In the rapidly evolving healthcare landscape, patients often find themselves navigating a maze of complex medical information, seeking answers to their questions and concerns. However, accessing accurate and comprehensible information can be a daunting task, leading to confusion and frustration. This is where the integration of cutting-edge technologies, such as audio-to-text translation and large language models (LLMs), holds the potential to revolutionize the way patients receive, process, and act on vital medical information.
As the healthcare industry continues to embrace digital transformation, solutions that combine advanced technologies like audio-to-text translation and LLMs will become increasingly valuable in addressing key challenges, such as patient education, engagement, and empowerment. By taking advantage of these innovative technologies, healthcare providers can deliver more personalized, efficient, and effective care, ultimately improving patient outcomes and driving progress in the life sciences domain.
For instance, envision a voice-enabled virtual assistant that not only understands your spoken queries, but also transcribes them into text with remarkable accuracy. This transcription then serves as the input for a powerful LLM, which draws upon its vast knowledge base to provide personalized, context-aware responses tailored to your specific situation. This solution can transform the patient education experience, empowering individuals to make informed decisions about their healthcare journey.
In this post, we discuss possible use cases for combining speech recognition technology with LLMs, and how the solution can revolutionize clinical trials.
By combining speech recognition technology with LLMs, the solution can accurately transcribe a patient’s spoken queries into text, enabling the LLM to understand and analyze the context of the question. The LLM can then use its extensive knowledge base, which can be regularly updated with the latest medical research and clinical trial data, to provide relevant and trustworthy responses tailored to the patient’s specific situation.
Some of the potential benefits of this integrated approach are that patients can receive instant access to reliable information, empowering them to make more informed decisions about their healthcare. Additionally, the solution can help alleviate the burden on healthcare professionals by providing patients with a convenient and accessible source of information, freeing up valuable time for more critical tasks. Furthermore, the voice-enabled interface can enhance accessibility for patients with disabilities or those who prefer verbal communication, making sure that no one is left behind in the pursuit of better health outcomes.
Use cases overview
In this section, we discuss several possible use cases for this solution.
Use case 1: Audio-to-text translation and LLM integration for clinical trial patient interactions
In the domain of clinical trials, effective communication between patients and physicians is crucial for gathering accurate data, enforcing patient adherence, and maintaining study integrity. This use case demonstrates how audio-to-text translation combined with LLM capabilities can streamline and enhance the process of capturing and analyzing patient-physician interactions during clinical trial visits and telemedicine sessions.
Don’t feel like reading the full use case? No problem! You can listen to the key details in our audio file instead.
The process flow consists of the following steps:
- Audio capture – During patient visits or telemedicine sessions, the audio of the patient-physician interaction is recorded securely, with appropriate consent and privacy measures in place. Audio-to-text translation – The recorded audio is processed through an advanced speech recognition (ASR) system, which converts the audio into text transcripts. This step provides an accurate and efficient conversion of spoken words into a format suitable for further analysis. Text preprocessing – The transcribed text undergoes preprocessing steps, such as removing identifying information, formatting the data, and enforcing compliance with relevant data privacy regulations. LLM integration – The preprocessed text is fed into a powerful LLM tailored for the healthcare and life sciences (HCLS) domain. The LLM analyzes the text, identifying key information relevant to the clinical trial, such as patient symptoms, adverse events, medication adherence, and treatment responses. Intelligent insights and recommendations – Using its large knowledge base and advanced natural language processing (NLP) capabilities, the LLM provides intelligent insights and recommendations based on the analyzed patient-physician interaction. These insights can include:
- Potential adverse event detection and reporting. Identification of protocol deviations or non-compliance. Recommendations for personalized patient care or adjustments to treatment regimens. Extraction of relevant data points for electronic health records (EHRs) and clinical trial databases.
The solution offers the following potential benefits:
- Improved data accuracy – By accurately capturing and analyzing patient-physician interactions, this approach minimizes the risks of manual transcription errors and provides high-quality data for clinical trial analysis and decision-making. Enhanced patient safety – The LLM’s ability to detect potential adverse events and protocol deviations can help identify and mitigate risks, improving patient safety and study integrity. Personalized patient care – Using the LLM’s insights, physicians can provide personalized care recommendations, tailored treatment plans, and better manage patient adherence, leading to improved patient outcomes. Streamlined data collection and analysis – Automating the process of extracting relevant data points from patient-physician interactions can significantly reduce the time and effort required for manual data entry and analysis, enabling more efficient clinical trial management. Regulatory compliance – By integrating the extracted insights and recommendations into clinical trial management systems and EHRs, this approach facilitates compliance with regulatory requirements for data capture, adverse event reporting, and trial monitoring.
