Hands-on Rust Developer Workshop: Build a Low-Latency Social Media App (Sponsored)
During this free interactive workshop oriented for developers, engineers, and architects, you will learn how to:
Create and compile a sample social media app with Rust
Connect the application to ScyllaDB (NoSQL data store) and Redpanda (streaming data)
Negotiate tradeoffs related to data modeling and querying
Manage and monitor the database for consistently low latencies
If you’re an application developer with an interest in Rust, Tokio, and event-driven architectures this workshop is for you! This is a great way to discover the NoSQL strategies used by top teams and apply them in a guided, supportive environment.
Disclaimer: The details in this post have been derived from the Canva Engineering Blog. All credit for the technical details goes to the Canva engineering team. The links to the original articles are present in the references section at the end of the post. We’ve attempted to analyze the details and provide our input about them. If you find any inaccuracies or omissions, please leave a comment, and we will do our best to fix them.
What if incorrect counting results in incorrect payments?
Either the company making the payment or the users receiving that payment based on the incorrect count lose money. Both scenarios are problematic from the business perspective.
This is exactly the situation that Canva faced when they launched the Creators Program.
As you might already know, Canva is a tool that makes design accessible to everyone worldwide. One of the main ways they make this possible is through the Canva Creators Program.
In the three years since its launch, the use of content from this program has doubled every 18 months. They process billions of content uses monthly based on which the creators are paid. This includes the use of templates, images, videos, and more.
It is a critical requirement for Canva to count the usage data of this content accurately since the payments made to the creators depend on this data. However, it also presents some big challenges:
Accuracy: The count has to be correct to maintain creator trust and ensure fair pay.
Scalability: The system needs to handle rapidly growing amounts of data
Operability: As data increases, so does the complexity of maintenance and problem-solving.
In this post, we will look at the various architectures Canva’s engineering team experimented with to implement a robust counting service and the lessons they learned in the process.
The Initial Counting Service Design
Canva's original design for the content usage counting service was built on a MySQL database, a familiar and widely-used technology stack.
This initial design comprised several key components:
A MySQL database for storing all usage data (including raw events, deduplicated usage, and aggregated counts).
Separate worker services handling different pipeline stages (data collection, deduplication, and aggregation).
The persistence of multiple layers of reusable intermediary output at various stages.
The process flow for the solution could be broken down into three main steps:
Data Collection: Usage events from various sources (web, and mobile apps) were collected and stored in MySQL.
Deduplication: A worker service identifies duplicated usage events and matches them with a specific set of rules.
Aggregation: Another worker scanned the updated deduplication table, incrementing counters in a separate table.
The diagram below shows the architecture on a high level.
This architecture employed a single-threaded sequential process for deduplication, using a pointer to track the latest scanned record. While this approach made it easier to reason about and verify data processing, especially during troubleshooting or incident recovery, it faced significant scalability challenges.
The system required at least one database round trip per usage record, resulting in O(N) database queries for N records, which became increasingly problematic as data volume grew.
The initial MySQL-based architecture prioritized simplicity and familiarity over scalability. It allowed for quick implementation but created substantial challenges as the system expanded.
The heavy reliance on MySQL for both storage and processing created a bottleneck, failing to leverage the strengths of distributed systems or parallel processing.
MySQL RDS does not horizontally scale through partitioning by itself. Every time they needed more storage, they doubled the RDS instance size. This happened every 8-10 months, resulting in significant operational overhead.
Once the MySQL RDS instance reached several TBs, maintaining it became costly.
Lastly, finding and fixing issues in case of incidents was difficult because engineers had to look into databases and manually fix the incorrect data.
Latest articles
If you’re not a paid subscriber, here’s what you missed.
A Crash Course on Microservices Design Patterns
A Crash Course on Domain-Driven Design
To receive all the full articles and support ByteByteGo, consider subscribing:
Migration to DynamoDB
Faced with the scalability limitations of the MySQL-based counting service, the team initially looked to DynamoDB as a potential solution.
