Financial institutions today face an increasingly complex regulatory world that demands robust, efficient compliance mechanisms. Although organizations traditionally invest countless hours reviewing regulations such as the Anti-Money Laundering (AML) rules and the Bank Secrecy Act (BSA), modern AI solutions offer a transformative approach to this challenge. By using Amazon Bedrock Knowledge Bases alongside CrewAI—an open source multi-agent orchestration framework, organizations can now deploy intelligent systems where multiple AI agents work together to automate and streamline specific compliance processes. This powerful combination enables financial institutions to move from manual, time-intensive compliance reviews to a streamlined, assisted compliance management approach that adapts to evolving regulatory requirements.
In this post, we explore how AI agents can streamline compliance and fulfill regulatory requirements for financial institutions using Amazon Bedrock and CrewAI. We demonstrate how to build a multi-agent system that can automatically summarize new regulations, assess their impact on operations, and provide prescriptive technical guidance. You’ll learn how to use Amazon Bedrock Knowledge Bases and Amazon Bedrock Agents with CrewAI to create a comprehensive, automated compliance solution.
This solution’s architecture can be adapted to help healthcare systems, enable manufacturers to maintain ISO safety documentation, and assist retailers in monitoring Federal Trade Commission (FTC) advertising regulations. It can also assist in other segments such as legal, finance, or human resources, offering wide-ranging potential for process automation and efficiency gains across various industries.The code used for this post is available on GitHub.
Solution overview
Traditional large language model (LLM) applications excel at following predefined instructions, but solving complex challenges such as compliance automation requires an autonomous network of specialized agents that mirror the structure of a comprehensive compliance department. Our system employs three key agents:
- Compliance analyst agent that continuously monitors and analyzes regulatory changes Compliance specialist agent that transforms requirements into organizational policies Enterprise architect agent that designs and implements the necessary security controls
In this multi-agent approach, specialized AI agents work together seamlessly to streamline the compliance lifecycle. The compliance analyst agent collects latest regulatory changes and helps to stay ahead of regulatory changes and their potential impact where the Compliance specialist agent translates these regulatory requirements into actionable organizational procedures. Meanwhile, the enterprise architect agent makes sure that the technical controls align with organizational controls. CrewAI provides an open source framework to orchestrate this collaborative system, enabling these agents to work in concert while maintaining clear handoffs and accountability. Next, we will explore how to create this multi-agent compliance automation system using CrewAI.
Although this solution demonstrates CrewAI’s capabilities, it’s important to note that Amazon Bedrock Agents has built-in support for multi-agent collaboration, and organizations could implement their agent workflows entirely within Amazon Bedrock Agents. However, we’ve chosen CrewAI for this demonstration to showcase how open source frameworks can extend Amazon Bedrock capabilities while maintaining enterprise-grade security through Bedrock Guardrails.
Solution components
This solution shows you how to combine multiple capabilities. It shows how to:
- Develop a multi-agent solution using a CrewAI framework Enrich the solution using domain-specific data using Amazon Bedrock Knowledge Bases Safeguard your generative AI application using Amazon Bedrock Guardrails Bring everything together using CrewAI and Amazon Bedrock Agents
You can use CrewAI to develop AI agents and coordinate tasks among those agents. This structure enables systematic management of complex AI workflows while maintaining oversight of agent interactions and outcomes. The framework has the following components, which are shown in the following figure:
CrewAI Framework is built around the following components:
- Agents in CrewAI are autonomous components designed to perform specific tasks or roles within a multi-agent system. They have specific roles (such as researcher or writer) and make autonomous decisions with or without using external tools. LLMs are the core intelligence behind CrewAI agents. LLMs enable agents to understand context, make decisions, and generate human-like responses. Tasks are defined jobs assigned to agents with clear objectives, including execution details and required resources. Crews are coordinated teams of agents working together on a shared goal. Crews require defining agent roles, task assignments, and execution order. Tools refer to the skills or functions that agents can use to carry out various actions. Processes are responsible for orchestrating how tasks are executed by agents, similar to project management in human teams. These processes make sure that tasks are allocated and completed efficiently, in accordance with a predefined strategy.
Prerequisites
Before getting started with the solution, you need to get access to Amazon Bedrock models:
- Sign in to the Amazon Bedrock console and in the navigation pane under Bedrock configurations, select Model access to request access to Amazon Bedrock models. This step is shown in the following screenshots.
In this example, we use Amazon Nova Pro through Amazon Bedrock as our LLM. CrewAI provides built-in integration with Amazon Bedrock.
- Clone the GitHub repo into a local folder
3. Use the following command to install the dependencies for running CrewAI in your Python environment:
Your compliance agents
In this step, you will define your agents:
- Define compliance agents in the
agents.yaml
file. Each agent has a specific role to play: Define tasks for the agents: The execution and process steps are defined in crew.py
: Define your LLM, topic, and runtime parameters in the .env
file: Run the crew as follows: The following demo shows the output of the crew. You can see the agents collaborating to generate a detailed solution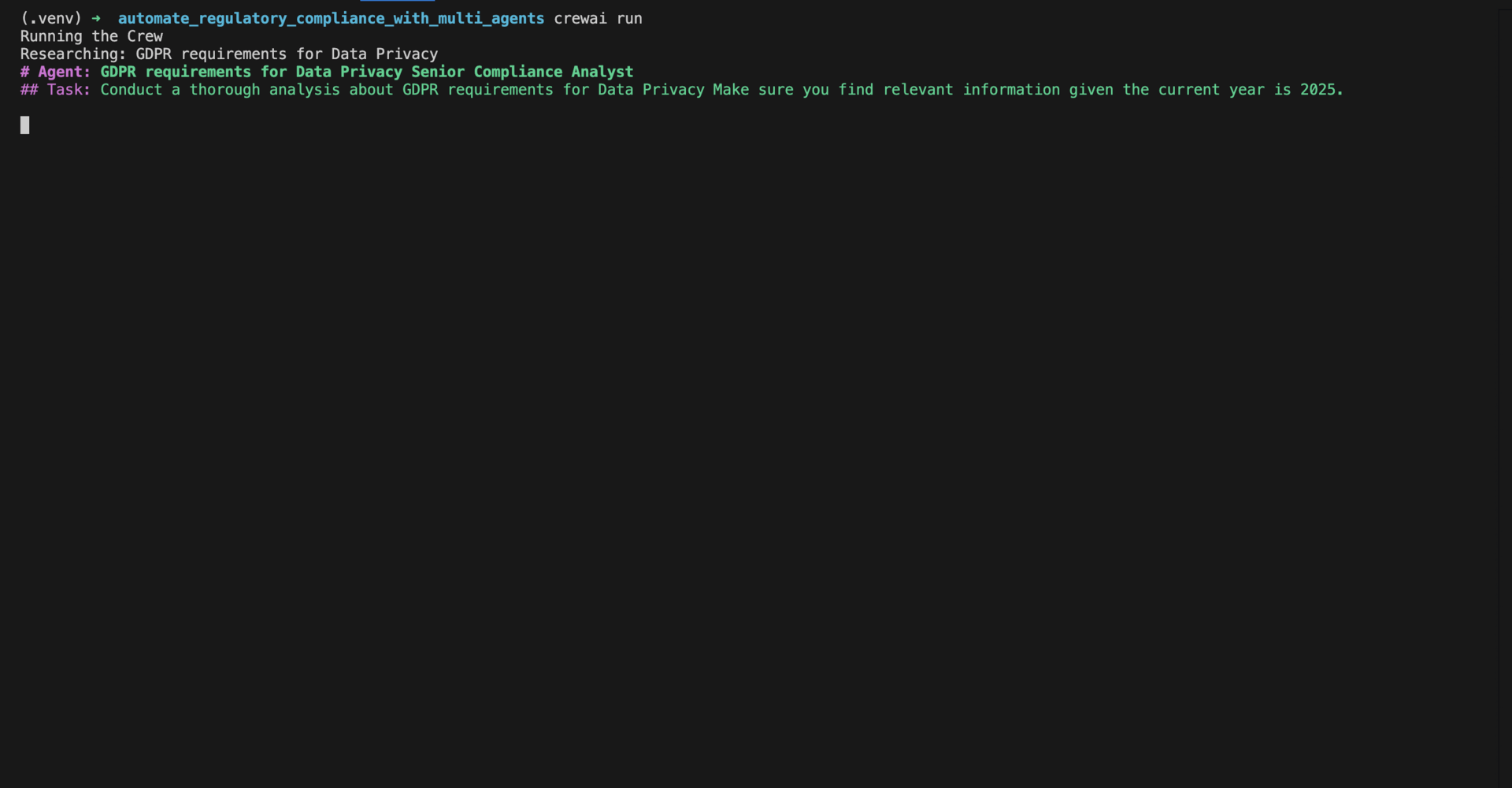
In the output, notice that the compliance analyst and the compliance specialist are working together to solve multiple aspects of General Data Protection Regulation (GDPR) requirements for trading services. Note the synergistic collaboration between agents as they refine their approach and develop a comprehensive compliance management response through iterative problem-solving.
