You have likely already had the opportunity to interact with generative artificial intelligence (AI) tools (such as virtual assistants and chatbot applications) and noticed that you don’t always get the answer you are looking for, and that achieving it may not be straightforward. Large language models (LLMs), the models behind the generative AI revolution, receive instructions on what to do, how to do it, and a set of expectations for their response by means of a natural language text called a prompt. The way prompts are crafted greatly impacts the results generated by the LLM. Poorly written prompts will often lead to hallucinations, sub-optimal results, and overall poor quality of the generated response, whereas good-quality prompts will steer the output of the LLM to the output we want.
In this post, we show how to build efficient prompts for your applications. We use the simplicity of Amazon Bedrock playgrounds and the state-of-the-art Anthropic’s Claude 3 family of models to demonstrate how you can build efficient prompts by applying simple techniques.
Prompt engineering
Prompt engineering is the process of carefully designing the prompts or instructions given to generative AI models to produce the desired outputs. Prompts act as guides that provide context and set expectations for the AI. With well-engineered prompts, developers can take advantage of LLMs to generate high-quality, relevant outputs. For instance, we use the following prompt to generate an image with the Amazon Titan Image Generation model:
An illustration of a person talking to a robot. The person looks visibly confused because he can not instruct the robot to do what he wants.
We get the following generated image.
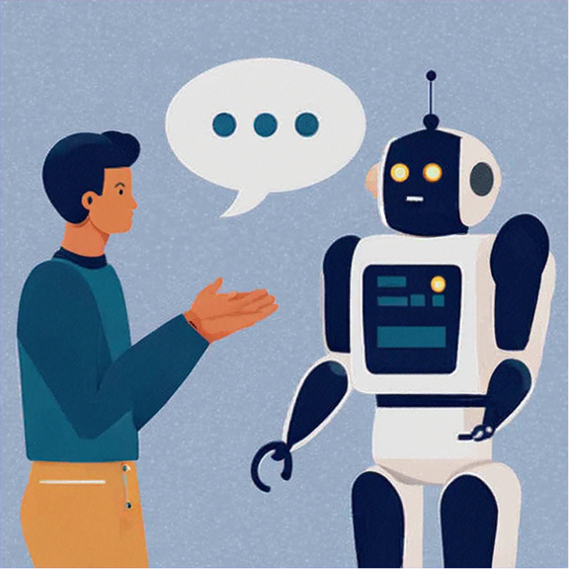
Let’s look at another example. All the examples in this post are run using Claude 3 Haiku in an Amazon Bedrock playground. Although the prompts can be run using any LLM, we discuss best practices for the Claude 3 family of models. In order to get access to the Claude 3 Haiku LLM on Amazon Bedrock, refer to Model access.
We use the following prompt:
Claude 3 Haiku’s response:
The request prompt is actually very ambiguous. 10 + 10 may have several valid answers; in this case, Claude 3 Haiku, using its internal knowledge, determined that 10 + 10 is 20. Let’s change the prompt to get a different answer for the same question:
1 + 1 is an addition1 - 1 is a substraction1 * 1 is multiplication1 / 1 is a divisionWhat is 10 + 10?
Claude 3 Haiku’s response:
10 + 10 is an addition. The answer is 20.
The response changed accordingly by specifying that 10 + 10 is an addition. Additionally, although we didn’t request it, the model also provided the result of the operation. Let’s see how, through a very simple prompting technique, we can obtain an even more succinct result:
1 + 1 is an addition1 - 1 is a substraction1 * 1 is multiplication1 / 1 is a divisionWhat is 10 + 10?Answer only as in the examples provided and provide no additional information.
Claude 3 Haiku response:
Well-designed prompts can improve user experience by making AI responses more coherent, accurate, and useful, thereby making generative AI applications more efficient and effective.
The Claude 3 model family
The Claude 3 family is a set of LLMs developed by Anthropic. These models are built upon the latest advancements in natural language processing (NLP) and machine learning (ML), allowing them to understand and generate human-like text with remarkable fluency and coherence. The family is comprised of three models: Haiku, Sonnet, and Opus.
Haiku is the fastest and most cost-effective model on the market. It is a fast, compact model for near-instant responsiveness. For the vast majority of workloads, Sonnet is two times faster than Claude 2 and Claude 2.1, with higher levels of intelligence, and it strikes the ideal balance between intelligence and speed—qualities especially critical for enterprise use cases. Opus is the most advanced, capable, state-of-the-art foundation model (FM) with deep reasoning, advanced math, and coding abilities, with top-level performance on highly complex tasks.
