Retrieval Augmented Generation (RAG) is a state-of-the-art approach to building question answering systems that combines the strengths of retrieval and generative language models. RAG models retrieve relevant information from a large corpus of text and then use a generative language model to synthesize an answer based on the retrieved information.
The complexity of developing and deploying an end-to-end RAG solution involves several components, including a knowledge base, retrieval system, and generative language model. Building and deploying these components can be complex and error-prone, especially when dealing with large-scale data and models.
This post demonstrates how to seamlessly automate the deployment of an end-to-end RAG solution using Knowledge Bases for Amazon Bedrock and the AWS Cloud Development Kit (AWS CDK), enabling organizations to quickly set up a powerful question answering system.
Solution overview
The solution provides an automated end-to-end deployment of a RAG workflow using Knowledge Bases for Amazon Bedrock. By using the AWS CDK, the solution sets up the necessary resources, including an AWS Identity and Access Management (IAM) role, Amazon OpenSearch Serverless collection and index, and knowledge base with its associated data source.
The RAG workflow enables you to use your document data stored in an Amazon Simple Storage Service (Amazon S3) bucket and integrate it with the powerful natural language processing (NLP) capabilities of foundation models (FMs) provided by Amazon Bedrock. The solution simplifies the setup process by allowing you to programmatically modify the infrastructure, deploy the model, and start querying your data using the selected FM.
Prerequisites
To implement the solution provided in this post, you should have the following:
- An active AWS account and familiarity with FMs, Amazon Bedrock, and Amazon OpenSearch Service. Model access enabled for the required models that you intend to experiment with. The AWS CDK already set up. For installation instructions, refer to the AWS CDK workshop. An S3 bucket set up with your documents in a supported format (.txt, .md, .html, .doc/docx, .csv, .xls/.xlsx, .pdf). The Amazon Titan Embeddings V2 model enabled in Amazon Bedrock. You can confirm it’s enabled on the Model Access page of the Amazon Bedrock console. If the Amazon Titan Embeddings V2 model is enabled, the access status will show as Access granted, as shown in the following screenshot.
Set up the solution
When the prerequisite steps are complete, you’re ready to set up the solution:
- Clone the GitHub repository containing the solution files:
The activation of the virtual environment differs based on the operating system; refer to the AWS CDK workshop for activating in other environments.
- After the virtual environment is activated, you can install the required dependencies:
You can now prepare the code .zip file and synthesize the AWS CloudFormation template for this code.
- In your terminal, export your AWS credentials for a role or user in
ACCOUNT_ID
. The role needs to have all necessary permissions for CDK deployment:export AWS_REGION=”<region>” # Same region as
ACCOUNT_REGION
aboveexport AWS_ACCESS_KEY_ID=”<access-key>” # Set to the access key of your role/user
export AWS_SECRET_ACCESS_KEY=”<secret-key>” # Set to the secret key of your role/user Create the dependency: If you’re deploying the AWS CDK for the first time, run the following command: To synthesize the CloudFormation template, run the following command: Because this deployment contains multiple stacks, you have to deploy them in a specific sequence. Deploy the stacks in the following order: Once deployment is finished, you can see these deployed stacks by visiting AWS CloudFormation console as shown below. Also you can note knowledge base details (i.e. name, id) under resources tab.
Test the solution
Now that you have deployed the solution using the AWS CDK, you can test it with the following steps:
- On the Amazon Bedrock console, choose Knowledge bases in the navigation page. Select the knowledge base you created. Choose Sync to initiate the data ingestion job.
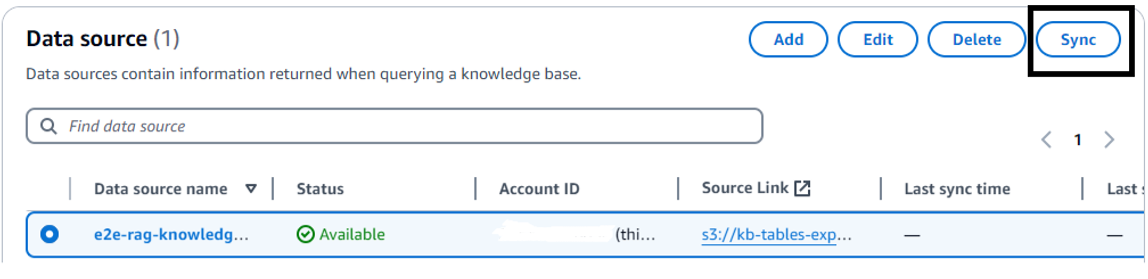
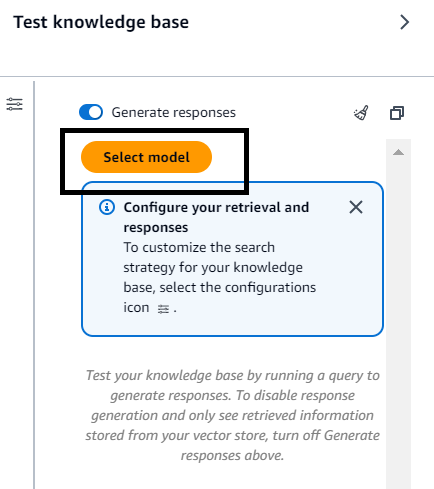
That’s it! You can now interact with your documents using the RAG workflow powered by Amazon Bedrock.
Clean up
To avoid incurring future charges on the AWS account, complete the following steps:
- Delete all files within the provisioned S3 bucket. Run the following command in the terminal to delete the CloudFormation stack provisioned using the AWS CDK:
Conclusion
In this post, we demonstrated how to quickly deploy an end-to-end RAG solution using Knowledge Bases for Amazon Bedrock and the AWS CDK.
This solution streamlines the process of setting up the necessary infrastructure, including an IAM role, OpenSearch Serverless collection and index, and knowledge base with an associated data source. The automated deployment process enabled by the AWS CDK minimizes the complexities and potential errors associated with manually configuring and deploying the various components required for a RAG solution. By taking advantage of the power of FMs provided by Amazon Bedrock, you can seamlessly integrate your document data with advanced NLP capabilities, enabling you to efficiently retrieve relevant information and generate high-quality answers to natural language queries.
This solution not only simplifies the deployment process, but also provides a scalable and efficient way to use the capabilities of RAG for question-answering systems. With the ability to programmatically modify the infrastructure, you can quickly adapt the solution to help meet your organization’s specific needs, making it a valuable tool for a wide range of applications that require accurate and contextual information retrieval and generation.
About the Authors
Sandeep Singh is a Senior Generative AI Data Scientist at Amazon Web Services, helping businesses innovate with generative AI. He specializes in generative AI, machine learning, and system design. He has successfully delivered state-of-the-art AI/ML-powered solutions to solve complex business problems for diverse industries, optimizing efficiency and scalability.
Manoj Krishna Mohan is a Machine Learning Engineering at Amazon. He specializes in building AI/ML solutions using Amazon SageMaker. He is passionate about developing ready-to-use solutions for the customers. Manoj holds a master’s degree in Computer Science specialized in Data Science from the University of North Carolina, Charlotte.
Mani Khanuja is a Tech Lead – Generative AI Specialists, author of the book Applied Machine Learning and High-Performance Computing on AWS, and a member of the Board of Directors for Women in Manufacturing Education Foundation Board. She leads machine learning projects in various domains such as computer vision, natural language processing, and generative AI. She speaks at internal and external conferences such AWS re:Invent, Women in Manufacturing West, YouTube webinars, and GHC 23. In her free time, she likes to go for long runs along the beach.