In this post, we discuss what embeddings are, show how to practically use language embeddings, and explore how to use them to add functionality such as zero-shot classification and semantic search. We then use Amazon Bedrock and language embeddings to add these features to a really simple syndication (RSS) aggregator application.
Amazon Bedrock is a fully managed service that makes foundation models (FMs) from leading AI startups and Amazon available through an API, so you can choose from a wide range of FMs to find the model that is best suited for your use case. Amazon Bedrock offers a serverless experience, so you can get started quickly, privately customize FMs with your own data, and integrate and deploy them into your applications using Amazon Web Services (AWS) services without having to manage infrastructure. For this post, we use the Cohere v3 Embed model on Amazon Bedrock to create our language embeddings.
Use case: RSS aggregator
To demonstrate some of the possible uses of these language embeddings, we developed an RSS aggregator website. RSS is a web feed that allows publications to publish updates in a standardized, computer-readable way. On our website, users can subscribe to an RSS feed and have an aggregated, categorized list of the new articles. We use embeddings to add the following functionalities:
- Zero-shot classification – Articles are classified between different topics. There are some default topics, such as Technology, Politics, and Health & Wellbeing, as shown in the following screenshot. Users can also create their own topics.
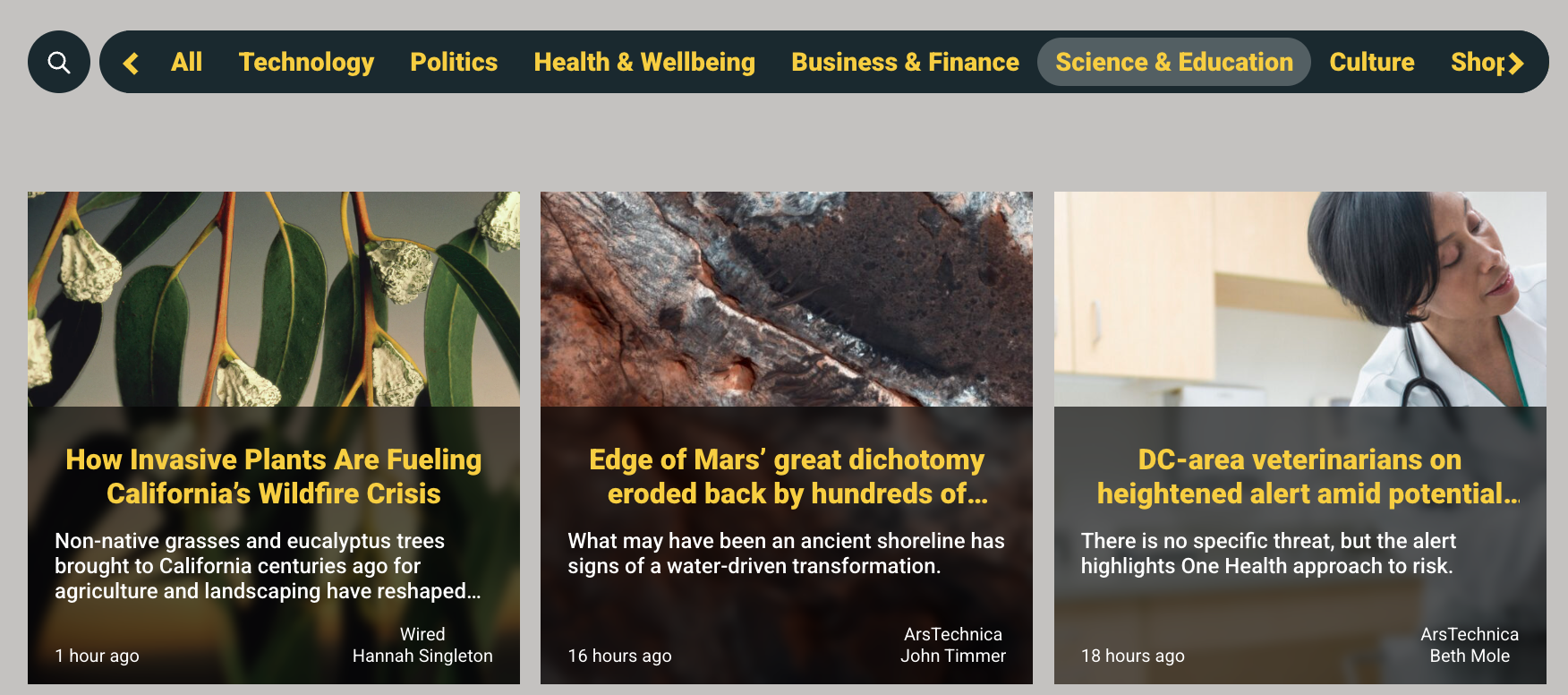
- Semantic search – Users can search their articles using semantic search, as shown in the following screenshot. Users can not only search for a specific topic but also narrow their search by factors such as tone or style.