Addressing LLM challenges with domain-specific knowledge
LLMs, although impressive in their broad knowledge, face two key limitations when dealing with specialized domains or recent information. First, they struggle with specialized information specific to your organization. Second, because their knowledge is limited to their training data, they might not reflect the latest updates in rapidly changing fields. This limitation becomes particularly important when dealing with evolving compliance requirements, such as Payment Card Industry Data Security Standard (PCI DSS), GDPR, AML rules, and Know Your Customer (KYC) regulations. Additionally, organizations need solutions that are customized to their specific compliance requirements and internal standards, rather than generic responses from LLMs.
Retrieval Augmented Generation (RAG) is a technique that enables generative AI models to retrieve and incorporate current organizational and domain-specific information from external databases. Amazon Bedrock Knowledge Base is a managed capability that helps you implement the entire RAG technique without having to build custom integrations to data sources and manage data flows. By incorporating a knowledge base containing the latest publications, regulatory updates, and compliance guidelines from authoritative sources such as NIST, ISO, PCI, and regulatory bodies, Amazon Bedrock Knowledge Bases helps make sure that your AI system stays current with the latest compliance requirements. During prompt generation, RAG first retrieves relevant data from this continually updated knowledge base, then uses it to create informed responses. This helps provide more relevant, accurate, and customized responses aligned with current regulatory and organizational standards. For example, when querying about PCI DSS v4.0 requirements or recent GDPR amendments, the system can pull the most up-to-date information directly from authoritative sources rather than relying on potentially outdated training data.
Create an Amazon Bedrock knowledge base with contextual information from your data sources
- From the Amazon Bedrock navigation pane, select Knowledge Bases under Builder tools and choose a Knowledge Base with vector store.
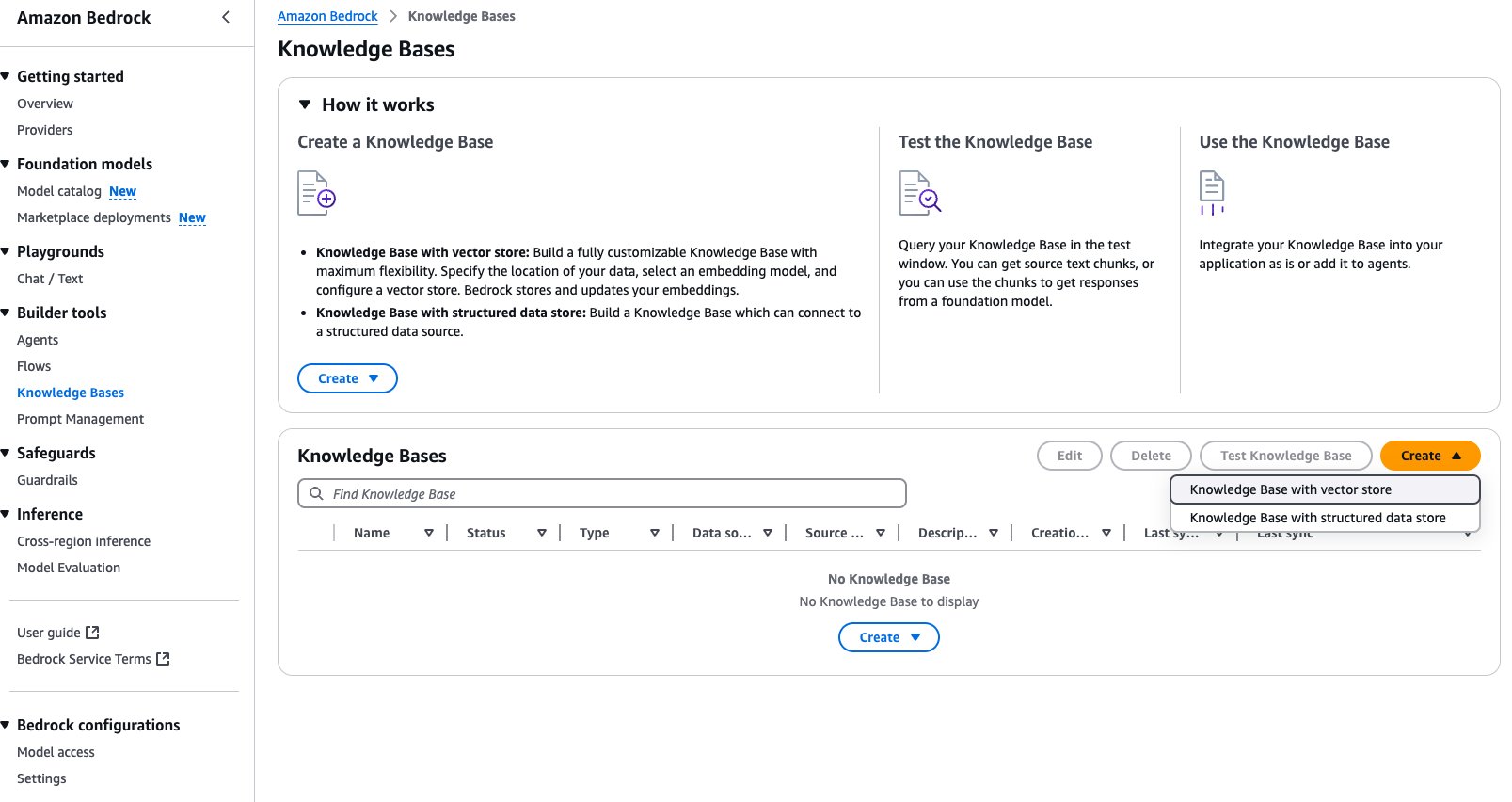
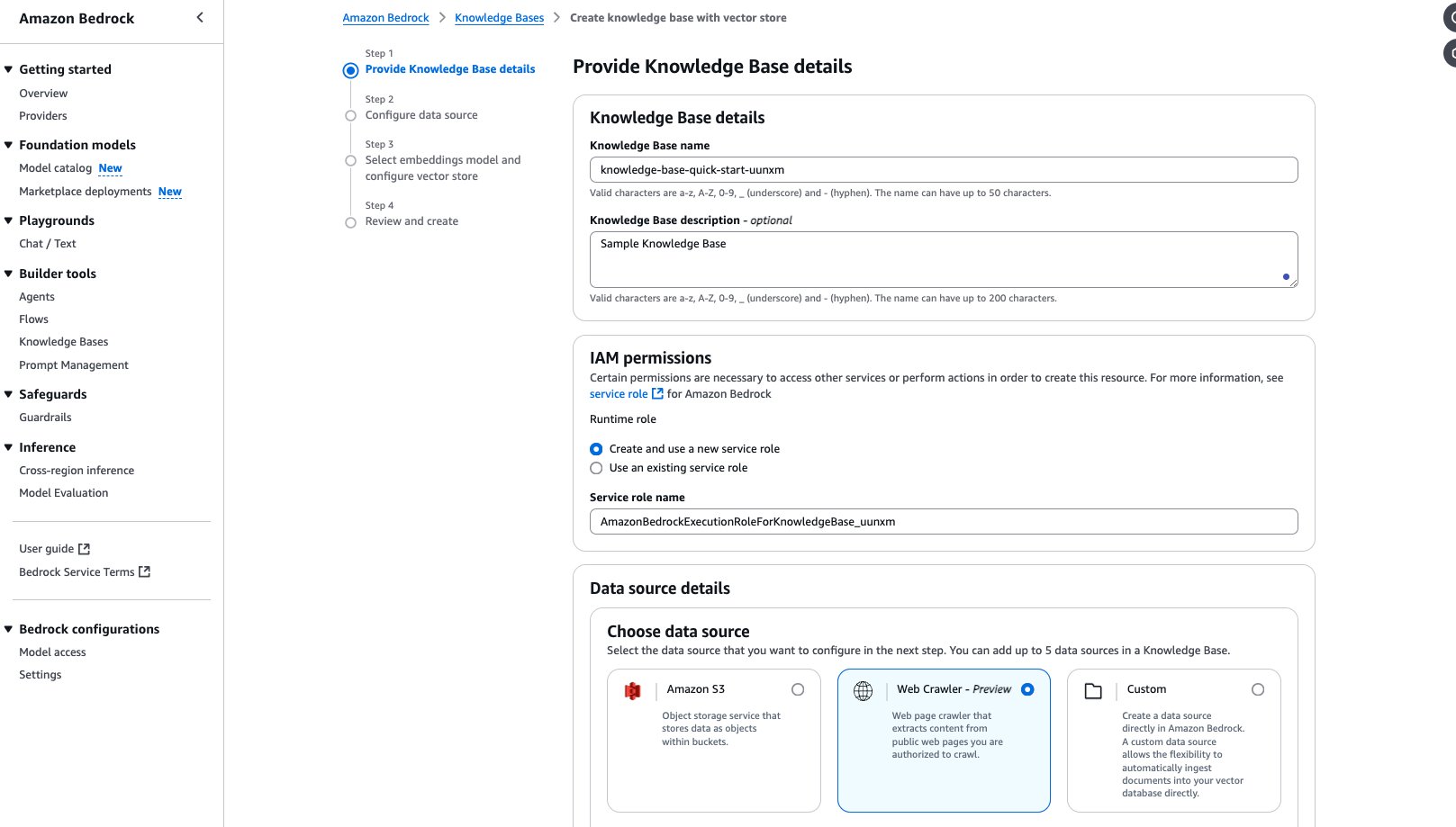
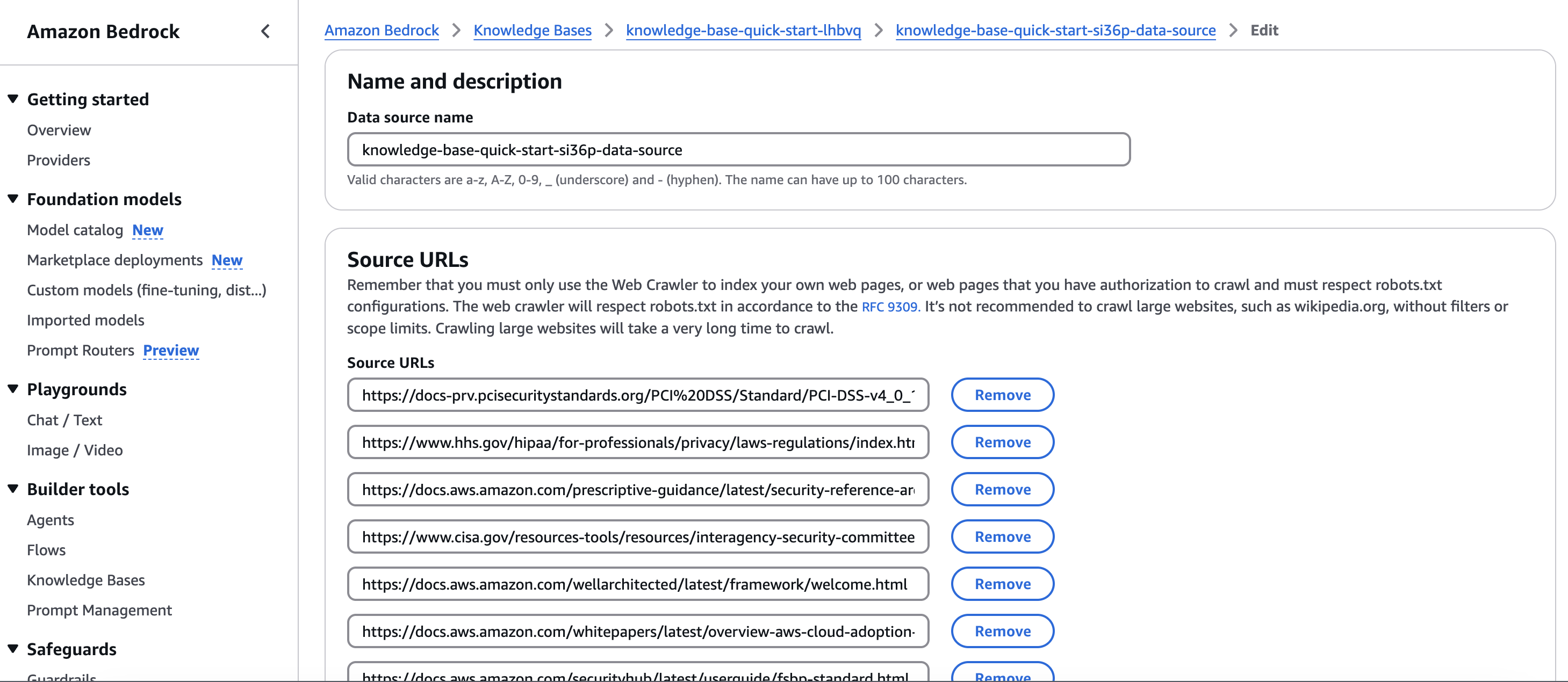
- Select the model for embeddings. We have selected Amazon Titan Text Embeddings v2, as shown in the following screenshot.