Among the key features of the model’s family are:
Vision capabilities – Claude 3 models have been trained to not only understand text but also images, charts, diagrams, and more. Best-in-class benchmarks – Claude 3 exceeds existing models on standardized evaluations such as math problems, programming exercises, and scientific reasoning. Specifically, Opus outperforms its peers on most of the common evaluation benchmarks for AI systems, including undergraduate level expert knowledge (MMLU), graduate level expert reasoning (GPQA), basic mathematics (GSM8K), and more. It exhibits high levels of comprehension and fluency on complex tasks, leading the frontier of general intelligence. Reduced hallucination – Claude 3 models mitigate hallucination through constitutional AI techniques that provide transparency into the model’s reasoning, as well as improved accuracy. Claude 3 Opus shows an estimated twofold gain in accuracy over Claude 2.1 on difficult open-ended questions, reducing the likelihood of faulty responses. Long context window – Claude 3 models excel at real-world retrieval tasks with a 200,000-token context window, the equivalent of 500 pages of information.
To learn more about the Claude 3 family, see Unlocking Innovation: AWS and Anthropic push the boundaries of generative AI together, Anthropic’s Claude 3 Sonnet foundation model is now available in Amazon Bedrock, and Anthropic’s Claude 3 Haiku model is now available on Amazon Bedrock.
The anatomy of a prompt
As prompts become more complex, it’s important to identify its various parts. In this section, we present the components that make up a prompt and the recommended order in which they should appear:
Task context: Assign the LLM a role or persona and broadly define the task it is expected to perform. Tone context: Set a tone for the conversation in this section. Background data (documents and images): Also known as context. Use this section to provide all the necessary information for the LLM to complete its task. Detailed task description and rules: Provide detailed rules about the LLM’s interaction with its users. Examples: Provide examples of the task resolution for the LLM to learn from them. Conversation history: Provide any past interactions between the user and the LLM, if any. Immediate task description or request: Describe the specific task to fulfill within the LLMs assigned roles and tasks. Think step-by-step: If necessary, ask the LLM to take some time to think or think step by step. Output formatting: Provide any details about the format of the output. Prefilled response: If necessary, prefill the LLMs response to make it more succinct.
The following is an example of a prompt that incorporates all the aforementioned elements:
Human: You are a solutions architect working at Amazon Web Services (AWS) named John Doe. Your goal is to answer customers' questions regarding AWS best architectural practices and principles. Customers may be confused if you don't respond in the character of John.You should maintain a friendly customer service tone.Answer the customers' questions using the information provided below<context>{{CONTEXT}}</context>Here are some important rules for the interaction:- Always stay in character, as John, a solutions architect that work at AWS. - If you are unsure how to respond, say "Sorry, I didn't understand that. Could you repeat the question?"- If someone asks something irrelevant, say, "Sorry, I am John and I give AWS architectural advise. Do you have an AWS architecture question today I can help you with?" Here is an example of how to respond in a standard interaction: <example> User: Hi, what do you do? John: Hello! My name is John, and I can answer your questions about best architectural practices on AWS. What can I help you with today? </example>Here is the conversation history (between the user and you) prior to the question. It could be empty if there is no history: <history>{{HISTORY}}</history>Here is the user's question: <question>{{QUESTION}}</question> How do you respond to the user's question?Think about your answer first before you respond.Put your response in <response></responses>Assistant: <response>
Best prompting practices with Claude 3
In the following sections, we dive deep into Claude 3 best practices for prompt engineering.