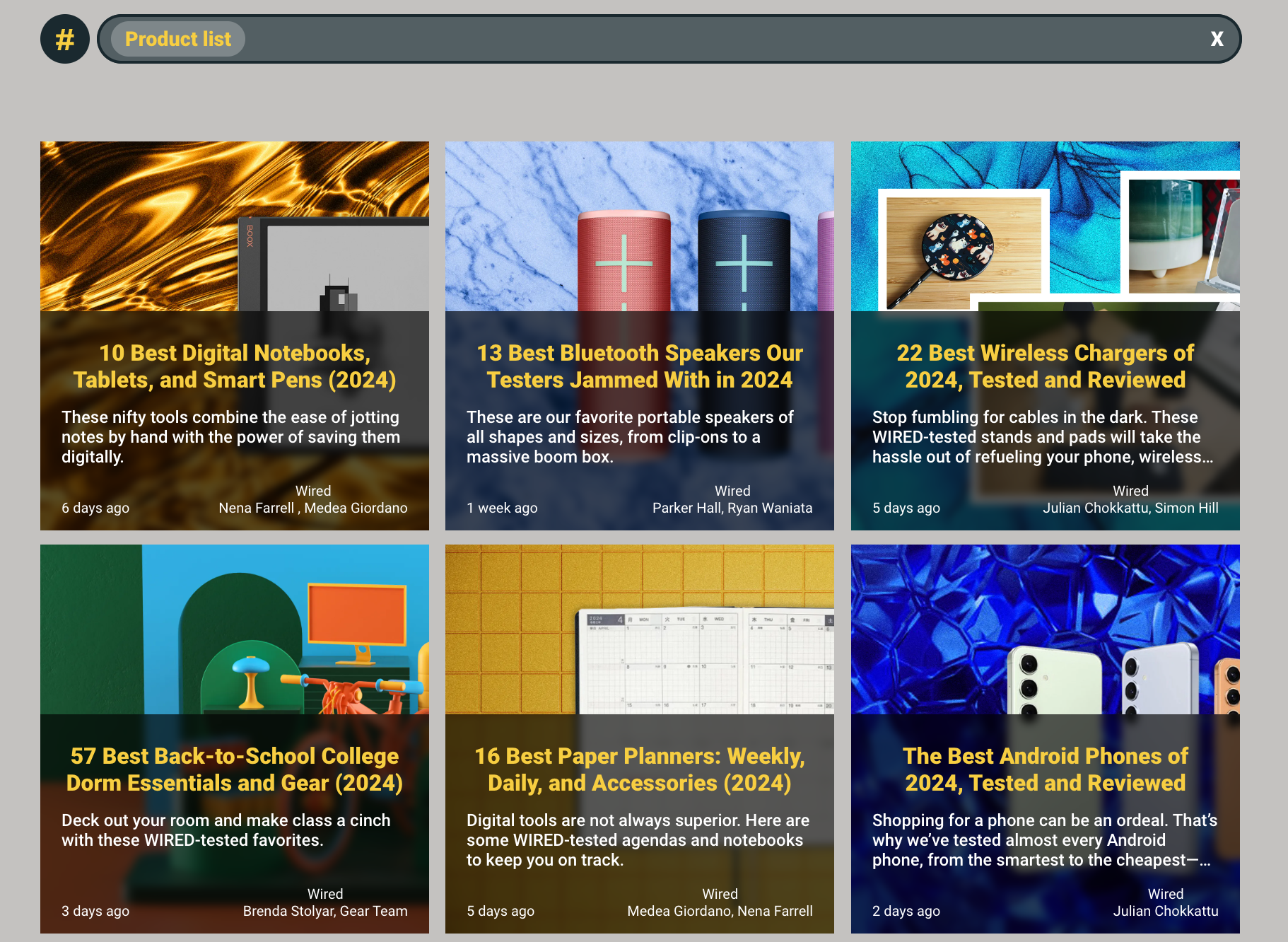
This post uses this application as a reference point to discuss the technical implementation of the semantic search and zero-shot classification features.
