.4 on LongVideoBench. On image benchmarks, the model attains 94.1 on DocVQA, 87.5 on ChartQA, and 80.4 on InfoVQA, among others.
Ablation studies confirm the importance of Eagle’s sampling strategies. Removal of IAP leads to performance degradation in high-resolution benchmarks, while omitting ADS reduces effectiveness in tasks requiring dense supervision. The model also benefits from progressive training: sequentially increasing context lengths provides more stable gains compared to one-shot long-context training. Importantly, the addition of Eagle-Video-110K notably enhances performance at higher frame counts (≥128 frames), underscoring the value of dedicated long-form datasets.
Conclusion
Eagle 2.5 presents a technically grounded approach to long-context vision-language modeling. Its emphasis on preserving contextual integrity, gradual training adaptation, and dataset diversity enables it to achieve strong performance while maintaining architectural generality. Without relying on model scaling alone, Eagle 2.5 demonstrates that careful training strategies and data design can yield competitive, efficient systems for complex multimodal understanding tasks. This positions Eagle 2.5 as a valuable step forward in building more context-aware AI systems suited for real-world multimedia applications.
Check out the Paper, GitHub Page and Project Page. Also, don’t forget to follow us on Twitter and join our Telegram Channel and LinkedIn Group. Don’t Forget to join our 90k+ ML SubReddit.
The post Long-Context Multimodal Understanding No Longer Requires Massive Models: NVIDIA AI Introduces Eagle 2.5, a Generalist Vision-Language Model that Matches GPT-4o on Video Tasks Using Just 8B Parameters appeared first on MarkTechPost.