Try Fully Managed Apache Airflow and get certified for FREE (Sponsored)
Run Airflow without the hassle and management complexity. Take Astro (the fully managed Airflow solution) for a test drive today and unlock a suite of features designed to simplify, optimize, and scale your data pipelines. For a limited time, new sign ups will receive a complimentary Airflow Fundamentals Certification exam (normally $150).
Disclaimer: The details in this post have been derived from the Uber Engineering Blog. All credit for the technical details goes to the Uber engineering team. The links to the original articles are present in the references section at the end of the post. We’ve attempted to analyze the details and provide our input about them. If you find any inaccuracies or omissions, please leave a comment, and we will do our best to fix them.
Ledgers are the source of truth of any financial event. By their very nature, ledgers are immutable. Also, we usually want to access data stored in these ledgers in various combinations.
With billions of trips and deliveries, Uber performs tens of billions of financial transactions. Merchants, riders, and customers are involved in these financial transactions. Money flows from the ones spending to the ones earning.
To manage this mountain of financial transaction data, the LedgerStore is an extremely critical storage solution for Uber. The myriad access patterns for the data stored in LedgerStore also create the need for a huge number of indexes.
In this post, we’ll look at how Uber implemented the indexing architecture for LedgerStore to handle trillions of indexes and how they migrated a trillion entries of Uber’s Ledger Data from DynamoDB to LedgerStore.
What is LedgerStore?
LedgerStore is Uber’s custom-built storage solution for managing financial transactions. Think of it as a giant, super-secure digital ledger book that keeps track of every financial event at Uber, from ride payments to food delivery charges.
What makes LedgerStore special is its ability to handle an enormous amount of data. We’re talking about trillions of entries.
Two main features supported by LedgerStore are:
Immutability: LedgerStore is designed to be immutable, which means once a record is written, it cannot be changed. This ensures the integrity of financial data.
Indexes: LedgerStore allows quick look-up of information using various types of indexes. For example, if someone needs to check all transactions for a particular user or all payments made on a specific date, LedgerStore can retrieve this information efficiently.
Ultimately, LedgerStore helps Uber manage its financial data more effectively, reducing costs compared to previous solutions.
Types of Indexes
LedgerStore supports three main types of indexes:
Strongly consistent indexes
Eventually consistent indexes
Time-range indexes
Let’s look at each of them in more detail.
Strongly Consistent Indexes
These indexes provide immediate read-your-write guarantees, crucial for scenarios like credit card authorization flows. For example, when a rider starts an Uber trip, a credit card hold is placed, which must be immediately visible to prevent duplicate charges.
See the diagram below that shows the credit-card payment flow for an Uber trip supported by strongly consistent indexes.
If the index is not strongly consistent, the hold may take a while to be visible upon reading. This can result in duplicate charges on the user’s credit card.
Strongly consistent indexes at Uber are built using the two-phase commit approach. Here’s how this approach works in the write path and read path.
1 - The Write Path
The write path consists of the following steps:
When a new record needs to be inserted, the system first writes an “index intent” to the index table. It could even be multiple indexes.
This intent signifies that a new record is about to be written. If the index intent write fails, the whole insert operation fails.
After the index intent is successfully written, the actual record is written to the main data store.
If the record write is also successful, the system commits the index. This is done asynchronously to avoid affecting the end-user insert latency.
The diagram below shows this entire process.
There is one special case to consider here: if the index intent write succeeds, but the record write fails, the index intent has to be rolled back to prevent the accumulation of unused intents. This part is handled during the read path.
2 - The Read Path
The below steps happen during the read path:
If a committed index entry is found, the response data is returned to the client.
If an index entry is found but with a “pending” status, the system must resolve its state. This is done by checking the main data store for the corresponding record.
If the record exists, the index is asynchronously committed and the record is returned to the user.
If the record doesn’t exist, the index intent is deleted or rolled back and the read operation does not return a result for the query.
The diagram below shows the process steps in more detail.
Reserve Your Seat Now! | Upcoming Cohort on Aug 26th, 2024 (Sponsored)
Build confidence without getting lost in technical jargon.
This cohort is designed to help you build a foundational understanding of software applications. You won’t just memorize a bunch of terms - you’ll actually understand how software products are designed and deployed to handle millions of users.
And our community will be here to give you the support, guidance, and accountability you’ll need to finally stick with it.