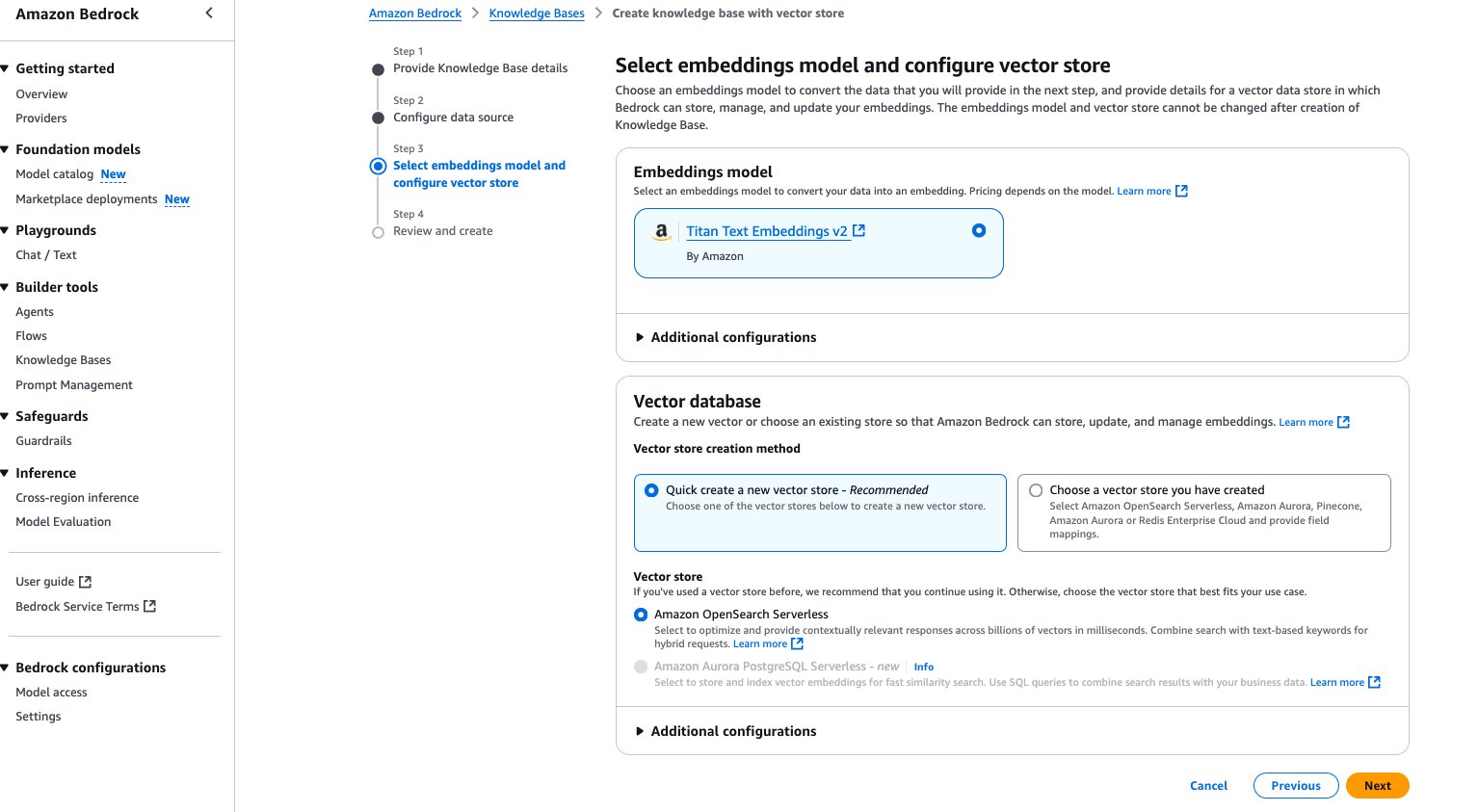
After a few minutes, the knowledge base will be ready. After it has synced, Amazon Bedrock Knowledge Bases handles generating, running, and formatting the result of the query, simplifying building natural language interfaces to structured data.
Amazon Bedrock Agents
Amazon Bedrock Agents is a comprehensive environment for building sophisticated AI agent systems. At its core, it enables seamless multi-agent collaboration and maintains conversation context through native memory retention across interactions. Amazon Bedrock Agents integrates naturally with knowledge bases and enforce security through built-in guardrails. For this solution, we focus on two key capabilities: the RAG feature, which allows agents to access and utilize information from knowledge bases, and the security features provided through Amazon Bedrock Guardrails. These guardrails serve as an essential safeguard for your generative AI applications, promoting responsible and secure AI interactions.
- To create an agent, from the Amazon Bedrock navigation pane under Builder tools, select Agents and select Create Agent.