Solution overview
This solution uses the following services:
- Amazon API Gateway – The API is accessible through Amazon API Gateway. Caching is performed on Amazon CloudFront for certain topics to ease the database load. Amazon Bedrock with Cohere v3 Embed – The articles and topics are converted into embeddings with the help of Amazon Bedrock and Cohere v3 Embed. Amazon CloudFront and Amazon Simple Storage Service (Amazon S3) – The single-page React application is hosted using Amazon S3 and Amazon CloudFront. Amazon Cognito – Authentication is done using Amazon Cognito user pools. Amazon EventBridge – Amazon EventBridge and EventBridge schedules are used to coordinate new updates. AWS Lambda – The API is a Fastify application written in TypeScript. It’s hosted on AWS Lambda. Amazon Aurora PostgreSQL-Compatible Edition and pgvector – Amazon Aurora PostgreSQL-Compatible is used as the database, both for the functionality of the application itself and as a vector store using pgvector. Amazon RDS Proxy – Amazon RDS Proxy is used for connection pooling. Amazon Simple Queue Service (Amazon SQS) – Amazon SQS is used to queue events. It consumes one event at a time so it doesn’t hit the rate limit of Cohere in Amazon Bedrock.
The following diagram illustrates the solution architecture.
What are embeddings?
This section offers a quick primer on what embeddings are and how they can be used.
Embeddings are numerical representations of concepts or objects, such as language or images. In this post, we discuss language embeddings. By reducing these concepts to numerical representations, we can then use them in a way that a computer can understand and operate on.
Let’s take Berlin and Paris as an example. As humans, we understand the conceptual links between these two words. Berlin and Paris are both cities, they’re capitals of their respective countries, and they’re both in Europe. We understand their conceptual similarities almost instinctively, because we can create a model of the world in our head. However, computers have no built-in way of representing these concepts.
To represent these concepts in a way a computer can understand, we convert them into language embeddings. Language embeddings are high dimensional vectors that learn their relationships with each other through the training of a neural network. During training, the neural network is exposed to enormous amounts of text and learns patterns based on how words are colocated and relate to each other in different contexts.
Embedding vectors allow computers to model the world from language. For instance, if we embed “Berlin” and “Paris,” we can now perform mathematical operations on these embeddings. We can then observe some fairly interesting relationships. For instance, we could do the following: Paris – France + Germany ~= Berlin. This is because the embeddings capture the relationships between the words “Paris” and “France” and between “Germany” and “Berlin”—specifically, that Paris and Berlin are both capital cities of their respective countries.
The following graph shows the word vector distance between countries and their respective capitals.
Subtracting “France” from “Paris” removes the country semantics, leaving a vector representing the concept of a capital city. Adding “Germany” to this vector, we are left with something closely resembling “Berlin,” the capital of Germany. The vectors for this relationship are shown in the following graph.
For our use case, we use the pre-trained Cohere Embeddings model in Amazon Bedrock, which embeds entire texts rather than a single word. The embeddings represent the meaning of the text and can be operated on using mathematical operations. This property can be useful to map relationships such as similarity between texts.
Zero-shot classification
One way in which we use language embeddings is by using their properties to calculate how similar an article is to one of the topics.
To do this, we break down a topic into a series of different and related embeddings. For instance, for culture, we have a set of embeddings for sports, TV programs, music, books, and so on. We then embed the incoming title and description of the RSS articles, and calculate the similarity against the topic embeddings. From this, we can assign topic labels to an article.
The following figure illustrates how this works. The embeddings that Cohere generates are highly dimensional, containing 1,024 values (or dimensions). However, to demonstrate how this system works, we use an algorithm designed to reduce the dimensionality of the embeddings, t-distributed Stochastic Neighbor Embedding (t-SNE), so that we can view them in two dimensions. The following image uses these embeddings to visualize how topics are clustered based on similarity and meaning.
You can use the embedding of an article and check the similarity of the article against the preceding embeddings. You can then say that if an article is clustered closely to one of these embeddings, it can be classified with the associated topic.
This is the k-nearest neighbor (k-NN) algorithm. This algorithm is used to perform classification and regression tasks. In k-NN, you can make assumptions around a data point based on its proximity to other data points. For instance, you can say that an article that has proximity to the music topic shown in the preceding diagram can be tagged with the culture topic.
The following figure demonstrates this with an ArsTechnica article. We plot against the embedding of an article’s title and description: (The climate is changing so fast that we haven’t seen how bad extreme weather could get: Decades-old statistics no longer represent what is possible in the present day).
The advantage of this approach is that you can add custom, user-generated topics. You can create a topic by first creating a series of embeddings of conceptually related items. For instance, an AI topic would be similar to the embeddings for AI, Generative AI, LLM, and Anthropic, as shown in the following screenshot.
In a traditional classification system, we’d be required to train a classifier—a supervised learning task where we’d need to provide a series of examples to establish whether an article belongs to its respective topic. Doing so can be quite an intensive task, requiring labeled data and training the model. For our use case, we can provide examples, create a cluster, and tag articles without having to provide labeled examples or train additional models. This is shown in the following screenshot of results page of our website.