This use case demonstrates the potential of combining audio-to-text translation and LLM capabilities to enhance patient-physician communication, improve data quality, and support informed decision-making in the context of clinical trials. By using advanced technologies, this integrated approach can contribute to more efficient, effective, and patient-centric clinical research processes.
Use case 2: Intelligent site monitoring with audio-to-text translation and LLM capabilities
In the HCLS domain, site monitoring plays a crucial role in maintaining the integrity and compliance of clinical trials. Site monitors conduct on-site visits, interview personnel, and verify documentation to assess adherence to protocols and regulatory requirements. However, this process can be time-consuming and prone to errors, particularly when dealing with extensive audio recordings and voluminous documentation.
By integrating audio-to-text translation and LLM capabilities, we can streamline and enhance the site monitoring process, leading to improved efficiency, accuracy, and decision-making support.
Don’t feel like reading the full use case? No problem! You can listen to the key details in our audio file instead.
The process flow consists of the following steps:
- Audio capture and transcription – During site visits, monitors record interviews with site personnel, capturing valuable insights and observations. These audio recordings are then converted into text using ASR and audio-to-text translation technologies. Document ingestion – Relevant site documents, such as patient records, consent forms, and protocol manuals, are digitized and ingested into the system. LLM-powered data analysis – The transcribed interviews and ingested documents are fed into a powerful LLM, which can understand and correlate the information from multiple sources. The LLM can identify key insights, potential issues, and areas of non-compliance by analyzing the content and context of the data. Case report form generation – Based on the LLM’s analysis, a comprehensive case report form (CRF) is generated, summarizing the site visit findings, identifying potential risks or deviations, and providing recommendations for corrective actions or improvements. Decision support and site selection – The CRFs and associated data can be further analyzed by the LLM to identify patterns, trends, and potential risks across multiple sites. This information can be used to support decision-making processes, such as site selection for future clinical trials, based on historical performance and compliance data.
The solution offers the following potential benefits:
- Improved efficiency – By automating the transcription and data analysis processes, site monitors can save significant time and effort, allowing them to focus on more critical tasks and cover more sites within the same time frame. Enhanced accuracy – LLMs can identify and correlate subtle patterns and nuances within the data, reducing the risk of overlooking critical information or making erroneous assumptions. Comprehensive documentation – The generated CRFs provide a standardized and detailed record of site visits, facilitating better communication and collaboration among stakeholders. Regulatory compliance – The LLM-powered analysis can help identify potential areas of non-compliance, enabling proactive measures to address issues and mitigate risks. Informed decision-making – The insights derived from the LLM’s analysis can support data-driven decision-making processes, such as site selection for future clinical trials, based on historical performance and compliance data.
By combining audio-to-text translation and LLM capabilities, this integrated approach offers a powerful solution for intelligent site monitoring in the HCLS domain, supporting improved efficiency, accuracy, and decision-making while providing regulatory compliance and quality assurance.
Use case 3: Enhancing adverse event reporting in clinical trials with audio-to-text and LLMs
Clinical trials are crucial for evaluating the safety and efficacy of investigational drugs and therapies. Accurate and comprehensive adverse event reporting is essential for identifying potential risks and making informed decisions. By combining audio-to-text translation with LLM capabilities, we can streamline and augment the adverse event reporting process, leading to improved patient safety and more efficient clinical research.
Don’t feel like reading the full use case? No problem! You can listen to the key details in our audio file instead.