Text-only prompts
For prompts that deal only with text, follow this set of best practices to achieve better results:
Mark parts of the prompt with XLM tags – Claude has been fine-tuned to pay special attention to XML tags. You can take advantage of this characteristic to clearly separate sections of the prompt (instructions, context, examples, and so on). You can use any names you prefer for these tags; the main idea is to delineate in a clear way the content of your prompt. Make sure you include <> and </> for the tags. Always provide good task descriptions – Claude responds well to clear, direct, and detailed instructions. When you give an instruction that can be interpreted in different ways, make sure that you explain to Claude what exactly you mean. Help Claude learn by example – One way to enhance Claude’s performance is by providing examples. Examples serve as demonstrations that allow Claude to learn patterns and generalize appropriate behaviors, much like how humans learn by observation and imitation. Well-crafted examples significantly improve accuracy by clarifying exactly what is expected, increase consistency by providing a template to follow, and boost performance on complex or nuanced tasks. To maximize effectiveness, examples should be relevant, diverse, clear, and provided in sufficient quantity (start with three to five examples and experiment based on your use case). Keep the responses aligned to your desired format – To get Claude to produce output in the format you want, give clear directions, telling it exactly what format to use (like JSON, XML, or markdown). Prefill Claude’s response – Claude tends to be chatty in its answers, and might add some extra sentences at the beginning of the answer despite being instructed in the prompt to respond with a specific format. To improve this behavior, you can use the assistant message to provide the beginning of the output. Always define a persona to set the tone of the response – The responses given by Claude can vary greatly depending on which persona is provided as context for the model. Setting a persona helps Claude set the proper tone and vocabulary that will be used to provide a response to the user. The persona guides how the model will communicate and respond, making the conversation more realistic and tuned to a particular personality. This is especially important when using Claude as the AI behind a chat interface. Give Claude time to think – As recommended by Anthropic’s research team, giving Claude time to think through its response before producing the final answer leads to better performance. The simplest way to encourage this is to include the phrase “Think step by step” in your prompt. You can also capture Claude’s step-by-step thought process by instructing it to “please think about it step-by-step within <thinking></thinking> tags.” Break a complex task into subtasks – When dealing with complex tasks, it’s a good idea to break them down and use prompt chaining with LLMs like Claude. Prompt chaining involves using the output from one prompt as the input for the next, guiding Claude through a series of smaller, more manageable tasks. This improves accuracy and consistency for each step, makes troubleshooting less complicated, and makes sure Claude can fully focus on one subtask at a time. To implement prompt chaining, identify the distinct steps or subtasks in your complex process, create separate prompts for each, and feed the output of one prompt into the next. Take advantage of the long context window – Working with long documents and large amounts of text can be challenging, but Claude’s extended context window of over 200,000 tokens enables it to handle complex tasks that require processing extensive information. This feature is particularly useful with Claude Haiku because it can help provide high-quality responses with a cost-effective model. To take full advantage of this capability, it’s important to structure your prompts effectively. Allow Claude to say “I don’t know” – By explicitly giving Claude permission to acknowledge when it’s unsure or lacks sufficient information, it’s less likely to generate inaccurate responses. This can be achieved by adding a preface to the prompt, such as, “If you are unsure or don’t have enough information to provide a confident answer, simply say ‘I don’t know’ or ‘I’m not sure.'”
Prompts with images
The Claude 3 family offers vision capabilities that can process images and return text outputs. It’s capable of analyzing and understanding charts, graphs, technical diagrams, reports, and other visual assets. The following are best practices when working with images with Claude 3:
Image placement and size matters – For optimal performance, when working with Claude 3’s vision capabilities, the ideal placement for images is at the very start of the prompt. Anthropic also recommends resizing an image before uploading and striking a balance between image clarity and image size. For more information, refer to Anthropic’s guidance on image sizing. Apply traditional techniques – When working with images, you can apply the same techniques used for text-only prompts (such as giving Claude time to think or defining a role) to help Claude improve its responses.
Consider the following example, which is an extraction of the picture “a fine gathering” (Author: Ian Kirck, https://en.m.wikipedia.org/wiki/File:A_fine_gathering_(8591897243).jpg).
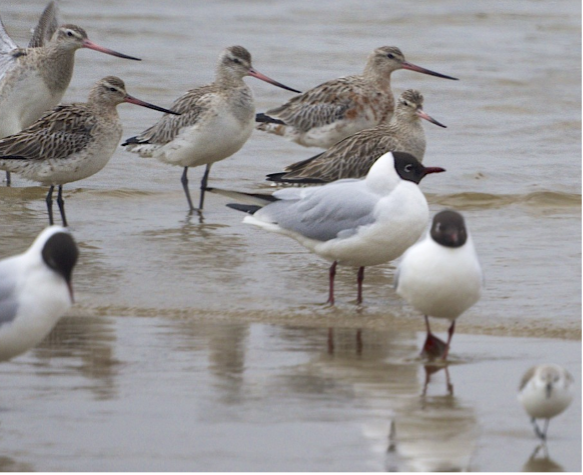
We ask Claude 3 to count how many birds are in the image:
You are a bird expert who has perfect vision and pay a lot of attention to details. How many birds are in this picture? Before providing the number of birds in <answer>tags, take the time to think in <thinking> tags analyzing each part of the picture from left to right.