In our application, we ingest new articles on a schedule. We use EventBridge schedules to periodically call a Lambda function, which checks if there are new articles. If there are, it creates an embedding from them using Amazon Bedrock and Cohere.
We calculate the article’s distance to the different topic embeddings, and can then determine whether the article belongs to that category. This is done with Aurora PostgreSQL with pgvector. We store the embeddings of the topics and then calculate their distance using the following SQL query:
The <-> operator in the preceding code calculates the Euclidean distance between the article and the topic embedding. This number allows us to understand how close an article is to one of the topics. We can then determine the appropriateness of a topic based on this ranking.
We then tag the article with the topic. We do this so that the subsequent request for a topic is as computationally as light as possible; we do a simple join rather than calculating the Euclidean distance.
We also cache a specific topic/feed combination because these are calculated hourly and aren’t expected to change in the interim.
Semantic search
As previously discussed, the embeddings produced by Cohere contain a multitude of features; they embed the meanings and semantics of a word of phrase. We’ve also found that we can perform mathematical operations on these embeddings to do things such as calculate the similarity between two phrases or words.
We can use these embeddings and calculate the similarity between a search term and an embedding of an article with the k-NN algorithm to find articles that have similar semantics and meanings to the search term we’ve provided.
For example, in one of our RSS feeds, we have a lot of different articles that rate products. In a traditional search system, we’d rely on keyword matches to provide relevant results. Although it might be simple to find a specific article (for example, by searching “best digital notebooks”), we would need a different method to capture multiple product list articles.
In a semantic search system, we first transform the term “Product list” in an embedding. We can then use the properties of this embedding to perform a search within our embedding space. Using the k-NN algorithm, we can find articles that are semantically similar. As shown in the following screenshot, despite not containing the text “Product list” in either the title or description, we’ve been able to find articles that contain a product list. This is because we were able to capture the semantics of the query and match it to the existing embeddings we have for each article.
In our application, we store these embeddings using pgvector on Aurora PostgreSQL. pgvector is an open source extension that enables vector similarity search in PostgreSQL. We transform our search term into an embedding using Amazon Bedrock and Cohere v3 Embed.
After we’ve converted the search term to an embedding, we can compare it with the embeddings on the article that have been saved during the ingestion process. We can then use pgvector to find articles that are clustered together. The SQL code for that is as follows:
This code calculates the distance between the topics, and the embedding of this article as “similarity.” If this distance is close, then we can assume that the topic of the article is related, and we therefore attach the topic to the article.
Prerequisites
To deploy this application in your own account, you need the following prerequisites:
- An active AWS account. Model access for Cohere Embed English. On the Amazon Bedrock console, choose Model access in the navigation pane, then choose Manage model access. Select the FMs of your choice and request access.
- The AWS Cloud Development Kit (AWS CDK) set up. For installation instructions, refer to Getting started with the AWS CDK. A virtual private cloud (VPC) set up with access to private VPCs. For more information, refer to Create a VPC.
Deploy the AWS CDK stack
When the prerequisite steps are complete, you’re ready to set up the solution:
- Clone the GitHub repository containing the solution files:
git clone https://github.com/aws-samples/rss-aggregator-using-cohere-embeddings-bedrock
- Navigate to the solution directory:
cd infrastructure
- In your terminal, export your AWS credentials for a role or user in ACCOUNT_ID. The role needs to have all necessary permissions for AWS CDK deployment:
- export AWS_REGION=”<region>”
– The AWS Region you want to deploy the application to export AWS_ACCESS_KEY_ID=”<access-key>”
– The access key of your role or user export AWS_SECRET_ACCESS_KEY=”<secret-key>”
– The secret key of your role or user
- If you’re deploying the AWS CDK for the first time, run the following command:
cdk bootstrap
- To synthesize the AWS CloudFormation template, run the following command:
cdk synth -c vpc_id=<ID Of your VPC>
- To deploy, use the following command:
cdk deploy -c vpc_id=<ID Of your VPC>
When deployment is finished, you can check these deployed stacks by visiting the AWS CloudFormation console, as shown in the following screenshot.
Clean up
Run the following command in the terminal to delete the CloudFormation stack provisioned using the AWS CDK:
cdk destroy --all
Conclusion
In this post, we explored what language embeddings are and how they can be used to enhance your application. We’ve learned how, by using the properties of embeddings, we can implement a real-time zero-shot classifier and can add powerful features such as semantic search.
The code for this application can be found on the accompanying GitHub repo. We encourage you to experiment with language embeddings and find out what powerful features they can enable for your applications!
About the Author
Thomas Rogers is a Solutions Architect based in Amsterdam, the Netherlands. He has a background in software engineering. At AWS, Thomas helps customers build cloud solutions, focusing on modernization, data, and integrations.