The rapid progress in artificial intelligence (AI) and machine learning (ML) research underscores the importance of accurately evaluating AI agents’ capabilities in replicating complex, empirical research tasks traditionally performed by human researchers. Currently, systematic evaluation tools that precisely measure the ability of AI agents to autonomously reproduce ML research findings remain limited, posing challenges in fully understanding the potential and limitations of such systems.
OpenAI has introduced PaperBench, a benchmark designed to evaluate the competence of AI agents in autonomously replicating state-of-the-art machine learning research. PaperBench specifically measures whether AI systems can accurately interpret research papers, independently develop the necessary codebases, and execute experiments to replicate empirical outcomes. The benchmark comprises 20 papers selected from ICML 2024, covering areas including reinforcement learning, robustness, and probabilistic methods. Detailed rubrics, co-developed with original paper authors, specify 8,316 individually gradable tasks to facilitate precise evaluation of AI capabilities.

From a technical perspective, PaperBench requires AI agents to process provided research papers and supplementary clarifications to develop comprehensive code repositories from scratch. These repositories must include complete experimental setups and execution scripts, notably the reproduce.sh file. To ensure genuine independent replication, agents are prohibited from referencing or reusing code from the original authors’ repositories. Rubrics are structured hierarchically to detail explicit pass-fail criteria at various levels, allowing systematic and objective assessment. Evaluation is conducted using SimpleJudge, an automated large language model (LLM)-based judge, which simplifies the grading process. SimpleJudge achieved an F1 score of 0.83 on JudgeEval, an auxiliary evaluation dataset specifically designed to validate automated grading accuracy.
Empirical evaluations of several advanced AI models indicate varying performance levels on PaperBench. Claude 3.5 Sonnet exhibited the highest capability with an average replication score of 21.0%. Other models such as OpenAI’s GPT-4o and Gemini 2.0 Flash attained significantly lower scores of 4.1% and 3.2%, respectively. Comparatively, expert human ML researchers achieved considerably higher accuracy, reaching up to 41.4% after 48 hours of dedicated effort. Analysis of model performance revealed strengths in initial rapid code generation and early experimental setup but highlighted substantial weaknesses in managing prolonged tasks, troubleshooting, and adapting strategic approaches over time.
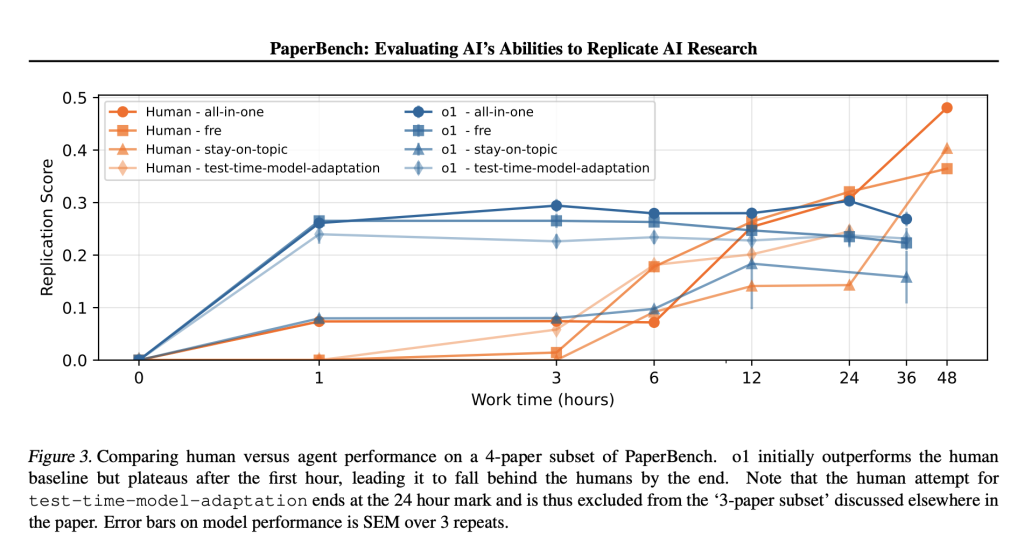
These results provide critical technical insights into current AI system capabilities. While AI models demonstrate competence in certain coding tasks and initial experiment implementation, significant gaps persist, particularly regarding sustained task execution, adaptive problem-solving, and strategic planning. Additionally, the introduction of PaperBench Code-Dev, a streamlined variant emphasizing code correctness without experimental execution, offers a practical alternative for broader and resource-limited community use due to reduced computational and evaluation costs.
In summary, PaperBench represents an important step toward methodically evaluating AI research capabilities. It provides a structured and detailed assessment environment that highlights specific strengths and limitations of contemporary AI models relative to human performance. The collaborative development of rubrics ensures precise and realistic evaluations. OpenAI’s open-sourcing of PaperBench supports further exploration and development in the field, enhancing understanding of autonomous AI research capabilities and informing responsible progression in this area.
Check out the Paper and GitHub page. All credit for this research goes to the researchers of this project. Also, feel free to follow us on Twitter and don’t forget to join our 85k+ ML SubReddit.
The post Open AI Releases PaperBench: A Challenging Benchmark for Assessing AI Agents’ Abilities to Replicate Cutting-Edge Machine Learning Research appeared first on MarkTechPost.