The process flow consists of the following steps:
- Audio data collection – During clinical trial visits or follow-ups, audio recordings of patient-doctor interactions are captured, capturing detailed descriptions of adverse events or symptoms experienced by the participants. These audio recordings can be obtained through various channels, such as in-person visits, telemedicine consultations, or dedicated voice reporting systems. Audio-to-text transcription – The audio recordings are processed through an audio-to-text translation system, converting the spoken words into written text format. ASR and NLP techniques provide accurate transcription, accounting for factors like accents, background noise, and medical terminology. Text data integration – The transcribed text data is integrated with other sources of adverse event reporting, such as electronic case report forms (eCRFs), patient diaries, and medication logs. This comprehensive dataset provides a holistic view of the adverse events reported across multiple data sources. LLM analysis – The integrated dataset is fed into an LLM specifically trained on medical and clinical trial data. The LLM analyzes the textual data, identifying patterns, extracting relevant information, and generating insights related to adverse event occurrences, severity, and potential causal relationships. Intelligent reporting and decision support – The LLM generates detailed adverse event reports, highlighting key findings, trends, and potential safety signals. These reports can be presented to clinical trial teams, regulatory bodies, and safety monitoring committees, supporting informed decision-making processes. The LLM can also provide recommendations for further investigation, protocol modifications, or risk mitigation strategies based on the identified adverse event patterns.
The solution offers the following potential benefits:
- Improved data capture – By using audio-to-text translation, valuable information from patient-doctor interactions can be captured and included in adverse event reporting, reducing the risk of missed or incomplete data. Enhanced accuracy and completeness – The integration of multiple data sources, combined with the LLM’s analysis capabilities, provides a comprehensive and accurate understanding of adverse events, reducing the potential for errors or omissions. Efficient data analysis – The LLM can rapidly process large volumes of textual data, identifying patterns and insights that might be difficult or time-consuming for human analysts to detect manually. Timely decision support – Real-time adverse event reporting and analysis enable clinical trial teams to promptly identify and address potential safety concerns, mitigating risks and providing participant well-being. Regulatory compliance – Comprehensive adverse event reporting and detailed documentation facilitate compliance with regulatory requirements and support transparent communication with regulatory agencies.
By integrating audio-to-text translation with LLM capabilities, this approach addresses the critical need for accurate and timely adverse event reporting in clinical trials, ultimately enhancing patient safety, improving research efficiency, and supporting informed decision-making in the HCLS domain.
Use case 4: Audio-to-text and LLM integration for enhanced patient care
In the healthcare domain, effective communication and accurate data capture are crucial for providing personalized and high-quality care. By integrating audio-to-text translation capabilities with LLM technology, we can streamline processes and unlock valuable insights, ultimately improving patient outcomes.
Don’t feel like reading the full use case? No problem! You can listen to the key details in our audio file instead.
The process flow consists of the following steps:
- Audio input collection – Caregivers or healthcare professionals can record audio updates on a patient’s condition, mood, or relevant observations using a secure and user-friendly interface. This could be done through mobile devices, dedicated recording stations, or during virtual consultations. Audio-to-text transcription – The recorded audio files are securely transmitted to a speech-to-text engine, which converts the spoken words into text format. Advanced NLP techniques provide accurate transcription, handling accents, medical terminology, and background noise. Text processing and contextualization – The transcribed text is then fed into an LLM trained on various healthcare datasets, including medical literature, clinical guidelines, and deidentified patient records. The LLM processes the text, identifies key information, and extracts relevant context and insights. LLM-powered analysis and recommendations – Using its sizeable knowledge base and natural language understanding capabilities, the LLM can perform various tasks, such as:
- Identifying potential health concerns or risks based on the reported symptoms and observations. Suggesting personalized care plans or treatment options aligned with evidence-based practices. Providing recommendations for follow-up assessments, diagnostic tests, or specialist consultations. Flagging potential drug interactions or contraindications based on the patient’s medical history. Generating summaries or reports in a structured format for efficient documentation and communication.
The solution offers the following potential benefits:
- Improved efficiency – By automating the transcription and analysis process, healthcare professionals can save time and focus on providing personalized care, rather than spending extensive hours on documentation and data entry. Enhanced accuracy – ASR and NLP techniques provide accurate transcription, reducing errors and improving data quality. Comprehensive patient insights – The LLM’s ability to process and contextualize unstructured audio data provides a more holistic understanding of the patient’s condition, enabling better-informed decision-making. Personalized care plans – By using the LLM’s knowledge base and analytical capabilities, healthcare professionals can develop tailored care plans aligned with the patient’s specific needs and medical history. Streamlined communication – Structured reports and summaries generated by the LLM facilitate efficient communication among healthcare teams, making sure everyone has access to the latest patient information. Continuous learning and improvement – As more data is processed, the LLM can continuously learn and refine its recommendations, improving its performance over time.