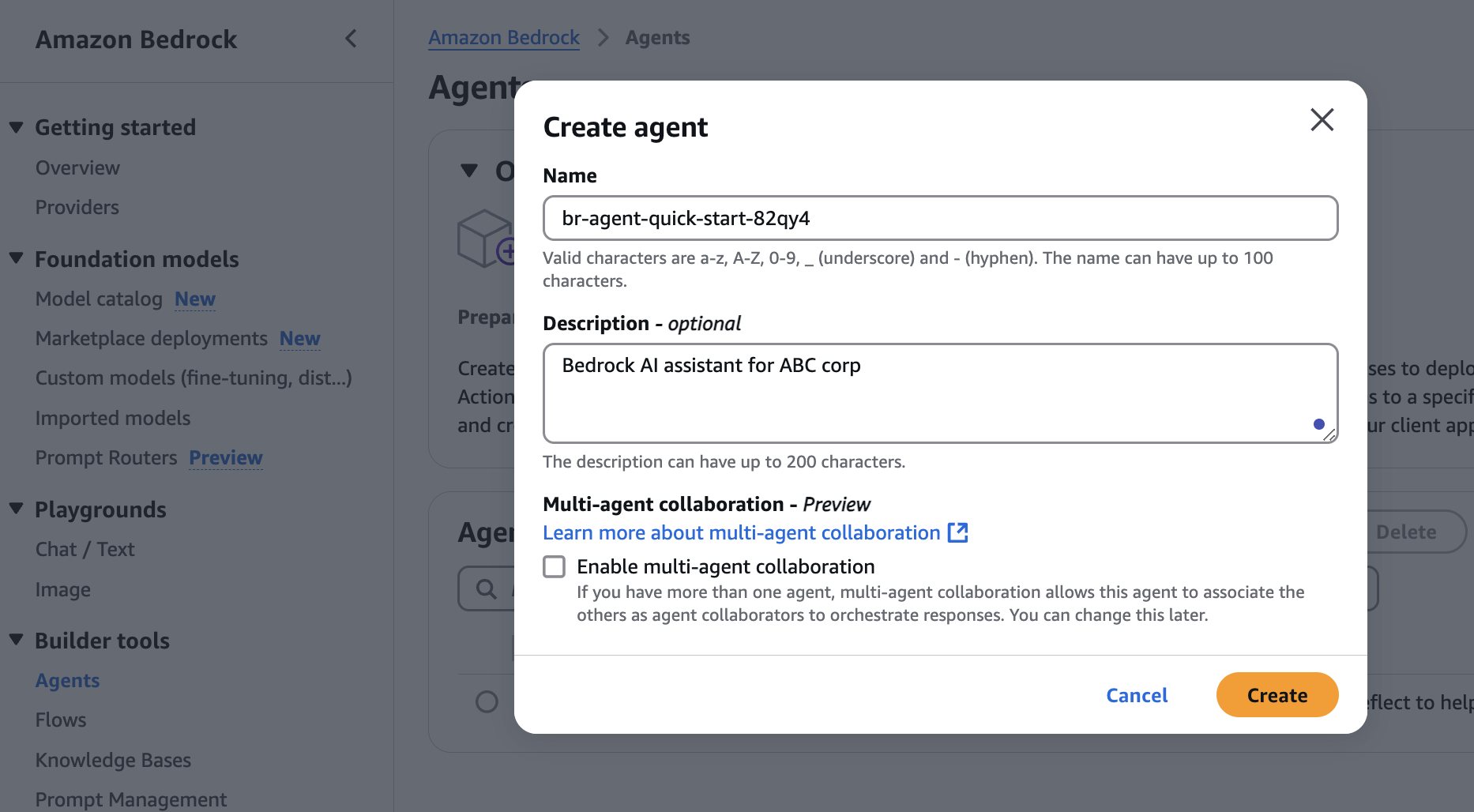
- Under Agent details, choose the model. We use Amazon Nova Pro for our use case, as shown in the following screenshot.
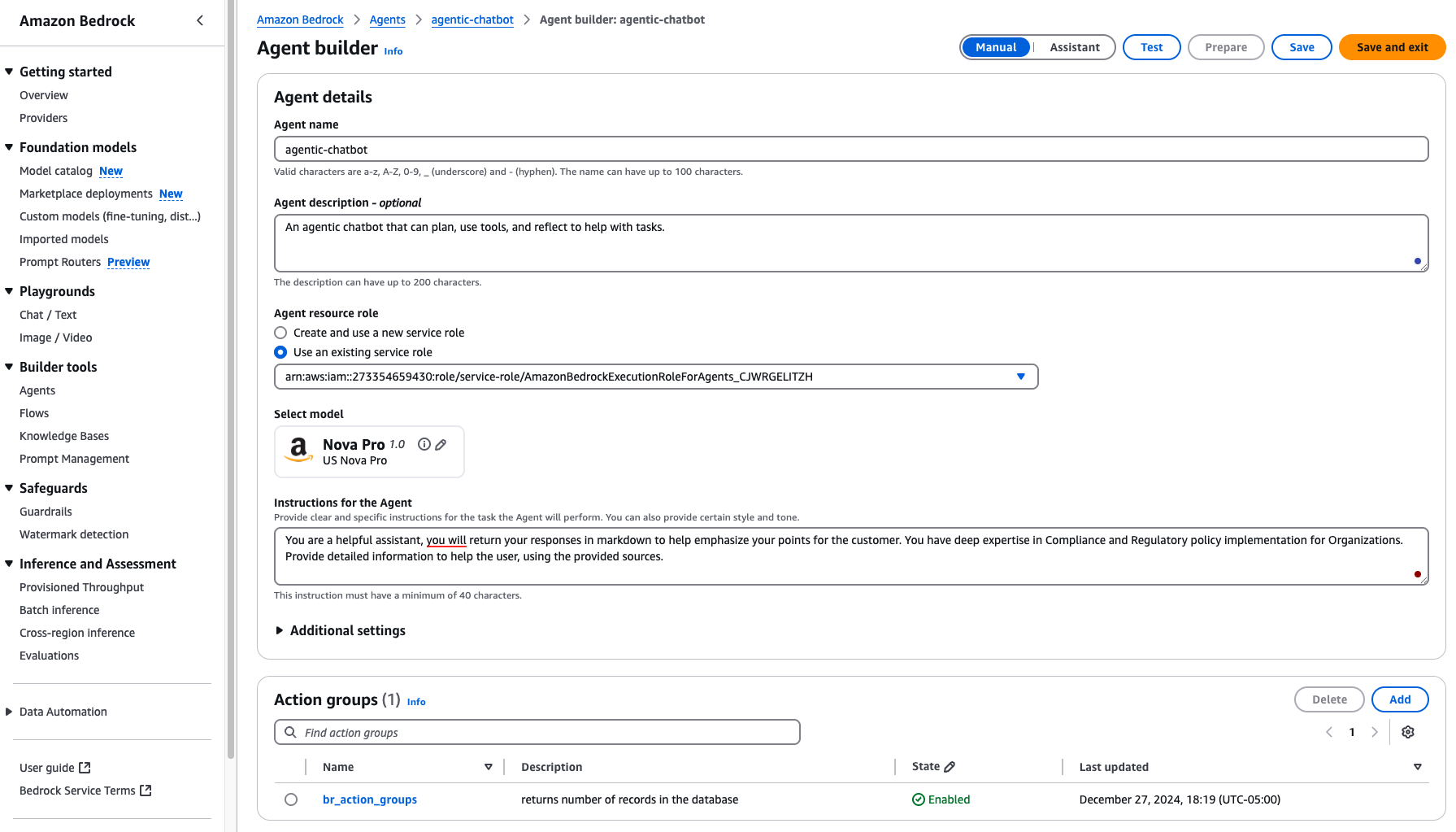
- Under Knowledge Bases, add knowledge bases to your agent.