This decision was primarily driven by DynamoDB's reputation for handling large-scale, high-throughput workloads - a perfect fit for Canva's rapidly growing data needs.
The migration process began with moving raw usage events from the data collection stage to DynamoDB, which provided immediate relief to the storage constraints. This initial success prompted the team to consider moving the entirety of their data to DynamoDB. It was a move that would have necessitated a substantial rewrite of their codebase.
However, after careful evaluation, Canva decided against a full migration to DynamoDB.
While DynamoDB could have effectively addressed the storage scalability issues, it wouldn't have solved the fundamental problem of processing scalability. The team found it challenging to eliminate the need for frequent database round trips, which was a key bottleneck in their existing system.
This reveals a crucial lesson in system design: sometimes, what appears to be a storage problem is a processing problem in disguise.
Canva's approach clearly shows the importance of thoroughly analyzing the root causes of system limitations before committing to major architectural changes. It also highlights the complexity of scaling data-intensive applications, where the interplay between storage and processing capabilities can be subtle and non-obvious.
The OLAP-based Counting Service
Canva's latest architecture for the content usage counting service shows a shift from traditional OLTP databases to an OLAP-based solution, specifically using Snowflake.
The change came after realizing that previous attempts with MySQL and DynamoDB couldn't adequately address their scalability and processing needs. The new architecture altered how Canva processed and stored the usage data, adopting an ELT (Extract, Load, Transform) approach.
The diagram below shows the new architecture:
In the extraction phase, Canva pulled raw usage data from various sources, including web browsers and mobile apps. This data was then loaded into Snowflake using a reliable data replication pipeline provided by Canva’s data platform team. The reliability of this data replication was crucial, as it formed the foundation for all subsequent processing.
The transformation phase used Snowflake's powerful computational capabilities. It also utilized DBT (Data Build Tool) to define complex transformations.
These transformations were written as SQL-like queries, allowing for end-to-end calculations directly on the source data. For example, one transformation aggregated usages per brand using a SQL query that selected data from a previous step named 'daily_template_usages' and grouped it by ‘day_id’ and ‘template_brand’.
The SQL below shows the aggregate query.
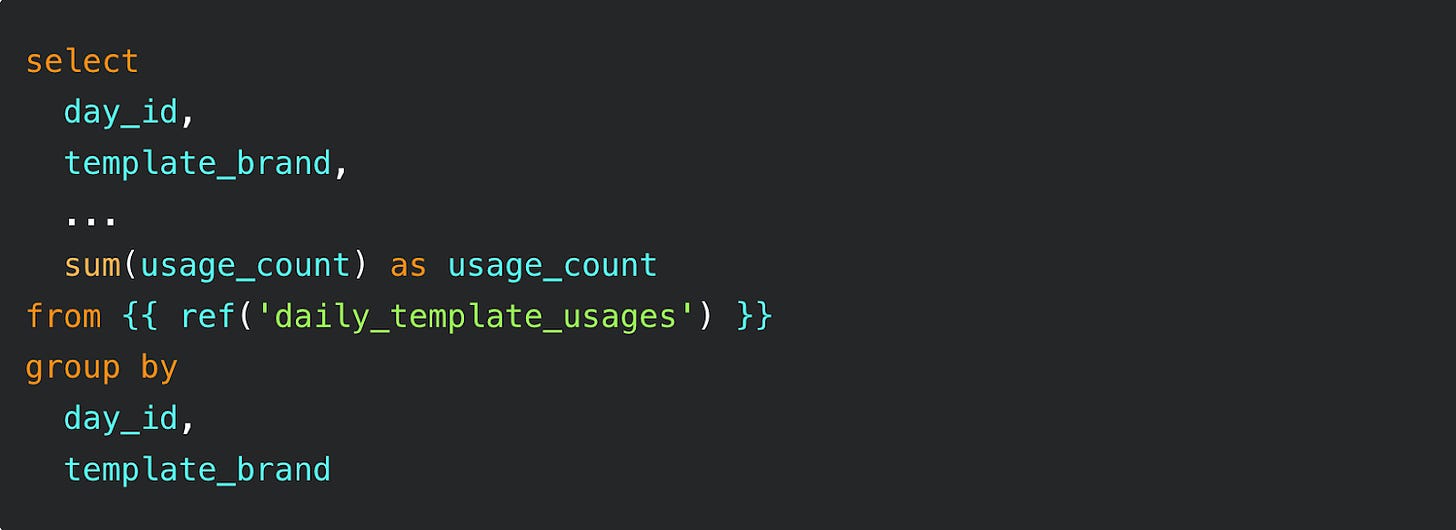
The main steps in the transformation process were as follows:
Extraction of source event data in JSON format from DynamoDB, which was not optimal for data warehouse query processing. Therefore, some JSON properties were projected into separate table columns within Snowflake for optimization.