By integrating audio-to-text translation and LLM capabilities, healthcare organizations can unlock new efficiencies, enhance patient-provider communication, and ultimately deliver superior care while staying at the forefront of technological advancements in the industry.
Use case 5: Audio-to-text translation and LLM integration for clinical trial protocol design
Efficient and accurate protocol design is crucial for successful study execution and regulatory compliance. By combining audio-to-text translation capabilities with the power of LLMs, we can streamline the protocol design process, using diverse data sources and AI-driven insights to create high-quality protocols in a timely manner.
Don’t feel like reading the full use case? No problem! You can listen to the key details in our audio file instead.
The process flow consists of the following steps:
- Audio input collection – Clinical researchers, subject matter experts, and stakeholders provide audio inputs, such as recorded meetings, discussions, or interviews, related to the proposed clinical trial. These audio files can capture valuable insights, requirements, and domain-specific knowledge. Audio-to-text transcription – Using ASR technology, the audio inputs are converted into text transcripts with high accuracy. This step makes sure that valuable information is captured and transformed into a format suitable for further processing by LLMs. Data integration – Relevant data sources, such as previous clinical trial protocols, regulatory guidelines, scientific literature, and medical databases, are integrated into the workflow. These data sources provide contextual information and serve as a knowledge base for the LLM. LLM processing – The transcribed text, along with the integrated data sources, is fed into a powerful LLM. The LLM uses its knowledge base and NLP capabilities to analyze the inputs, identify key elements, and generate a draft clinical trial protocol. Protocol refinement and review – The draft protocol generated by the LLM is reviewed by clinical researchers, medical experts, and regulatory professionals. They provide feedback, make necessary modifications, and enforce compliance with relevant guidelines and best practices. Iterative improvement – As the AI system receives feedback and correlated outcomes from completed clinical trials, it continuously learns and refines its protocol design capabilities. This iterative process enables the LLM to become more accurate and efficient over time, leading to higher-quality protocol designs.
The solution offers the following potential benefits:
- Efficiency – By automating the initial protocol design process, researchers can save valuable time and resources, allowing them to focus on more critical aspects of clinical trial execution. Accuracy and consistency – LLMs can use vast amounts of data and domain-specific knowledge, reducing the risk of errors and providing consistency across protocols. Knowledge integration – The ability to seamlessly integrate diverse data sources, including audio recordings, scientific literature, and regulatory guidelines, enhances the quality and comprehensiveness of the protocol design. Continuous improvement – The iterative learning process allows the AI system to adapt and improve its protocol design capabilities based on real-world outcomes, leading to increasingly accurate and effective protocols over time. Decision-making support – By providing well-structured and comprehensive protocols, the AI-driven approach enables better-informed decision-making for clinical researchers, sponsors, and regulatory bodies.
This integrated approach using audio-to-text translation and LLM capabilities has the potential to revolutionize the clinical trial protocol design process, ultimately contributing to more efficient and successful clinical trials, accelerating the development of life-saving treatments, and improving patient outcomes.
Use case 6: Voice-enabled clinical trial and disease information assistant
In the HCLS domain, effective communication and access to accurate information are crucial for patients, caregivers, and healthcare professionals. This use case demonstrates how audio-to-text translation combined with LLM capabilities can address these needs by providing an intelligent, voice-enabled assistant for clinical trial and disease information.
Don’t feel like reading the full use case? No problem! You can listen to the key details in our audio file instead.
The process flow consists of the following steps:
- Audio input – The user, whether a patient, caregiver, or healthcare professional, can initiate the process by providing a voice query related to a specific disease or clinical trial. This could include questions about the disease itself, treatment options, ongoing trials, eligibility criteria, or other relevant information. Audio-to-text translation – The audio input is converted into text using state-of-the-art speech recognition technology. This step makes sure that the user’s query is accurately transcribed and ready for further processing by the LLM. Data integration – The system integrates various data sources, including clinical trial data, disease-specific information from reputable sources (such as PubMed or WebMD), and other relevant third-party resources. This comprehensive data integration makes sure that the LLM has access to a large knowledge base for generating accurate and comprehensive responses. LLM processing – The transcribed query is fed into the LLM, which uses its natural language understanding capabilities to comprehend the user’s intent and extract relevant information from the integrated data sources. The LLM can provide intelligent responses, insights, and recommendations based on the query and the available data. Response generation – The LLM generates a detailed, context-aware response addressing the user’s query. This response can be presented in various formats, such as text, audio (using text-to-speech technology), or a combination of both, depending on the user’s preferences and accessibility needs. Feedback and continuous improvement – The system can incorporate user feedback mechanisms to improve its performance over time. This feedback can be used to refine the LLM’s understanding, enhance the data integration process, and make sure that the system remains up to date with the latest clinical trial and disease information.