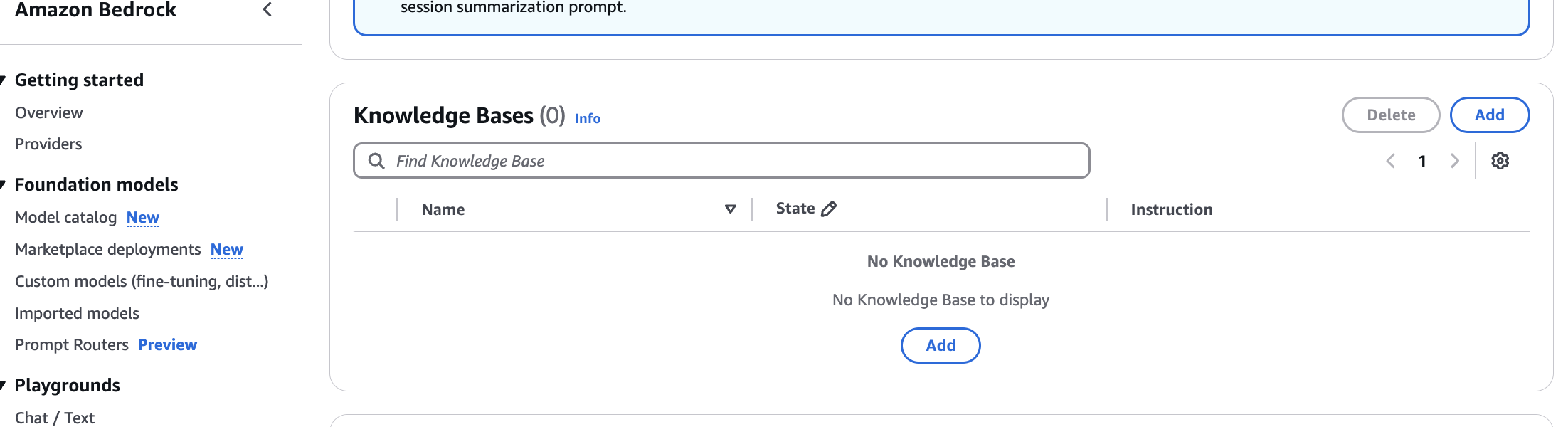
- Choose the knowledge base name from the dropdown list, as shown in the following screenshot.
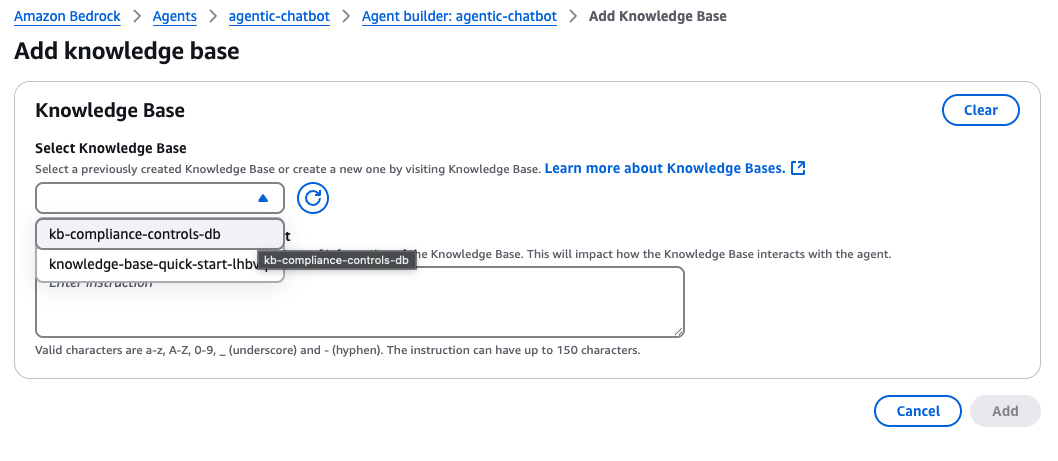
Amazon Bedrock Guardrails
Amazon Bedrock Guardrails provides safety controls that help maintain responsible AI use by providing a layer of security. Guardrails provide content filtering to monitor and filter AI model outputs to help prevent harmful, inappropriate, or biased content. You can set up filters for things such as hate speech, explicit content, or personally identifiable information (PII). You can also apply customizable rules and input/output validation.
- You can find Guardrails in the Amazon Bedrock navigation pane under Safeguards. Choose Create guardrail and provide a guardrail name