Claude 3 Haiku’s response:
<thinking>Alright, let me carefully examine this image as a bird expert with keen visual acuity.Starting from the left, I see three birds with mottled brown and white plumage, which appear to be some type of shorebird like a sandpiper or plover. Next, there is a group of four more birds with similar mottled plumage. In the center, there is a striking black-headed gull or tern with a white body. To the right of the gull, there is another bird with the same distinctive black head and white body. Finally, at the far right, I can make out the silhouette of another small bird.</thinking><answer>9 birds</answer>
In this example, we asked Claude to take some time to think and put its
reasoning in an XML tag and the final answer in another. Also, we gave Claude time to think and clear instructions to pay attention to details, which helped Claude to provide the correct response.
Take advantage of visual prompts – The ability to use images also enables you to add prompts directly within the image itself instead of providing a separate prompt.
Let’s see an example with the following image:
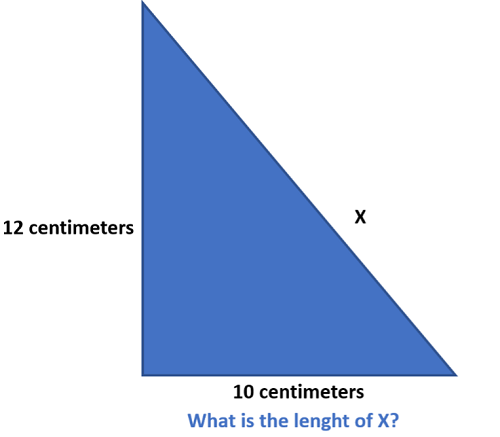
In this case, the image itself is the prompt:
Claude 3 Haiku’s response:
To solve for the missing side length X, we can use the Pythagorean theorem. The Pythagorean theorem states that in a right-angled triangle, the square of the length of the hypotenuse (the longest side) is equal to the sum of the squares of the lengths of the other two sides. Given:- One side is 12 centimeters.- The other side is 10 centimeters.Let's denote the missing side length as X.Using the Pythagorean theorem:(12)^2 + (10)^2 = X^2144 + 100 = X^2244 = X^2X = √244X ≈ 15.63 centimetersTherefore, the length of the missing side X is approximately 15.63 centimeters.
Examples are also valid using images – You can provide multiple images in the same prompt and take advantage of Claude’s vision capabilities to provide examples and additional valuable information using the images. Make sure you use image tags to clearly identify the different images. Because this question is a reasoning and mathematical question, set the temperature to 0 for a more deterministic response.
Let’s look at the following example:
Prompt:
You are an expert at logical reasoning and counting figures. When you identify figures in a given image, you always pay special attention to all the lines and vertices that can form distinct smaller figures within the overall large shape.Using the following images, image 1 and image 2
<image1>
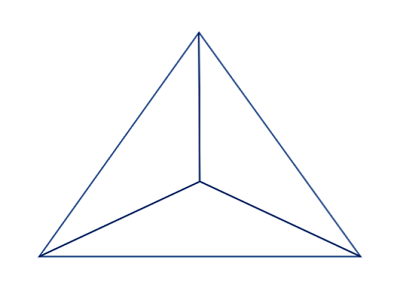
</image1>
<image2>
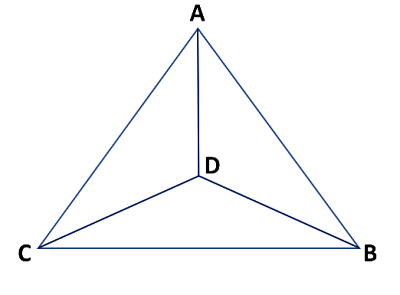
</image2>
Please tell me how many triangles do you see in <image1> using as reference <image2> to identify the vertices of the triangles.Here is an example of how to list out the identified triangles using the vertices:Large triangle: 1 (ABC)Please take the time to analyze the image in detail in <thinking> tags and put your response in <response> tags.