The solution offers the following potential benefits:
- Improved access to information – By using voice input and NLP capabilities, the system empowers patients, caregivers, and healthcare professionals to access accurate and comprehensive information about diseases and clinical trials, regardless of their technical expertise or literacy levels. Enhanced communication – The voice-enabled interface facilitates seamless communication between users and the system, enabling them to ask questions and receive responses in a conversational manner, mimicking human-to-human interaction. Personalized insights – The LLM can provide personalized insights and recommendations based on the user’s specific query and context, enabling more informed decision-making and tailored support for individuals. Time and efficiency gains – By automating the process of information retrieval and providing intelligent responses, the system can significantly reduce the time and effort required for healthcare professionals to manually search and synthesize information from multiple sources. Improved patient engagement – By offering accessible and user-friendly access to disease and clinical trial information, the system can empower patients and caregivers to actively participate in their healthcare journey, fostering better engagement and understanding.
This use case highlights the potential of integrating audio-to-text translation with LLM capabilities to address real-world challenges in the HCLS domain. By using cutting-edge technologies, this solution can improve information accessibility, enhance communication, and support more informed decision-making for all stakeholders involved in clinical trials and disease management.
For the demonstration purpose we will focus on following use case:
Use case overview: Patient reporting and analysis in clinical trials
In clinical trials, it’s crucial to gather accurate and comprehensive patient data to assess the safety and efficacy of investigational drugs or therapies. Traditional methods of collecting patient reports can be time-consuming, prone to errors, and might result in incomplete or inconsistent data. By combining audio-to-text translation with LLM capabilities, we can streamline the patient reporting process and unlock valuable insights to support decision-making.
Don’t feel like reading the full use case? No problem! You can listen to the key details in our audio file instead.
The process flow consists of the following steps:
- Audio input – Patients participating in clinical trials can provide their updates, symptoms, and feedback through voice recordings using a mobile application or a dedicated recording device. Audio-to-text transcription – The recorded audio files are securely transmitted to a cloud-based infrastructure, where they undergo automated transcription using ASR technology. The audio is converted into text, providing accurate and verbatim transcripts. Data consolidation – The transcribed patient reports are consolidated into a structured database, enabling efficient storage, retrieval, and analysis. LLM processing – The consolidated textual data is then processed by an LLM trained on biomedical and clinical trial data. The LLM can perform various tasks, including:
- Natural language processing – Extracting relevant information and identifying key symptoms, adverse events, or treatment responses from the patient reports. Sentiment analysis – Analyzing the emotional and psychological state of patients based on their language and tone, which can provide valuable insights into their overall well-being and treatment experience. Pattern recognition – Identifying recurring themes, trends, or anomalies across multiple patient reports, enabling early detection of potential safety concerns or efficacy signals. Knowledge extraction – Using the LLM’s understanding of biomedical concepts and clinical trial protocols to derive meaningful insights and recommendations from the patient data.
The solution offers the following potential benefits:
- Improved data quality – By using audio-to-text transcription, the risk of errors and inconsistencies associated with manual data entry is minimized, providing high-quality patient data. Time and cost-efficiency – Automated transcription and LLM-powered analysis can significantly reduce the time and resources required for data collection, processing, and analysis, leading to faster decision-making and cost savings. Enhanced patient experience – Patients can provide their updates conveniently through voice recordings, reducing the burden of manual data entry and enabling more natural communication. Comprehensive analysis – The combination of NLP, sentiment analysis, and pattern recognition capabilities offered by LLMs allows for a holistic understanding of patient experiences, treatment responses, and potential safety signals. Regulatory compliance – Accurate and comprehensive patient data, coupled with robust analysis, can support compliance with regulatory requirements for clinical trial reporting and data documentation.