After only 4 weeks, you’ll look back and think.. “WOW! I can’t believe I did that.”
Now imagine if you could:
✅ Master technical concepts without getting lost in an internet maze.
✅ Stop asking engineers to dumb down concepts when talking to you.
✅ Predict risks, anticipate issues, and avoid endless back-and-forth.
✅ Improve your communication with engineers, users, and technical stakeholders.
Grab your spot now with an exclusive 25% off discount for ByteByteGo Readers. See you there!
Eventually Consistent Indexes
These indexes are designed for scenarios where real-time consistency is not critical, and a slight delay in index updates is acceptable. They offer better performance and availability at the cost of immediate consistency.
From a technical implementation point of view, the eventually consistent indexes are generated using the Materialized Views feature of Uber’s Docstore.
Materialized views are pre-computed result sets stored as a table, based on a query against the base table(s). The materialized view is updated asynchronously when the base table changes.
When a write occurs to the main data store, it doesn’t immediately update the index. Instead, a separate process periodically scans for changes and updates the materialized view. The consistency window is configurable and determines how frequently the background process runs to update the indexes.
In Uber’s case, the Payment History Display screen uses the eventually consistent indexes.
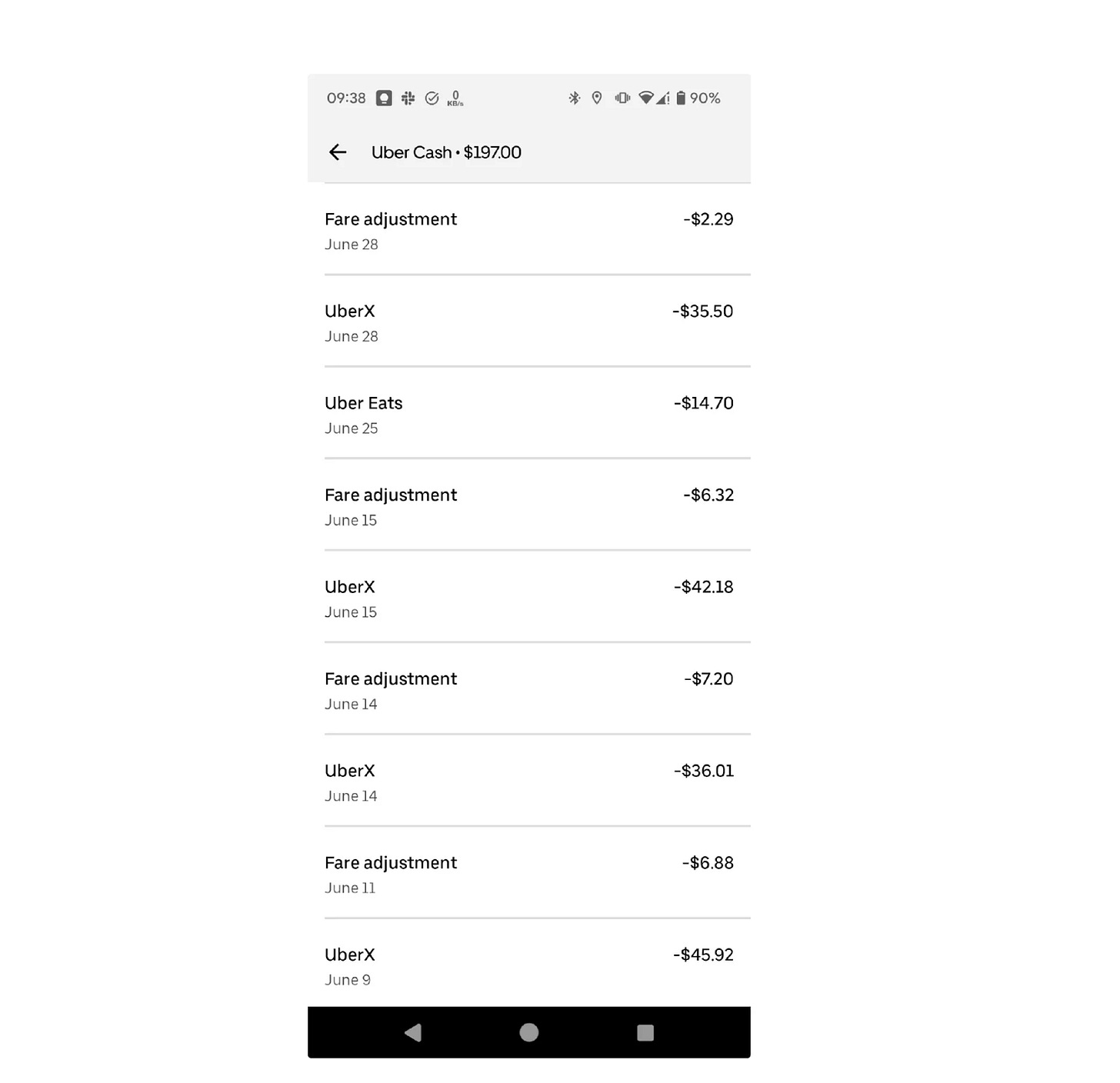
Time-range Indexes
Time-range indexes are a crucial component of LedgerStore, designed to query data within specific time ranges efficiently.