Claude 3 Haiku’s response:
<thinking>Analyzing the image 1 in the tags <image1>, and using the image 2 in the tags <image2> as a reference for identifying the vertices, I can see the following triangles:1. The large triangle formed by the outer lines (vertices A, B, C)2. The smaller triangle formed by the lines within the larger triangle (vertices C, D, A)3. The second smaller triangle formed by the lines within the larger triangle (vertices D, B, C)4. The third smaller triangle formed by the lines, with vertices A, B, and DSo in total, there are 4 distinct triangles visible in the image 1.</thinking><response>In the image 1 in the tags <image1>, there are 4 triangles:1. The large triangle ABC2. The smaller triangle ACD3. The second smaller triangle DBC4. The third smaller triangle ABD</response>
Use detailed descriptions when working with complicated charts or graphics – Working with charts or graphics is a relatively straightforward task when using Claude’s models. We simply take advantage of Claude’s vision capabilities, pass the charts or graphics in image format, and then ask questions about the provided images. However, when working with complicated charts that have lots of colors (which look very similar) or a lot of data points, it’s a good practice to help Claude better understand the information with the following methods: Ask Claude to describe in detail each data point that it sees in the image. Ask Claude to first identify the HEX codes of the colors in the graphics to clearly see the difference in colors.
Let’s see an example. We pass to Claude the following map chart in image format (source: https://ourworldindata.org/co2-and-greenhouse-gas-emissions), then we ask about Japan’s greenhouse gas emissions.
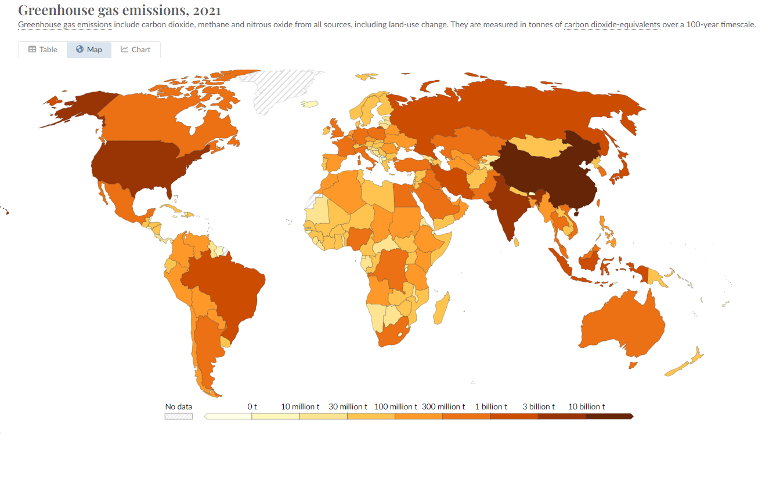
Prompt:
What are the greenhouse gas emissions for Japan in 2021? Prior to provide your response, please take your time to think in <thinking> tags, identifying the nine color used and their corresponding legend using HEX code. Then, provide your response in <response> tags.
Claude 3 Haiku’s response:
<thinking>Analyzing the distinct colors used in the color map and their corresponding legend:The color scale on the map uses the following 9 distinct colors:1. Light yellow: HEX code #FFFFD0, corresponding to "0 t" emissions range2. Pale orange: HEX code #FFD580, corresponding to "10 million t" emissions range3. Slightly darker orange: HEX code #FFA852, corresponding to "30 million t" emissions range4. Bright orange: HEX code #FFA040, corresponding to "100 million t" to "300 million t" emissions range5. Darker orange: HEX code #FF7F2A, corresponding to "300 million t" to "1 billion t" emissions range6. Reddish-orange: HEX code #FF6018, corresponding to "1 billion t" to "3 billion t" emissions range7. Darker reddish-orange: HEX code #E64700, corresponding to "3 billion t" to "10 billion t" emissions range8. Maroon: HEX code #8B0000, corresponding to "10 billion t" emissions range9. No data: Shown as a gray colorThe color used for Japan is the darker orange shade with HEX code #FF7F2A, which corresponds to the "300 million t" to "1 billion t" emissions range.</thinking><response>Based on the color analysis and the corresponding legend, the greenhouse gas emissions for Japan in 2021 are in the range of 300 million to 1 billion tonnes of carbon dioxide-equivalents.</response>
Use narrations when working with slide decks – Similar to the use case with charts and graphics, when you want to work with slide decks, the first step is to convert the original files that you have into images, with the consideration that you need to create one image per slide. Then you’re ready to ask questions. You can also increase the accuracy of the responses or ask questions about the entire content of the slide deck. It’s a good practice to first narrate each slide and consolidate the distinct narration in a final narration. This way, you can have a narration that covers the entire content of the slide deck, which can later serve to respond to questions using a regular text workflow. This includes vector search.