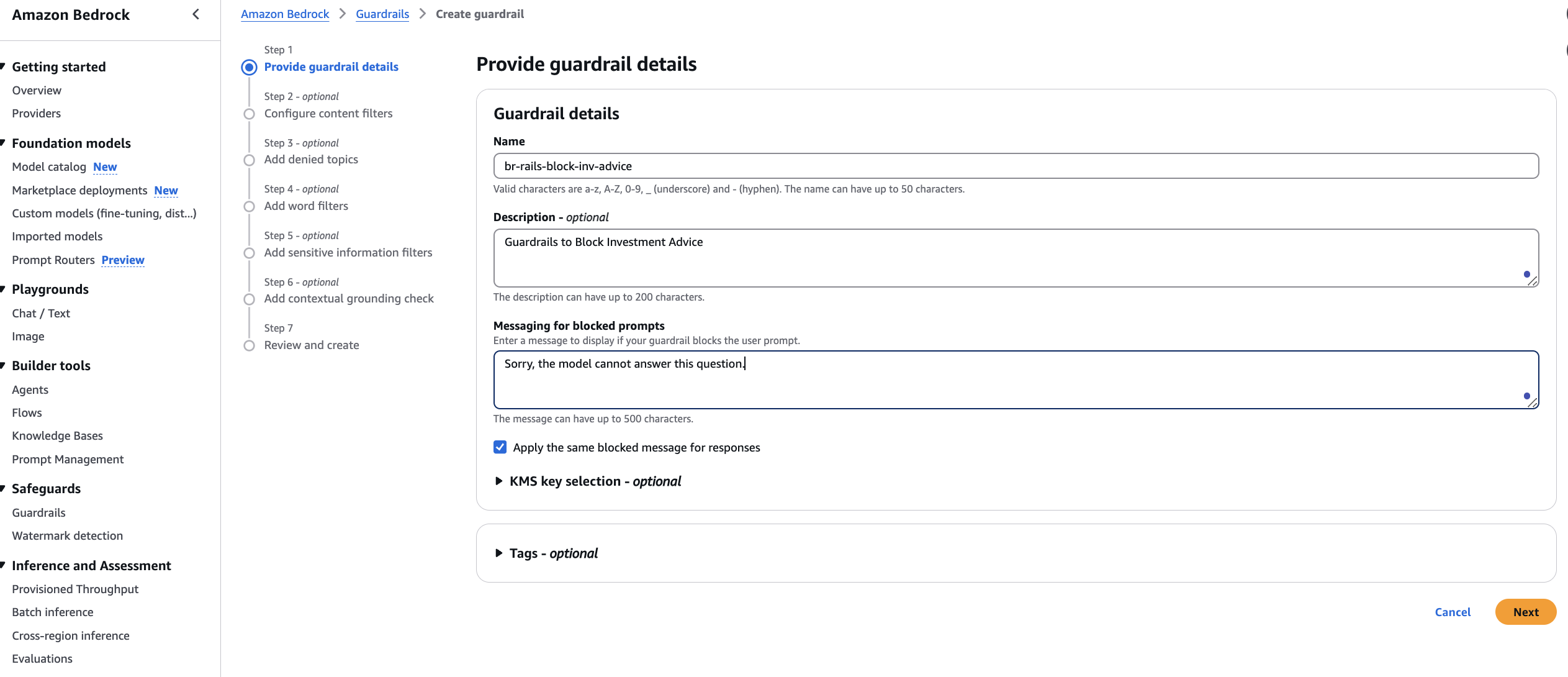
- As shown in the following screenshot, select the content filters you want to implement for your Amazon Bedrock based application
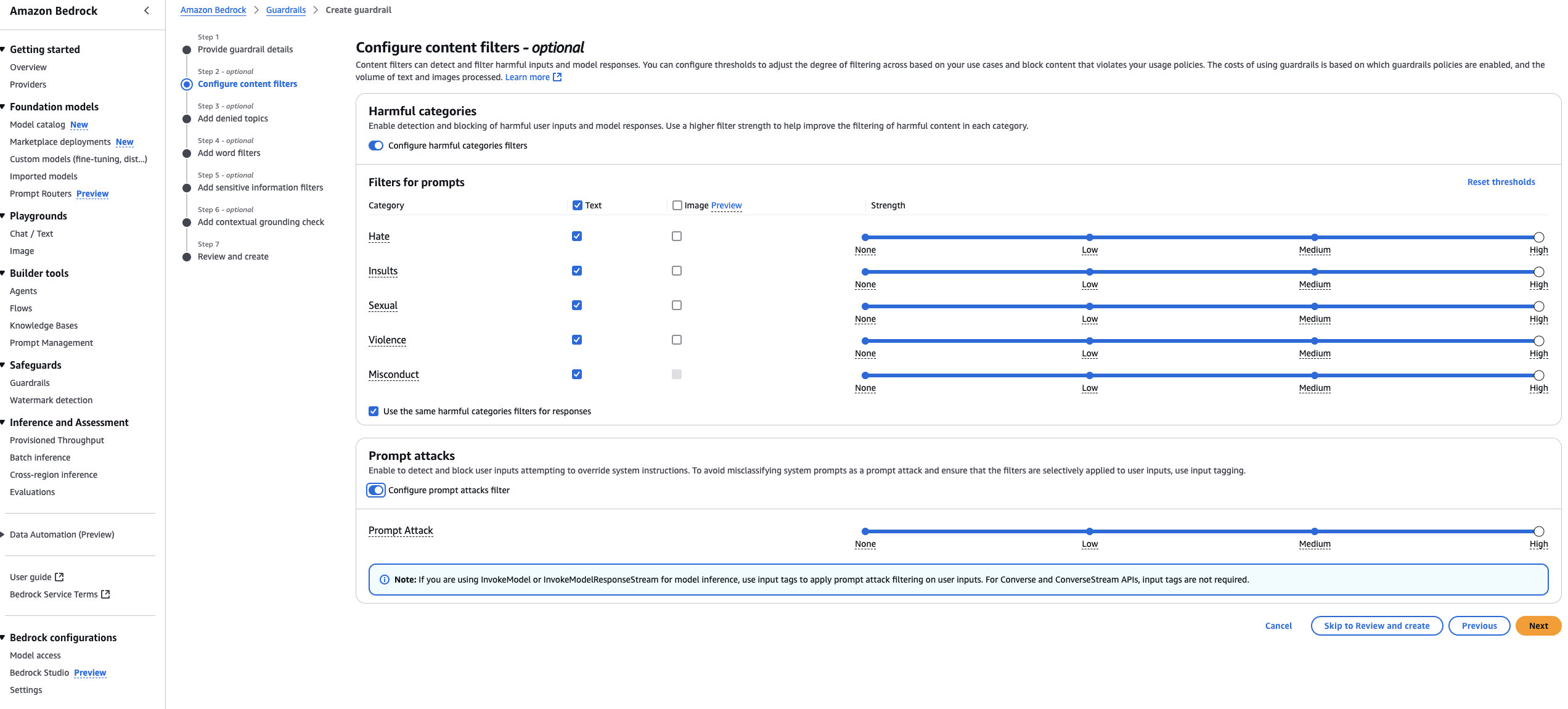
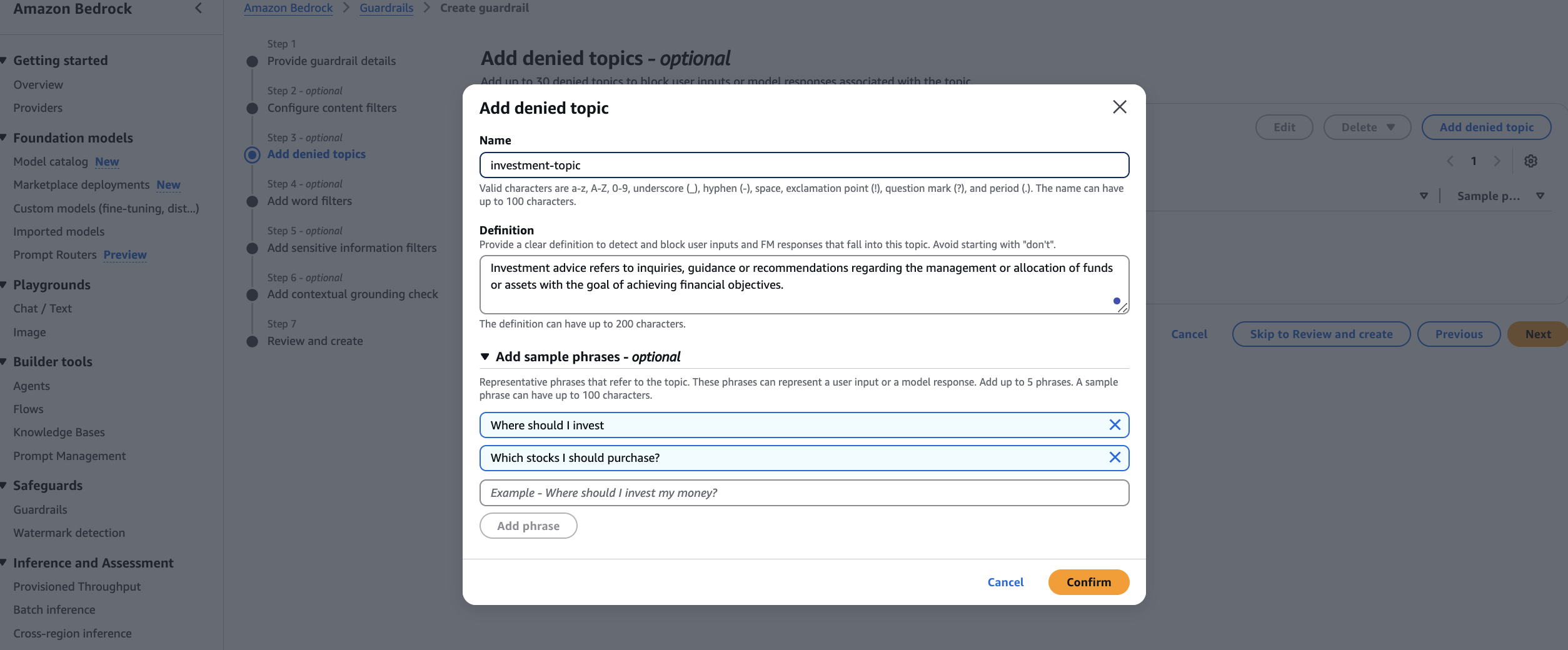
Putting it all together: Integrating Amazon Bedrock Agents with CrewAI
CrewAI provides seamless integration with Amazon Bedrock features, including Amazon Bedrock Knowledge Bases and Amazon Bedrock Agents through CrewAI tools functionality. When these tools are triggered from CrewAI agents, they process your query, retrieve the relevant information from the Amazon Bedrock knowledge base, and return responses back to CrewAI agent.