Deduplicate and aggregate the usage events.
A key aspect of this new architecture was the elimination of intermediary outputs. Instead of persisting data at various pipeline stages, Canva materialized intermediate transformation outputs as SQL Views.
The Advantage of OLAP Database
The separation of storage and computing in an OLAP database like Snowflake was a game-changer for Canva. It enabled them to scale computational resources independently.
As a result, they could now aggregate billions of usage records within minutes, a task that previously took over a day. This improvement was largely due to most of the computation being done in memory, which is several orders of magnitude faster than the database round trips required in their previous architecture.
There were several improvements such as:
The pipeline latency was reduced from over a day to under an hour.
Incident handling became more manageable. Most issues could be resolved by simply re-running the pipeline end-to-end, without manual database interventions.
Reduction in over 50% of the stored data and elimination of thousands of lines of deduplication and aggregation calculation code. The logic rewritten in SQL was simpler compared to the previous code.
The number of incidents dropped to once every few months or fewer.
Challenges of the New Solution
Despite the advantages, the solution also introduced new challenges such as:
Transformation Complexity: The data transformation jobs, written in an SQL-like language and deployed as a standalone service, introduced new deployment and compatibility considerations.
Data Unloading: Canva needed to build a reliable pipeline to unload data from Snowflake into databases used by other services. This involved using S3 as intermediary storage and integrating with SQS for durability. Optimizing the ingestion query and carefully tuning rate limits was crucial to prevent service database throttling.
Infrastructure Complexity: The new architecture increased infrastructure complexity due to data replication integration and standalone services running transformation jobs. This required additional effort to maintain observability across different toolsets.
Conclusion
Canva’s journey of implementing the counting service for the Creators Program is full of learning for software developers and architects.
Some of the key points to take away are as follows:
Simplicity is crucial for designing reliable services. In other words, reducing code and data complexity is the key. For example, minimizing intermediary output in various counting pipeline stages using OLAP and ELT helped simplify the system.
It’s good to start small and be pragmatic. The initial MySQL-based design served its purpose for the first two years and allowed timely delivery of the functionality to the users. Once scalability became a problem, they looked at alternative solutions.
Comprehensive observability, while requiring extra overhead, helps identify and resolve problems. Close monitoring from day one of every part of the pipeline was crucial to make the right decisions.
Recognizing when solutions are no longer adequate and being open to change is important. In Canva’s case, this was demonstrated by the willingness to pivot from familiar MySQL to a more suitable solution using OLAP database.
Big changes happen in increments. For example, the journey from MySQL to DynamoDB and finally to an OLAP solution showed the power of iterative improvements.
Reliable infrastructure is important to build a stable platform.
References:
SPONSOR US
Get your product in front of more than 1,000,000 tech professionals.
Our newsletter puts your products and services directly in front of an audience that matters - hundreds of thousands of engineering leaders and senior engineers - who have influence over significant tech decisions and big purchases.
Space Fills Up Fast - Reserve Today
Ad spots typically sell out about 4 weeks in advance. To ensure your ad reaches this influential audience, reserve your space now by emailing sponsorship@bytebytego.com