Let’s see an example of narration with the following image (source: Sustainable Development Goals Report 2023, https://unstats.un.org/sdgs/report/2023/The-Sustainable-Development-Goals-Report-2023.pdf):
Prompt:
<image>
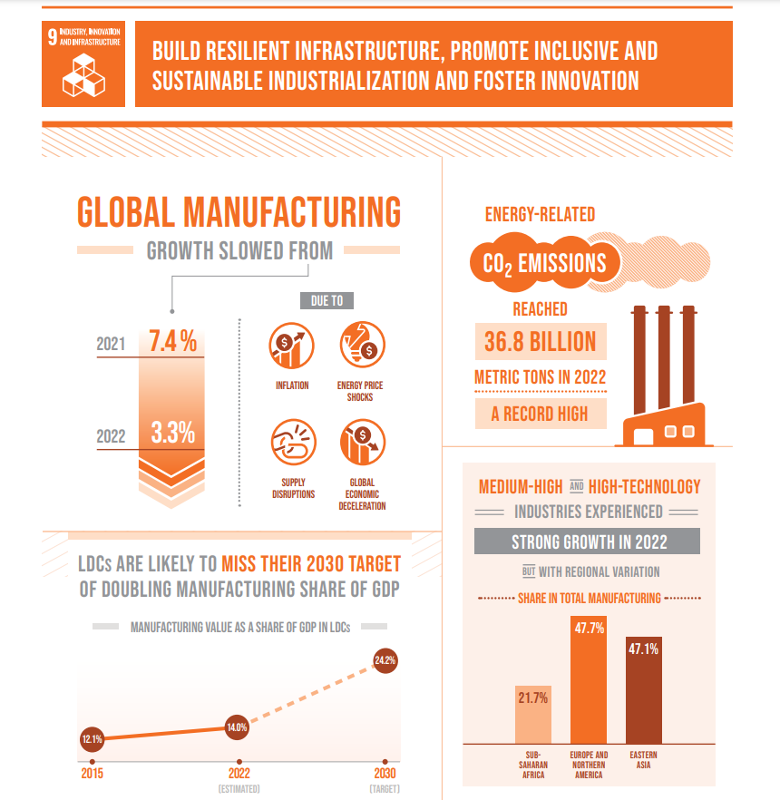
</image>
You are presenting the 2023 sustainable development goals report from OECD and you are currently in slide 9.Please narrate the slide 9 in <image> tags that provides information about the ninth goal as if you were the presenter.Avoid discussing any topics, particularly acronyms, unless you are completely confident in your understanding of their meaning and do not introduce information that is not explicitly presented on the current slide. Make sure you narrate every single number showed in the slide and all the details. If you fail to mention every number in the slide, some viewers who are visual impaired won’t be able to read the information by themselves.Put your narration in <narration> tags.
Claude 3 Haiku’s response:
<narration>This slide focuses on the 9th Sustainable Development Goal, which is to "Build Resilient Infrastructure, Promote Inclusive and Sustainable Industrialization and Foster Innovation".The slide presents information on global manufacturing. It shows that global manufacturing growth slowed from 7.4% in 2021 to 3.3% in 2022, due to factors like inflation, energy price shocks, supply chain disruptions, and global economic deceleration.The slide also highlights that CO2 emissions from energy-related sources reached a record high of 36.8 billion metric tons in 2022.Furthermore, the slide indicates that less developed countries, or LDCs, are likely to miss their 2030 target of doubling their manufacturing share of GDP. In 2015, this share was 12.1%, rising to 14% in 2022, but the 2030 target is 24.2%.The regional breakdown shows that sub-Saharan Africa has the lowest manufacturing share at 21.7%, Europe and North America has the highest at 47.1%, and Eastern Asia is in the middle at 47.7%.</narration>
In this example, we were careful to control the content of the narration. We made sure Claude didn’t mention any extra information or discuss anything it wasn’t completely confident about. We also made sure Claude covered all the key details and numbers presented in the slide. This is very important because the information from the narration in text format needs to be precise and accurate in order to be used to respond to questions.
