Just as there are widely understood empirical laws of nature — for example, what goes up must come down, or every action has an equal and opposite reaction — the field of AI was long defined by a single idea: that more compute, more training data and more parameters makes a better AI model.
However, AI has since grown to need three distinct laws that describe how applying compute resources in different ways impacts model performance. Together, these AI scaling laws — pretraining scaling, post-training scaling and test-time scaling, also called long thinking — reflect how the field has evolved with techniques to use additional compute in a wide variety of increasingly complex AI use cases.
The recent rise of test-time scaling — applying more compute at inference time to improve accuracy — has enabled AI reasoning models, a new class of large language models (LLMs) that perform multiple inference passes to work through complex problems, while describing the steps required to solve a task. Test-time scaling requires intensive amounts of computational resources to support AI reasoning, which will drive further demand for accelerated computing.
What Is Pretraining Scaling?
Pretraining scaling is the original law of AI development. It demonstrated that by increasing training dataset size, model parameter count and computational resources, developers could expect predictable improvements in model intelligence and accuracy.
Each of these three elements — data, model size, compute — is interrelated. Per the pretraining scaling law, outlined in this research paper, when larger models are fed with more data, the overall performance of the models improves. To make this feasible, developers must scale up their compute — creating the need for powerful accelerated computing resources to run those larger training workloads.
This principle of pretraining scaling led to large models that achieved groundbreaking capabilities. It also spurred major innovations in model architecture, including the rise of billion- and trillion-parameter transformer models, mixture of experts models and new distributed training techniques — all demanding significant compute.
And the relevance of the pretraining scaling law continues — as humans continue to produce growing amounts of multimodal data, this trove of text, images, audio, video and sensor information will be used to train powerful future AI models.
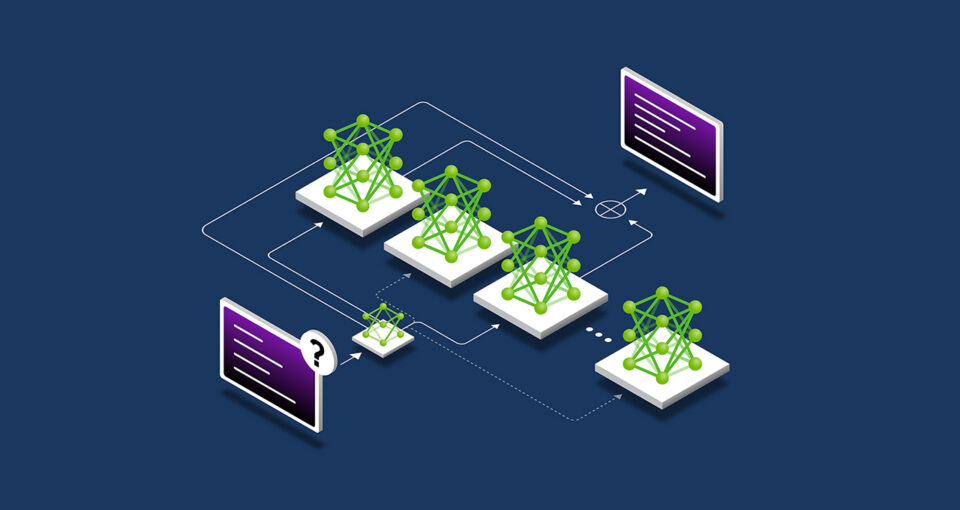
What Is Post-Training Scaling?
Pretraining a large foundation model isn’t for everyone — it takes significant investment, skilled experts and datasets. But once an organization pretrains and releases a model, they lower the barrier to AI adoption by enabling others to use their pretrained model as a foundation to adapt for their own applications.
This post-training process drives additional cumulative demand for accelerated computing across enterprises and the broader developer community. Popular open-source models can have hundreds or thousands of derivative models, trained across numerous domains.
Developing this ecosystem of derivative models for a variety of use cases could take around 30x more compute than pretraining the original foundation model.
Developing this ecosystem of derivative models for a variety of use cases could take around 30x more compute than pretraining the original foundation model.
Post-training techniques can further improve a model’s specificity and relevance for an organization’s desired use case. While pretraining is like sending an AI model to school to learn foundational skills, post-training enhances the model with skills applicable to its intended job. An LLM, for example, could be post-trained to tackle a task like sentiment analysis or translation — or understand the jargon of a specific domain, like healthcare or law.