These indexes are important for operations like offloading older ledger data to cold storage or sealing data for compliance purposes. The main characteristic of these indexes is their ability to handle deterministic time-range batch queries that are significantly larger in scope compared to other index types.
Earlier, the time-range indexes were implemented using a dual-table design approach in DynamoDB. However, it was operationally complex.
The migration of LedgerStore to Uber’s Docstore paved the path for a simpler implementation of the time-range index. Here’s a detailed look at the Docstore implementation for the time-range indexes:
Single Table Design: Only one table is used for time-range indexes in Docstore.
Partitioning Strategy: Index entries are partitioned based on full timestamp value. This ensures a uniform distribution of writes across partitions, eliminating the chances of hot partitions and write throttling.
Sorted Data Storage: Data is stored in a sorted manner based on the primary key (partition + sort keys).
Read Operation: Reads involve a prefix scanning of each shard of the table up to a certain time granularity. For example, to read 30 minutes of data, the operation might be broken down into three 10-minute interval scans. Each scan is bounded by start and end timestamps. After scanning, a scatter-gather operation is performed, followed by sort merging across shards to obtain all time-range index entries in the given window, in a sorted fashion.
For clarity, consider a query to fetch all ledger entries between “2024-08-09 10:00:00” and “2024-08-09 10:30:00”. The query would be broken down into three 10-minute scans:
2024-08-09 10:00:00 to 2024-08-09 10:10:00
2024-08-09 10:10:00 to 2024-08-09 10:20:00
2024-08-09 10:20:00 to 2024-08-09 10:30:00
Each of these scans would be executed across all shards in parallel. The results would then be merged and sorted to provide the final result set.
The diagram below shows the overall process:
Index Lifecycle Management
Index lifecycle management is another component of LedgerStore’s architecture that handles the design, modification, and decommissioning of indexes.
Let’s look at the main parts of the index lifecycle management system.
Index Lifecycle State Machine
This component orchestrates the entire lifecycle of an index:
Creating the index table
Backfilling it with historical index entries
Validating the entries for completeness
Swapping the old index with the new one for read/write operations
Decommissioning the old index
The state machine ensures that each step is completed correctly before moving to the next, maintaining data integrity throughout the process.
The diagram below shows all the steps:
Historical Index Data Backfill
When new indexes are defined or existing ones are modified, it’s essential to backfill historical data to ensure completeness.
The historical index data backfill component performs the following tasks:
Builds indexes from historical data stored in cold storage.
Backfills the data to the storage layer in a scalable manner.
Uses configurable rate-limiting and batching to manage the process efficiently.
Index Validation
After indexes are backfilled, they need to be verified for completeness. This is done through an offline job that:
Computes order-independent checksums at a certain time-window granularity.
Compares these checksums across the source of truth data and the index table.
From a technical point of view, the component uses a time-window approach i.e. computing checksums for every 1-hour block of data. Even if a single entry is missed, the aggregate checksum for that time window will lead to a mismatch.
For example, If checksums are computed for 1-hour blocks, and an entry from 2:30 PM is missing, the checksum for the 2:00 PM - 3:00 PM block will not match.
Migration of Uber’s Payment Data to LedgerStore
Now that we have understood about LedgerStore’s indexing architecture and capabilities, let’s look at the massive migration of Uber’s payment data to LedgerStore.
Uber’s payment platform, Gulfstream, was initially launched in 2017 using Amazon DynamoDB for storage. However, as Uber’s operations grew, this setup became increasingly expensive and complex.