- Refer to the sample code demonstrating CrewAI tools for Amazon Bedrock Agent. You need to define your Amazon Bedrock AgentId and Alias as parameters in the .env file Execute the crew again with Amazon Bedrock Agents: You can find the generated output below
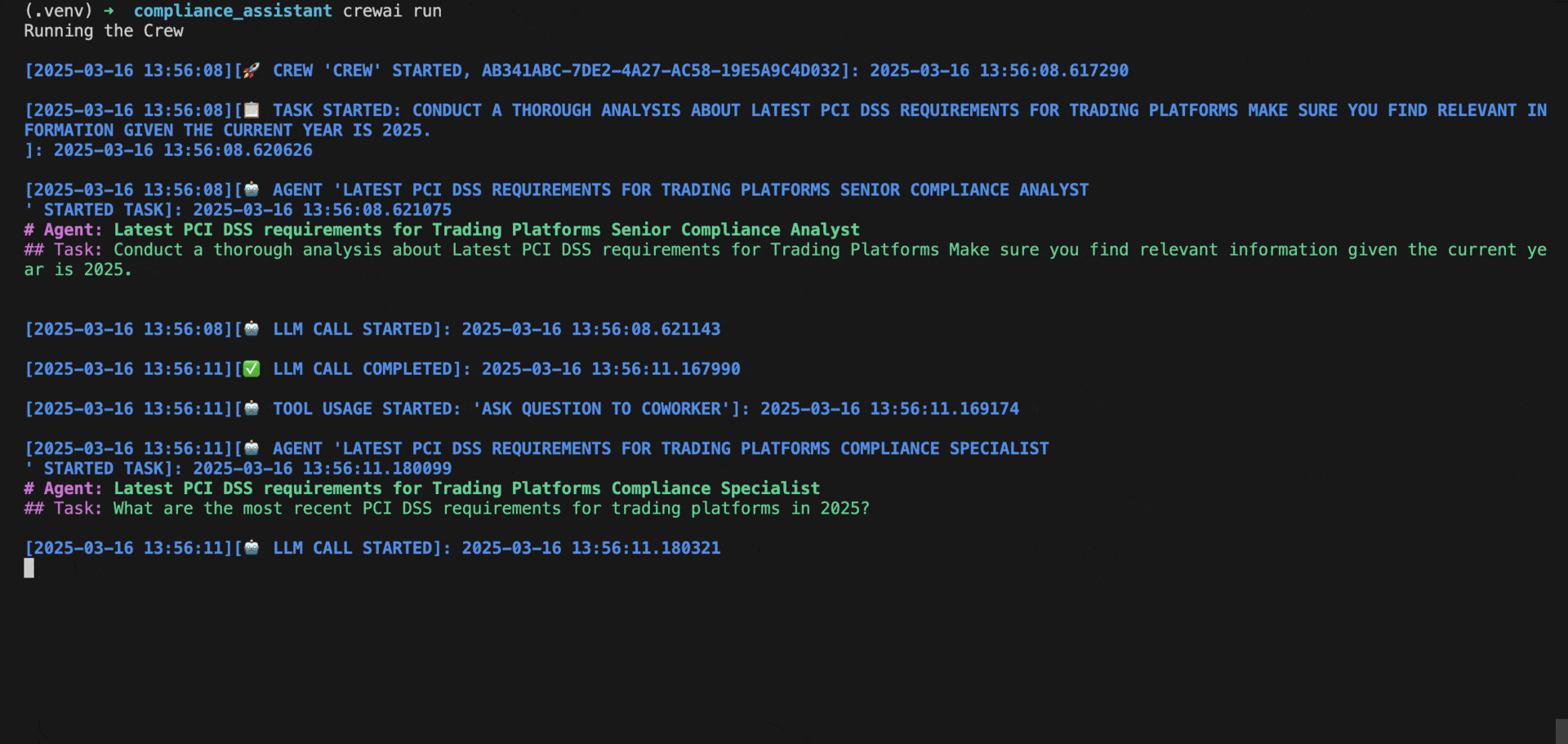
When you execute the crew, the compliance analyst agent initiates the process by invoking the CrewAI Bedrock tool to extract regulatory requirements from Amazon Bedrock Knowledge Bases, which is then seamlessly transformed into technical requirements by the compliance specialist agent. Through iterative collaboration, these specialized agents work together to fill information gaps, and the enterprise architect agent synthesizes the gathered insights to develop a robust implementation strategy and execution plan. This streamlined process demonstrates how multiple AI agents can effectively coordinate to transform compliance requirements into actionable technical solutions.
Clean up
To avoid ongoing charges, follow these steps to clean up resources:
- Delete the Amazon Bedrock knowledge base that you created:
- Delete the Amazon Bedrock agents that you created:
Conclusion
In this post, we demonstrated how to:
- Build a multi-agent AI system using CrewAI that mimics the structure of a comprehensive compliance department with specialized agents for different functions Enhance AI responses with domain-specific knowledge by implementing RAG using Amazon Bedrock Knowledge Bases Safeguard your generative AI applications with Amazon Bedrock Guardrails to help prevent harmful, inappropriate, or biased content Create custom tools in CrewAI to integrate with Amazon Bedrock Agents for more powerful and context-aware compliance solutions Automate the entire compliance lifecycle from monitoring regulatory changes to implementing technical controls without extensive manual effort Deploy a production-ready solution that continually adapts to evolving regulatory requirements in financial services and other highly regulated industries
This solution combines Amazon Bedrock Knowledge Bases and CrewAI to create smart, multi-agent AI systems that help streamline regulatory compliance tasks. With simplified RAG implementation, sophisticated workflows that mirror human teams, and faster adaptation to new regulations, this approach shows how AI can assist organizations with specific aspects of complex regulatory requirements.
This solution serves as a practical starting point for organizations looking to enhance their compliance processes with AI capabilities, demonstrating how intelligent systems could complement and streamline existing compliance workflows. The complete source code for this project is available on the GitHub repository. Feel free to explore, fork, or contribute!
About the Authors
Balu Mathew is a Senior Solutions Architect at AWS, based in Raleigh, NC. He collaborates with Global Financial Services customers to design and implement secure, scalable and resilient solutions on AWS. With deep expertise in security, machine learning, and the financial services industry, he helps organizations build, protect, and scale large-scale distributed systems efficiently. Outside of work, he enjoys spending time with his kids and exploring the mountains and the outdoors.