The post-training scaling law posits that a pretrained model’s performance can further improve — in computational efficiency, accuracy or domain specificity — using techniques including fine-tuning, pruning, quantization, distillation, reinforcement learning and synthetic data augmentation.
- Fine-tuning uses additional training data to tailor an AI model for specific domains and applications. This can be done using an organization’s internal datasets, or with pairs of sample model input and outputs.Distillation requires a pair of AI models: a large, complex teacher model and a lightweight student model. In the most common distillation technique, called offline distillation, the student model learns to mimic the outputs of a pretrained teacher model.Reinforcement learning, or RL, is a machine learning technique that uses a reward model to train an agent to make decisions that align with a specific use case. The agent aims to make decisions that maximize cumulative rewards over time as it interacts with an environment — for example, a chatbot LLM that is positively reinforced by “thumbs up” reactions from users. This technique is known as reinforcement learning from human feedback (RLHF). Another, newer technique, reinforcement learning from AI feedback (RLAIF), instead uses feedback from AI models to guide the learning process, streamlining post-training efforts.Best-of-n sampling generates multiple outputs from a language model and selects the one with the highest reward score based on a reward model. It’s often used to improve an AI’s outputs without modifying model parameters, offering an alternative to fine-tuning with reinforcement learning.Search methods explore a range of potential decision paths before selecting a final output. This post-training technique can iteratively improve the model’s responses.
To support post-training, developers can use synthetic data to augment or complement their fine-tuning dataset. Supplementing real-world datasets with AI-generated data can help models improve their ability to handle edge cases that are underrepresented or missing in the original training data.
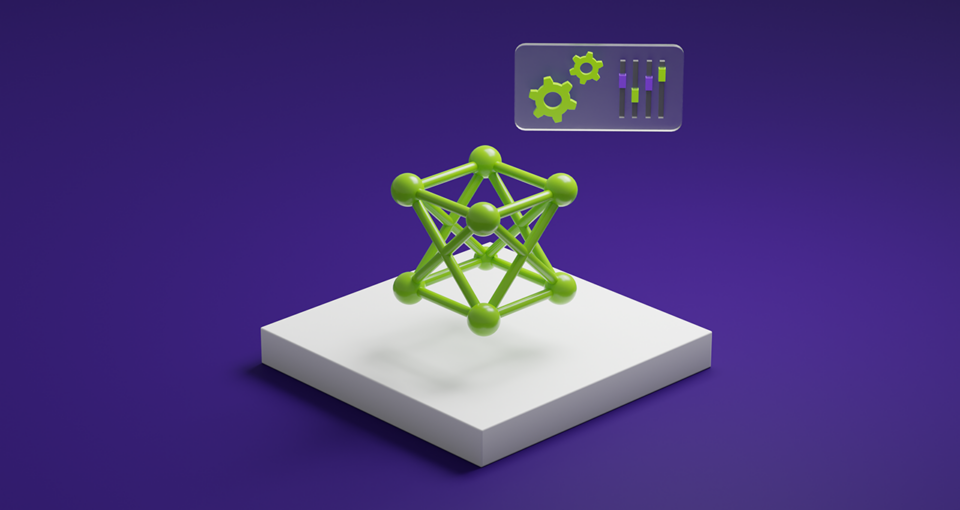
What Is Test-Time Scaling?
LLMs generate quick responses to input prompts. While this process is well suited for getting the right answers to simple questions, it may not work as well when a user poses complex queries. Answering complex questions — an essential capability for agentic AI workloads — requires the LLM to reason through the question before coming up with an answer.
It’s similar to the way most humans think — when asked to add two plus two, they provide an instant answer, without needing to talk through the fundamentals of addition or integers. But if asked on the spot to develop a business plan that could grow a company’s profits by 10%, a person will likely reason through various options and provide a multistep answer.
Test-time scaling, also known as long thinking, takes place during inference. Instead of traditional AI models that rapidly generate a one-shot answer to a user prompt, models using this technique allocate extra computational effort during inference, allowing them to reason through multiple potential responses before arriving at the best answer.
On tasks like generating complex, customized code for developers, this AI reasoning process can take multiple minutes, or even hours — and can easily require over 100x compute for challenging queries compared to a single inference pass on a traditional LLM, which would be highly unlikely to produce a correct answer in response to a complex problem on the first try.