By integrating audio-to-text translation and LLM capabilities, clinical trial sponsors and research organizations can benefit from streamlined patient reporting, enhanced data quality, and powerful insights to support informed decision-making throughout the clinical development process.
Solution overview
The following diagram illustrates the solution architecture.
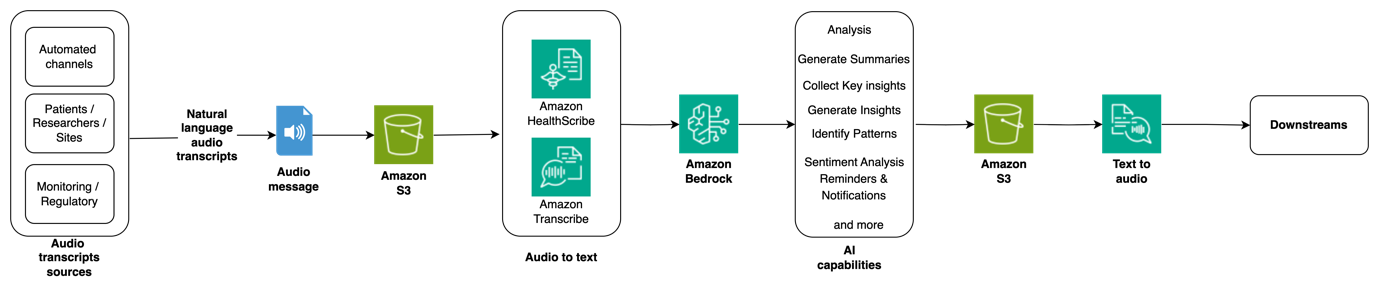
Solution overview: patient reporting and analysis in clinical trials
Key AWS services used in this solution include Amazon Simple Storage Service (Amazon S3), AWS HealthScribe, Amazon Transcribe, and Amazon Bedrock.
Prerequisites
This solution requires the following prerequisites:
- A basic understanding of clinical trails in healthcare. An AWS account. If you don’t have one, you can register for a new AWS account. Access to Anthropic’s Claude 3 Sonnet model on Amazon Bedrock (model ID: claude-3-sonnet-20240229-v1:0). For more details, see Add or remove access to Amazon Bedrock foundation models.
Data samples
To illustrate the concept and provide a practical understanding, we have curated a collection of audio samples. These samples serve as representative examples, simulating site interviews conducted by researchers at clinical trial sites with patient participants.
The audio recordings offer a glimpse into the type of data typically encountered during such interviews. We encourage you to listen to these samples to gain a better appreciation of the data and its context.
These samples are for demonstration purposes only and don’t contain any real patient information or sensitive data. They are intended solely to provide a sample structure and format for the audio recordings used in this particular use case.
Sample Data | Audio File |
Site interview 1 | |
Site Interview 2 | |
Site Interview 3 | |
Site Interview 4 | |
Site Interview 5 |
Prompt templates
Prior to deploying and executing this solution, it’s essential to comprehend the input prompts and the anticipated output from the LLM. Although this is merely a sample, the potential outcomes and possibilities can be vastly expanded by crafting creative prompts.
