In today’s rapidly evolving technological landscape, developers and organizations often grapple with a series of practical challenges. One of the most significant hurdles is the efficient processing of diverse data types—text, speech, and vision—within a single system. Traditional approaches have typically required separate pipelines for each modality, leading to increased complexity, higher latency, and greater computational costs. In many applications—from healthcare diagnostics to financial analytics—these limitations can hinder the development of responsive and adaptive AI solutions. The need for models that balance robustness with efficiency is more pressing than ever. In this context, Microsoft’s recent work on small language models (SLMs) provides a promising approach by striving to consolidate capabilities in a compact, versatile package.
Microsoft AI has recently introduced Phi-4-multimodal and Phi-4-mini, the newest additions to its Phi family of SLMs. These models have been developed with a clear focus on streamlining multimodal processing. Phi-4-multimodal is designed to handle text, speech, and visual inputs concurrently, all within a unified architecture. This integrated approach means that a single model can now interpret and generate responses based on varied data types without the need for separate, specialized systems.
In contrast, Phi-4-mini is tailored specifically for text-based tasks. Despite being more compact, it has been engineered to excel in reasoning, coding, and instruction following. Both models are made accessible via platforms like Azure AI Foundry and Hugging Face, ensuring that developers from a range of industries can experiment with and integrate these models into their applications. This balanced release represents a thoughtful step towards making advanced AI more practical and accessible.
Technical Details and Benefits
At the technical level, Phi-4-multimodal is a 5.6-billion-parameter model that incorporates a mixture-of-LoRAs—a method that allows the integration of speech, vision, and text within a single representation space. This design significantly simplifies the architecture by removing the need for separate processing pipelines. As a result, the model not only reduces computational overhead but also achieves lower latency, which is particularly beneficial for real-time applications.
Phi-4-mini, with its 3.8-billion parameters, is built as a dense, decoder-only transformer. It features grouped-query attention and boasts a vocabulary of 200,000 tokens, enabling it to handle sequences of up to 128,000 tokens. Despite its smaller size, Phi-4-mini performs remarkably well in tasks that require deep reasoning and language understanding. One of its standout features is the capability for function calling—allowing it to interact with external tools and APIs, thus extending its practical utility without requiring a larger, more resource-intensive model.