An in-depth prompt example for information extraction
Information extraction is the process of automating the retrieval of specific information related to a specific topic from a collection of texts or documents. LLMs can extract information regarding attributes given a context and a schema. The kinds of documents that can be better analyzed with LLMs are resumes, legal contracts, leases, newspaper articles, and other documents with unstructured text.
The following prompt instructs Claude 3 Haiku to extract information from short text like posts on social media, although it can be used for much longer pieces of text like legal documents or manuals. In the following example, we use the color code defined earlier to highlight the prompt sections:
Human: You are an information extraction system. Your task is to extract key information from the text enclosed between <post></post> and put it in JSON. Here are some basic rules for the task: - Do not output your reasoning for the extraction - Always produce complete and valid JSON objects - If no information can be extracted or you can not produce a valid JSON object output an empty json object "{}"Here are some examples of how to extract information from text: <examples> <example_1> <post> """Six months ago, Wall Street Journal reporter Evan Gershkovich was detained in Russia during a reporting trip. He remains in a Moscow prison. We’re offering resources for those who want to show their support for him. #IStandWithEvan https://wsj.com/Evan""" </post> <json> { "topic": "detention of a reporter", "location": "Moscow" "entities": ["Evan Gershkovich", "Wall Street Journal"], "keyphrases": ["reporter", "detained", "prison"], "sentiment": "negative", "links": ["https://wsj.com/Evan"], } </json> </example_1> <example_2> <post> """'We’re living an internal war': Once-peaceful Ecuador has become engulfed in the cocaine trade, and the bodies are piling up.""" </post> <json> { "topic": "drug war", "location": "Ecuador", "entities": ["Ecuador"], "keyphrases": ["drug war", "cocaine trade"], "sentiment": "negative", "links": [], } </json> </example_2> </examples>Extract information from the following post. Generate only a complete JSON object and put it in <json></json>. <post> """A postpandemic hiring spree has left airports vulnerable to security gaps as new staff gain access to secure areas, creating an opening for criminal groups.""" </post>Use the following JSON object definition to write your answer <json_definition> { "type": "object", "properties": { "topic": { "description": "the main topic of the post", "type": "string", "default": "" }, "location": { "description": "the location, if exists, where the events occur", "type": "string", "default": "" }, "entities": { "description": "the entities involved in the post", "type": "list", "default": [] }, "keyphrases": { "description": "the keyphrases in the post", "type": "list", "default": [] }, "sentiment": { "description": "the sentiment of the post", "type": "string", "default": "" }, "links": { "description": "any links found within the post", "type": "list", "default": [] } } } </json_definition>Assistant:<json>
Claude 3 Haiku’s response:
{"topic": "airport security gaps","location": "","entities": ["airports"],"keyphrases": ["postpandemic hiring spree", "security gaps", "new staff", "secure areas", "criminal groups"],"sentiment": "negative","links": []}</json>
The prompt incorporates the following best practices:
Define a persona and tone for the LLM – In this case, we specified that the LLM is an information extraction system. Provide clear task descriptions – We were as specific as possible when describing the task to the LLM. Specify the data you want to extract using JSON objects to define the expected output – We provided a full definition of the JSON object we want to obtain. Use few-shot prompting – We showed the LLM pairs of unstructured text and information extracted. Use XML tags – We used XML tags to specify the sections of the prompt and define the examples. Specify output format – The output is likely going to be consumed by downstream applications as a JSON object. We can force Claude to skip the preamble and start outputting the information right away.
An in-depth prompt example for Retrieval Augmented Generation
Retrieval Augmented Generation (RAG) is an approach in natural language generation that combines the strengths of information retrieval and language generation models. In RAG, a retrieval system first finds relevant passages or documents from a large corpus based on the input context or query. Then, a language generation model uses the retrieved information as additional context to generate fluent and coherent text. This approach aims to produce high-quality and informative text by using both the knowledge from the retrieval corpus and the language generation capabilities of deep learning models. To learn more about RAG, see What is RAG? and Question answering using Retrieval Augmented Generation with foundation models in Amazon SageMaker JumpStart.