This AI reasoning process can take multiple minutes, or even hours — and can easily require over 100x compute for challenging queries compared to a single inference pass on a traditional LLM.
This test-time compute capability enables AI models to explore different solutions to a problem and break down complex requests into multiple steps — in many cases, showing their work to the user as they reason. Studies have found that test-time scaling results in higher-quality responses when AI models are given open-ended prompts that require several reasoning and planning steps.
The test-time compute methodology has many approaches, including:
- Chain-of-thought prompting: Breaking down complex problems into a series of simpler steps.
- Sampling with majority voting: Generating multiple responses to the same prompt, then selecting the most frequently recurring answer as the final output.
- Search: Exploring and evaluating multiple paths present in a tree-like structure of responses.
Post-training methods like best-of-n sampling can also be used for long thinking during inference to optimize responses in alignment with human preferences or other objectives.
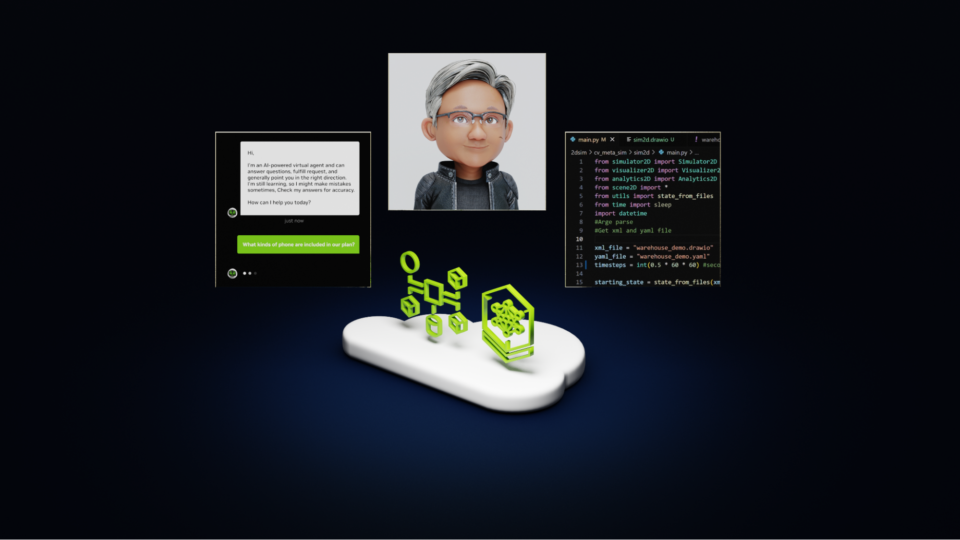
How Test-Time Scaling Enables AI Reasoning
The rise of test-time compute unlocks the ability for AI to offer well-reasoned, helpful and more accurate responses to complex, open-ended user queries. These capabilities will be critical for the detailed, multistep reasoning tasks expected of autonomous agentic AI and physical AI applications. Across industries, they could boost efficiency and productivity by providing users with highly capable assistants to accelerate their work.
In healthcare, models could use test-time scaling to analyze vast amounts of data and infer how a disease will progress, as well as predict potential complications that could stem from new treatments based on the chemical structure of a drug molecule. Or, it could comb through a database of clinical trials to suggest options that match an individual’s disease profile, sharing its reasoning process about the pros and cons of different studies.
In retail and supply chain logistics, long thinking can help with the complex decision-making required to address near-term operational challenges and long-term strategic goals. Reasoning techniques can help businesses reduce risk and address scalability challenges by predicting and evaluating multiple scenarios simultaneously — which could enable more accurate demand forecasting, streamlined supply chain travel routes, and sourcing decisions that align with an organization’s sustainability initiatives.
And for global enterprises, this technique could be applied to draft detailed business plans, generate complex code to debug software, or optimize travel routes for delivery trucks, warehouse robots and robotaxis.
AI reasoning models are rapidly evolving. OpenAI o1-mini and o3-mini, DeepSeek R1, and Google DeepMind’s Gemini 2.0 Flash Thinking were all introduced in the last few weeks, and additional new models are expected to follow soon.
Models like these require considerably more compute to reason during inference and generate correct answers to complex questions — which means that enterprises need to scale their accelerated computing resources to deliver the next generation of AI reasoning tools that can support complex problem-solving, coding and multistep planning.
Learn about the benefits of NVIDIA AI for accelerated inference.