Both models have been optimized for on-device execution. This optimization is particularly important for applications in environments with limited compute resources or in edge computing scenarios. The models’ reduced computational requirements make them a cost-effective choice, ensuring that advanced AI functionalities can be deployed even on devices that do not have extensive processing capabilities.
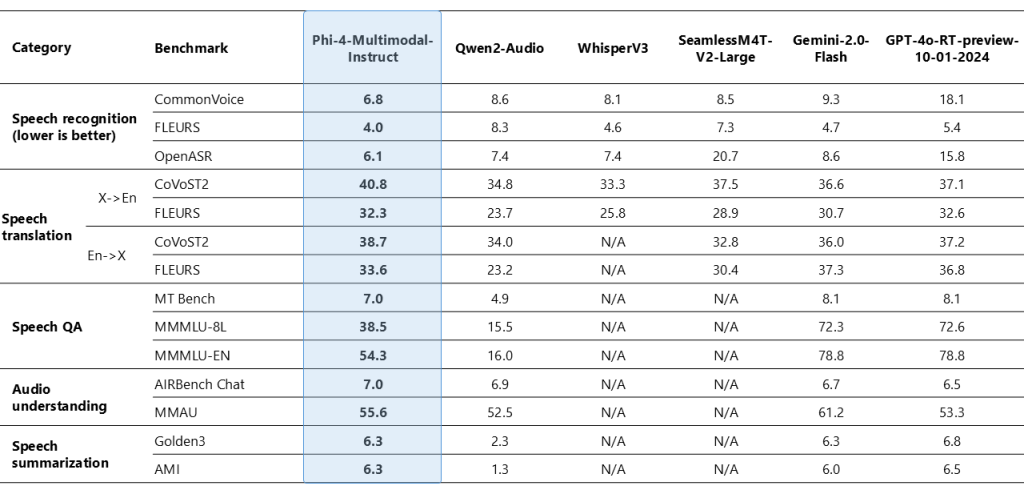
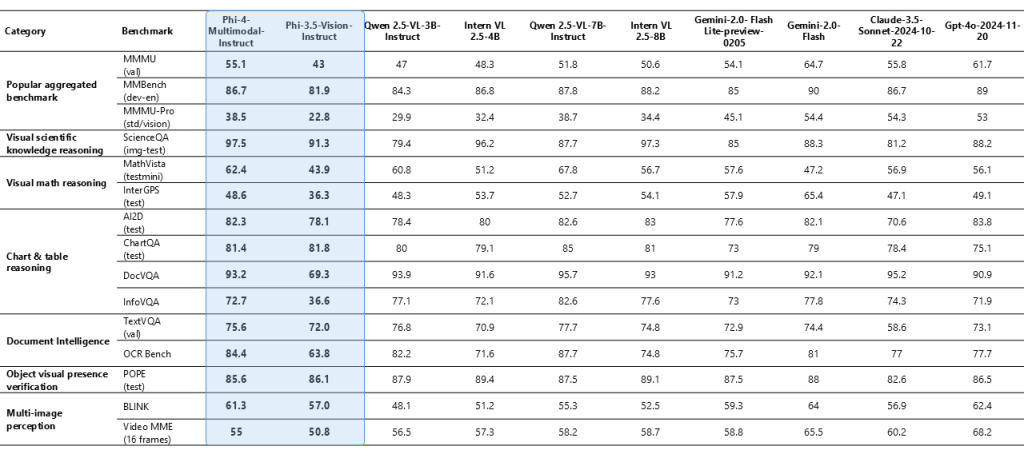
Performance Insights and Benchmark Data
Benchmark results provide a clear view of how these models perform in practical scenarios. For instance, Phi-4-multimodal has demonstrated an impressive word error rate (WER) of 6.14% in automatic speech recognition (ASR) tasks. This is a modest improvement over previous models like WhisperV3, which reported a WER of 6.5%. Such improvements are particularly significant in applications where accuracy in speech recognition is critical.
Beyond ASR, Phi-4-multimodal also shows robust performance in tasks such as speech translation and summarization. Its ability to process visual inputs is notable in tasks like document reasoning, chart understanding, and optical character recognition (OCR). In several benchmarks—ranging from synthetic speech interpretation on visual data to document analysis—the model’s performance consistently aligns with or exceeds that of larger, more resource-intensive models.
Similarly, Phi-4-mini has been evaluated on a variety of language benchmarks, where it holds its own despite its more compact design. Its aptitude for reasoning, handling complex mathematical problems, and coding tasks underlines its versatility in text-based applications. The inclusion of a function-calling mechanism further enriches its potential, enabling the model to draw on external data and tools seamlessly. These results underscore a measured and thoughtful improvement in multimodal and language processing capabilities, providing clear benefits without overstating its performance.
Conclusion
The introduction of Phi-4-multimodal and Phi-4-mini by Microsoft marks an important evolution in the field of AI. Rather than relying on bulky, resource-demanding architectures, these models offer a refined balance between efficiency and performance. By integrating multiple modalities in a single, cohesive framework, Phi-4-multimodal simplifies the complexity inherent in multimodal processing. Meanwhile, Phi-4-mini provides a robust solution for text-intensive tasks, proving that smaller models can indeed offer significant capabilities.
Check out the Technical details and Model on Hugging Face. All credit for this research goes to the researchers of this project. Also, feel free to follow us on Twitter and don’t forget to join our 80k+ ML SubReddit.
The post Microsoft AI Releases Phi-4-multimodal and Phi-4-mini: The Newest Models in Microsoft’s Phi Family of Small Language Models (SLMs) appeared first on MarkTechPost.