We use the following input prompt template:
You are an expert medical research analyst for clinical trials of medicines. \ You will be provided with a dictionary containing text transcriptions of clinical trial interviews conducted between patients and interviewers. \ The dictionary keys represent the interview_id, and the values contain the interview transcripts.\ \ <interview_transcripts>add_interview_transcripts</interview_transcripts>\ \ Your task is to analyze all the transcripts and generate a comprehensive report summarizing the key findings and conclusions from the clinical trial. |
The response Amazon Bedrock will be as below:
Based on the interview transcripts provided, here is a comprehensive report summarizing the key findings and conclusions from the clinical trial: Introduction: This report analyzes transcripts from interviews conducted with patients participating in a clinical trial for a new investigational drug. The interviews cover various aspects of the trial, including the informed consent process, randomization procedures, dosing schedules, follow-up visits, and patient experiences with potential side effects. Key Findings: 1. Informed Consent Process: – The informed consent process was thorough, with detailed explanations provided to patients about the trial’s procedures, potential risks, and benefits (Transcript 5). – Patients were given ample time to review the consent documents, discuss them with family members, and have their questions addressed satisfactorily by the study team (Transcript 5). – Overall, patients felt they fully understood the commitments and requirements of participating in the trial (Transcript 5). 2. Randomization and Blinding: – Patients were randomized to either receive the investigational drug or a placebo, as part of a placebo-controlled study design (Transcript 2). – The randomization process was adequately explained to patients, and they understood the rationale behind blinding, which is to prevent bias in the results (Transcript 2). – Patients expressed acceptance of the possibility of receiving a placebo, recognizing its importance for the research (Transcript 2). 3. Dosing Schedule and Adherence: – The dosing schedule involved taking the medication twice daily, in the morning and evening (Transcript 4). – Some patients reported occasional difficulties in remembering the evening dose but implemented strategies like setting reminders on their phones to improve adherence (Transcript 4). 4. Follow-up Visits and Assessments: – Follow-up visits were scheduled at specific intervals, such as 30 days, 3 months, and 6 months after the last dose (Transcripts 1 and 3). – During these visits, various assessments were conducted, including blood tests, physical exams, ECGs, and evaluation of patient-reported outcomes like pain levels (Transcripts 1 and 3). – Patients were informed that they would receive clinically significant findings from these assessments (Transcript 3). 5. Patient-Reported Side Effects: – Some patients reported experiencing mild side effects, such as headaches, nausea, and joint pain improvement (Transcripts 3 and 4). – The study team diligently documented and monitored these side effects, noting them in case report forms for further evaluation (Transcript 4). 6. Study Conduct and Communication: – The study team provided 24/7 contact information, allowing patients to reach out with concerns between scheduled visits (Transcript 1). – Patients were informed that they would receive information about the overall study results once available (Transcript 1). – Patients were made aware of their ability to withdraw from the study at any time if they became uncomfortable (Transcript 2). Conclusions: Based on the interview transcripts, the clinical trial appears to have been conducted in a thorough and ethical manner, adhering to principles of informed consent, randomization, and blinding. Patients were adequately informed about the trial procedures, potential risks, and their rights as participants. The study team diligently monitored patient safety, documented adverse events, and maintained open communication channels. Overall, the transcripts suggest a well-managed clinical trial with a focus on patient safety, data integrity, and adherence to research protocols. |
Deploy resources with AWS CloudFormation
To deploy the solution, use AWS CloudFormation template
Test the application
To test the application, complete the following steps:
- On the Amazon S3 console, choose Buckets in the navigation pane. Locate your bucket starting with blog-hcls-assets-*. Navigate to the S3 prefix hcls-framework/samples-input-audio/. You will see sample audio files, which we reviewed earlier in this post. Select these files, and on the Actions menu, choose Copy.
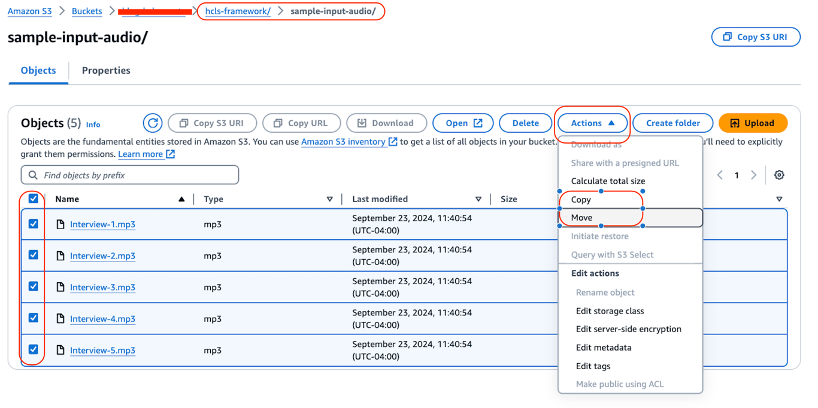
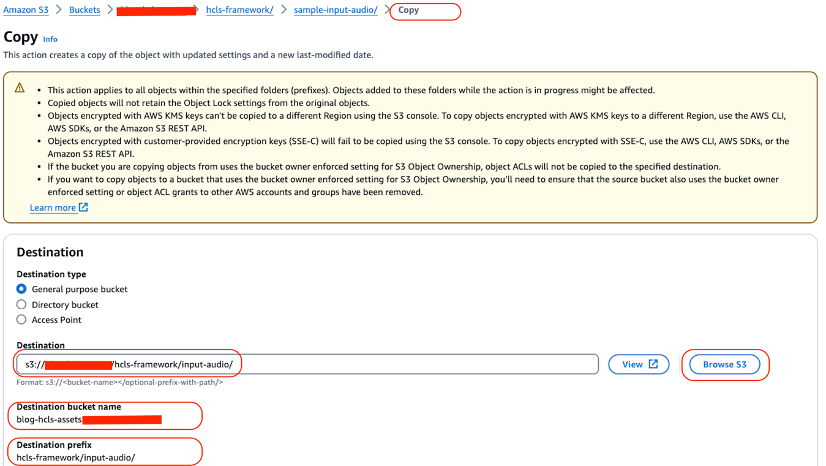
Copying these sample files will trigger an S3 event invoking the AWS Lambda function audio-to-text. To review the invocations of the Lambda function on the AWS Lambda console, navigate to the audio-to-text function and then the Monitor tab, which contains detailed logs.
You can review the status of the Amazon Transcribe jobs on the Amazon Transcribe console.
At this step, the interview transcripts are ready. They should be available in Amazon S3 under the prefix hcls-framework/input-text/.
You can download a sample file and review the contents. You will notice the content of this file as JSON with a text transcript available under the key transcripts, along with other metadata.
Now let’s run Anthropic’s Claude 3 Sonnet using the Lambda function hcls_clinical_trial_analysis to analyze the transcripts and generate a comprehensive report summarizing the key findings and conclusions from the clinical trial.
- On the Lambda console, navigate to the function named hcls_clinical_trial_analysis. Choose Test. If the console prompts you to create a new test event, do so with default or no input to the test event.
- Run the test event.
To review the output, open the Lambda console and navigate to the function named hcls_clinical_trial_analysis, and then on the Monitor tab, for detailed logs, choose View CloudWatch Logs. In the logs, you will see your comprehensive report on the clinical trial.
So far, we have completed a process involving:
- Collecting audio interviews from clinical trials Transcribing the audio to text Compiling transcripts into a dictionary Using Amazon Bedrock (Anthropic’s Claude 3 Sonnet) to generate a comprehensive summary
Although we focused on summarization, this approach can be extended to other applications such as sentiment analysis, extracting key learnings, identifying common complaints, and more.
Summary
Healthcare patients often find themselves in need of reliable information about their conditions, clinical trials, or treatment options. However, accessing accurate and up-to-date medical knowledge can be a daunting task. Our innovative solution integrates cutting-edge audio-to-text translation and LLM capabilities to revolutionize how patients receive vital healthcare information. By using speech recognition technology, we can accurately transcribe patients’ spoken queries, allowing our LLM to comprehend the context and provide personalized, evidence-based responses tailored to their specific needs. This empowers patients to make informed decisions, enhances accessibility for those with disabilities or preferences for verbal communication, and alleviates the workload on healthcare professionals, ultimately improving patient outcomes and driving progress in the HCLS domain.
Take charge of your healthcare journey with our innovative voice-enabled virtual assistant. Empower yourself with accurate and personalized information by simply asking your questions aloud. Our cutting-edge solution integrates speech recognition and advanced language models to provide reliable, context-aware responses tailored to your specific needs. Embrace the future of healthcare today and experience the convenience of instantaneous access to vital medical information.
About the Authors
Vrinda Dabke leads AWS Professional Services North America Delivery. Prior to joining AWS, Vrinda held a variety of leadership roles in Fortune 100 companies like UnitedHealth Group, The Hartford, Aetna, and Pfizer. Her work has been focused on in the areas of business intelligence, analytics, and AI/ML. She is a motivational people leader with experience in leading and managing high-performing global teams in complex matrix organizations.
Kannan Raman leads the North America Delivery for AWS Professional Services Healthcare and Life Sciences practice at AWS. He has over 24 years of healthcare and life sciences experience and provides thought leadership in digital transformation. He works with C level customer executives to help them with their digital transformation agenda.
Rushabh Lokhande is a Senior Data & ML Engineer with AWS Professional Services Analytics Practice. He helps customers implement big data, machine learning, and analytics solutions. Outside of work, he enjoys spending time with family, reading, running, and playing golf.
Bruno Klein is a Senior Machine Learning Engineer with AWS Professional Services Analytics Practice. He helps customers implement big data and analytics solutions. Outside of work, he enjoys spending time with family, traveling, and trying new food.