By 2021, Gulfstream was using a combination of three storage systems:
DynamoDB for the most recent 12 weeks of data. This was the hot data.
TerraBlob (Uber’s internal blob store like AWS S3) for older or cold data.
LedgerStore (LSG) where new data was being written and where they wanted to migrate all data.
The primary reasons for migrating to LedgerStore were as follows:
Cost savings: Moving to LedgerStore promised significant recurring cost savings compared to DynamoDB.
Simplification: Consolidating from three storage systems to one would simplify the code and design of the Gulfstream services.
Improved Performance: LedgerStore offered shorter indexing lag and reduced latency due to being on-premise.
Purpose-Built Design: LedgerStore was specifically designed for storing payment-style data, offering features like verifiable immutability and tiered storage for cost management.
The migration was a massive undertaking. Some statistics are as follows:
1.2 Petabytes of compressed data
Over 1 trillion entries
0.5 PB of uncompressed data for secondary indexes.
For reference, storing this data on typical 1 TB hard drives requires a total of 1200 hard drives just for the compressed data.
Checks
One of the key goals of the migration was to ensure that the backfill was correct and acceptable. Also, the current traffic requirements needed to be fulfilled.
Key validation methods adopted were as follows:
1 - Shadow Validation
This ensured that the new LedgerStore system could handle current traffic patterns without disruption.
Here’s how it worked:
The system would compare responses from the existing DynamoDB-based system with what the LedgerStore would return for the same queries. This allowed the team to catch any discrepancies in real time.
An ambitious goal was to ensure 99.99% completeness and correctness with an upper bound of 99.9999%.
To achieve six nines of confidence, the team needed to compare at least 100 million records. At a rate of 1000 comparisons per second, this would take more than a day to collect sufficient data.
During shadow validation, production traffic was duplicated on LedgerStore. This helped the team verify the LedgerStore’s ability to handle the production load.
2 - Offline Validation
While shadow validation was effective for current traffic patterns, it couldn’t provide strong guarantees about rarely-accessed historical data. This is where offline validation came into play.
Here’s how it worked:
Offline validation involved comparing complete data dumps from DynamoDB with the data in LedgerStore. The largest offline validation job compared 760 billion records, involving 70 TB of compressed data.
The team used Apache Spark for these massive comparison jobs, leveraging distributed shuffle-as-a-service for Spark.
Backfill Issues
The process of migrating Uber’s massive ledger data from DynamoDB to LedgerStore involved several backfill challenges that had to be solved:
Scalability: The engineering team learned that starting small and gradually increasing the scale was crucial. Blindly pushing beyond the system’s limit could create a DDoS attack on their systems.
Incremental Backfills: Given the enormous volume of data, attempting to backfill all at once would generate 10 times the normal traffic load. The solution was to break the backfill into smaller, manageable batches that could be completed within minutes.
Rate Control: To ensure consistent behavior during backfill, the team implemented rate control using Guava’s RateLimiter in Java/Scala. The team also developed a system to dynamically adjust the backfill rate based on the current system load. For this, they used an additive increase/multiplicative decrease approach similar to TCP congestion control.
Data File Size Management: The team found that managing the size of data files was important. They aimed to keep the file sizes around 1 GB, with flexibility between 100 MB and 10 GB. This approach helped avoid issues like MultiPart limitations in various tools and prevented problems associated with having too many small files.
Fault Tolerance: Data quality issues and data corruption were inevitable. The team’s solution was to monitor statistics. If the failure rate was high, they would manually stop the backfill, fix the issue, and continue. For less frequent issues, they let the backfill continue while addressing problems in parallel.
Logging Challenges: Excessive logging during backfill could overload the logging infrastructure. The solution was to use a rate limiter for logging. For example, they might log only once every 30 seconds for routine operations while logging all errors if they occurred infrequently.
Conclusion
The impact of Uber’s ledger data migration to LedgerStore has been amazing, with over 2 trillion unique indexes successfully transferred without a single data inconsistency detected in over six months of production use.
This migration, involving 1.2 PB of compressed data and over 1 trillion entries, showcases Uber’s ability to handle massive-scale data operations without disrupting critical financial processes. It also provides great learning points for the readers.
The cost savings from this migration have been substantial, with estimated yearly savings exceeding $6 million due to reduced spend on DynamoDB. Performance improvements have been notable, with LedgerStore offering shorter indexing lag and better network latency due to its on-premise deployment within Uber’s data centers.
References: