Despite notable advancements in large language models (LLMs), effective performance on reasoning-intensive tasks—such as mathematical problem solving, algorithmic planning, or coding—remains constrained by model size, training methodology, and inference-time capabilities. Models that perform well on general NLP benchmarks often lack the ability to construct multi-step reasoning chains or reflect on intermediate problem-solving states. Furthermore, while scaling up model size can improve reasoning capacity, it introduces prohibitive computational and deployment costs, especially for applied use in education, engineering, and decision-support systems.
Microsoft Releases Phi-4 Reasoning Model Suite
Microsoft recently introduced the Phi-4 reasoning family, consisting of three models—Phi-4-reasoning, Phi-4-reasoning-plus, and Phi-4-mini-reasoning. These models are derived from the Phi-4 base (14B parameters) and are specifically trained to handle complex reasoning tasks in mathematics, scientific domains, and software-related problem solving. Each variant addresses different trade-offs between computational efficiency and output precision. Phi-4-reasoning is optimized via supervised fine-tuning, while Phi-4-reasoning-plus extends this with outcome-based reinforcement learning, particularly targeting improved performance in high-variance tasks such as competition-level mathematics.
The models were released with transparent training details and evaluation logs, including contamination-aware benchmark design, and are hosted openly on Hugging Face for reproducibility and public access.
Technical Composition and Methodological Advances
The Phi-4-reasoning models build upon the Phi-4 architecture with targeted improvements to model behavior and training regime. Key methodological decisions include:
- Structured Supervised Fine-Tuning (SFT): Over 1.4M prompts were curated with a focus on “boundary” cases—problems at the edge of Phi-4’s baseline capabilities. Prompts were sourced and filtered to emphasize multi-step reasoning rather than factual recall, and responses were synthetically generated using o3-mini in high-reasoning mode.Chain-of-Thought Format: To facilitate structured reasoning, models were trained to generate output using explicit
<think>
tags, encouraging separation between reasoning traces and final answers.Extended Context Handling: The RoPE base frequency was modified to support a 32K token context window, allowing for deeper solution traces, particularly relevant in multi-turn or long-form question formats.Reinforcement Learning (Phi-4-reasoning-plus): Using Group Relative Policy Optimization (GRPO), Phi-4-reasoning-plus was further refined on a small curated set of ∼6,400 math-focused problems. A reward function was crafted to favor correct, concise, and well-structured outputs, while penalizing verbosity, repetition, and format violations.This data-centric and format-aware training regime supports better inference-time utilization and model generalization across domains, including unseen symbolic reasoning problems.
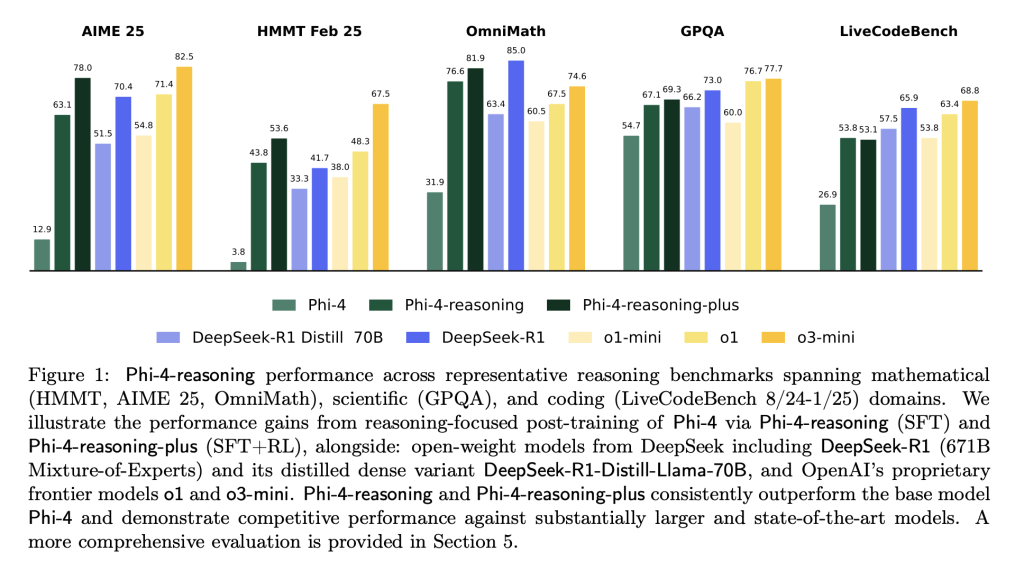
Evaluation and Comparative Performance
Across a broad range of reasoning benchmarks, Phi-4-reasoning and Phi-4-reasoning-plus deliver competitive results relative to significantly larger open-weight models:
Phi-4-reasoning-plus shows strong performance not only on domain-specific evaluations but also generalizes well to planning and combinatorial problems like TSP and 3SAT, despite no explicit training in these areas. Performance gains were also observed in instruction-following (IFEval) and long-context QA (FlenQA), suggesting the chain-of-thought formulation improves broader model utility.
Importantly, Microsoft reports full variance distributions across 50+ generation runs for sensitive datasets like AIME 2025, revealing that Phi-4-reasoning-plus matches or exceeds the performance consistency of models like o3-mini, while remaining disjoint from smaller baseline distributions like DeepSeek-R1-Distill.

Conclusion and Implications
The Phi-4 reasoning models represent a methodologically rigorous effort to advance small model capabilities in structured reasoning. By combining data-centric training, architectural tuning, and minimal but well-targeted reinforcement learning, Microsoft demonstrates that 14B-scale models can match or outperform much larger systems in tasks requiring multi-step inference and generalization.
The models’ open weight availability and transparent benchmarking set a precedent for future development in small LLMs, particularly for applied domains where interpretability, cost, and reliability are paramount. Future work is expected to extend the reasoning capabilities into additional STEM fields, improve decoding strategies, and explore scalable reinforcement learning on longer horizons.
Check out the Paper, HuggingFace Page and Microsoft Blog. Also, don’t forget to follow us on Twitter and join our Telegram Channel and LinkedIn Group. Don’t Forget to join our 90k+ ML SubReddit.
The post Microsoft AI Released Phi-4-Reasoning: A 14B Parameter Open-Weight Reasoning Model that Achieves Strong Performance on Complex Reasoning Tasks appeared first on MarkTechPost.