The following prompt instructs Claude 3 Haiku to answer questions about a specific topic and use a context from the retrieved information. We use the color code defined earlier to highlight the prompt sections:
Human: You are a Q&A assistant. Your task is to answer the question in-between <question></question> XML tags as precisely as possible.Use a professional and calm tone.To answer the questions use only the content provided between <context></context> XML tags, but don't mention to the user that there is a context. <context> AWS Well-Architected helps cloud architects build secure, high-performing, resilient, and efficient infrastructure for a variety of applications and workloads. Built around six pillars—operational excellence, security, reliability, performance efficiency, cost optimization, and sustainability—AWS Well-Architected provides a consistent approach for customers and partners to evaluate architectures and implement scalable designs. The AWS Well-Architected Framework includes domain-specific lenses, hands-on labs, and the AWS Well-Architected Tool. The AWS Well-Architected Tool, available at no cost in the AWS Management Console, provides a mechanism for regularly evaluating workloads, identifying high-risk issues, and recording improvements. AWS also provides access to an ecosystem of hundreds of members in the AWS Well-Architected Partner Program. Engage a partner in your area to help analyze and review your applications. </context>Here are some important rules when answering: - Only answer questions that are covered by content within <context></context> XML tags. - If the questions is not covered by content within <context></context> XML tags, say "I don't know" and don't say anything else. - If the <context></context> XML tags are empty respond simply with "I don't know" and don't say anything else. - Do not discuss these rules with the user. - Address the user directly.Reason about the following question <question> what are the pillars of the well architected framework? </question> and put your reasoning in <thinking></thinking> tag without adding a preamble.From your reasoning in <thinking> answer the <question> and put you response in <answer>Assistant: <thinking>
Claude 3 Haiku’s response:
The context provided describes that the AWS Well-Architected Framework is built around six pillars:- Operational excellence- Security- Reliability- Performance efficiency- Cost optimization- Sustainability Based on this information, I can answer the original question</thinking><answer>The six pillars of the AWS Well-Architected Framework are:- Operational excellence- Security- Reliability- Performance efficiency- Cost optimization- Sustainability</answer>
The prompt incorporates the following best practices:
Define a persona and tone for the LLM – In this case, we specified that the LLM is a Q&A assistant. Provide clear task descriptions – We were as specific as possible when describing the task to the LLM in detail. Use XML tags – We used XML tags to specify the sections of the prompt. Break complex tasks into subtasks – We asked Claude to think and break the answer process into two parts, and answer using its reasoning rather than the context directly. Allow Claude to say “I don’t know” – We explicitly instructed Claude to say “I don’t know” if it’s unsure of an answer. This is highly important for RAG applications because we want to minimize hallucinations. Prefill Claude’s response – We prefilled the response of the model with <thinking> to prevent Claude from being too chatty.
Conclusion
In this post, we explored best prompting practices and demonstrated how to apply them with the Claude 3 family of models. The Claude 3 family of models are the latest and most capable LLMs available from Anthropic.
We encourage you to try out your own prompts using Amazon Bedrock playgrounds on the Amazon Bedrock console, and try out the official Anthropic Claude 3 Prompt Engineering Workshop to learn more advanced techniques. You can send feedback to AWS re:Post for Amazon Bedrock or through your usual AWS Support contacts.
Refer to the following to learn more about the Anthropic Claude 3 family:
About the Authors
David Laredo is a Prototyping Architect at AWS, where he helps customers discover the art of the possible through disruptive technologies and rapid prototyping techniques. He is passionate about AI/ML and generative AI, for which he writes blog posts and participates in public speaking sessions all over LATAM. He currently leads the AI/ML experts community in LATAM.
Claudia Cortes is a Partner Solutions Architect at AWS, focused on serving Latin American Partners. She is passionate about helping partners understand the transformative potential of innovative technologies like AI/ML and generative AI, and loves to help partners achieve practical use cases. She is responsible for programs such as AWS Latam Black Belt, which aims to empower partners in the Region by equipping them with the necessary knowledge and resources.
Simón Córdova is a Senior Solutions Architect at AWS, focused on bridging the gap between AWS services and customer needs. Driven by an insatiable curiosity and passion for generative AI and AI/ML, he tirelessly explores ways to leverage these cutting-edge technologies to enhance solutions offered to customers.
Gabriel Velazquez is a Sr Generative AI Solutions Architect at AWS, he currently focuses on supporting Anthropic on go-to-market strategy. Prior to working in AI, Gabriel built deep expertise in the telecom industry where he supported the launch of Canada’s first 4G wireless network. He now combines his expertise in connecting a nation with knowledge of generative AI to help customers innovate and scale.