A critical advancement in recent times has been exploring reinforcement learning (RL) techniques to improve LLMs beyond traditional supervised fine-tuning methods. RL allows models to learn optimal responses through reward signals, enhancing their reasoning and decision-making capabilities. RL introduces a feedback-driven training loop that better aligns with human-like learning processes, particularly in tasks involving step-by-step problem-solving or math reasoning. This intersection of LLMs and RL is becoming a prominent area for academic research and industry innovation.
A central challenge in improving LLMs for complex reasoning tasks is ensuring these models develop better thinking skills rather than longer outputs. In reinforcement learning-based training of LLMs, a pattern has emerged where models begin generating excessively long responses without necessarily improving answer quality. This raises concerns about optimization biases in RL methods that may favor verbosity over correctness. Another complication arises from the base models themselves; some already show signs of reasoning capabilities, which makes it difficult to isolate the real impact of RL tuning. Therefore, understanding how training strategies and model foundations affect final performance becomes essential.
Previously, reinforcement learning post-training for LLMs often relied on algorithms like Proximal Policy Optimization (PPO), commonly used in various open-source implementations. These implementations frequently included a response-length normalization step, which inadvertently introduced biases favoring longer or shorter outputs depending on the correctness of the response. In particular, Group Relative Policy Optimization (GRPO) was introduced as a variant to optimize policy updates at the group level. While effective, GRPO has been criticized for embedding subtle optimization biases that affect the length and quality of model responses. These existing techniques, though innovative, have shown limitations that obscure the actual gains from reinforcement learning.
Researchers from Sea AI Lab, the National University of Singapore, and Singapore Management University introduced a new approach called Dr. GRPO (Group Relative Policy Optimization Done Right) to address these issues. This method removes the problematic normalization terms from the GRPO formulation. Specifically, it eliminates the response length and standard deviation scaling factors that caused imbalances in model updates. The revised algorithm computes gradients more fairly across different responses and question types. They applied this method to train Qwen2.5-Math-7B, an open-source base model and demonstrated its effectiveness on multiple benchmarks. The training process used 27 hours of computing on 8× A100 GPUs, a relatively modest setup considering the results achieved.
The researchers tested their method on prominent math reasoning benchmarks, including AIME 2024, AMC, MATH500, Minerva Math, and OlympiadBench. The model trained with Dr. GRPO achieved 43.3% accuracy on AIME 2024, significantly outperforming SimpleRL-Zero-7B (36.0%), Prime-Zero-7B (27.6%), and OpenReasoner-Zero-7B (16.7%). It also demonstrated strong average performance across all tasks: 40.9% on MATH500, 45.8% on Minerva, and 62.7% on OlympiadBench. These results validate the effectiveness of the bias-free RL method. Importantly, the model performed better and showed more efficient token usage. Incorrect responses became shorter and more focused, a notable shift from previous training methods encouraging overextended answers regardless of correctness.

Beyond the training algorithm, the team also examined the nature of base models used in R1-Zero-like RL settings. They found that some models, such as Qwen2.5, display advanced capabilities even before training, possibly due to pretraining on concatenated question-answer data. For example, the Qwen2.5-Math-7B model achieved 38.2% average accuracy without any RL fine-tuning, outperforming many models trained using traditional methods. This preexisting reasoning capacity complicates claims about the benefits of RL, as improvements may partly stem from prior training strategies rather than new learning through reinforcement. DeepSeek-V3-Base, another examined model, showed spontaneous “Aha moments” and instances of self-reflection before RL, further suggesting that some reasoning skills may already be embedded in base models.
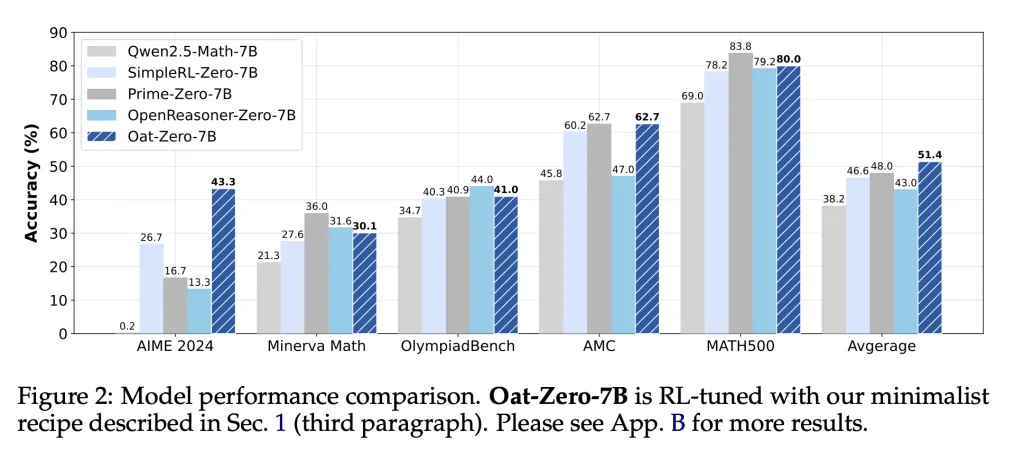
The performance dynamics were carefully tracked during training. Using Dr. GRPO, models avoided the tendency to inflate response lengths. The evaluation revealed that Dr. GRPO kept output lengths stable while increasing reward signals, suggesting a direct correlation between training and improved accuracy, not just verbosity. In contrast, traditional GRPO led to progressively longer incorrect responses, falsely indicating improvement. This observation aligns with findings that many open-source PPO implementations unwittingly introduce response-length bias, a flaw inherited from pretraining practices.

The researchers also explored how different templates and question sets influence model behavior. The Qwen2.5-Math-1.5B base model performed best without prompt templates, scoring 61.6% on Minerva Math and 45.8% on MATH500. Surprisingly, using templates often decreased performance before RL recovered it. This highlights how mismatches between model pretraining and inference format can obscure true reasoning capabilities. Also, models trained on small, simple question sets like GSM-8K often outperformed those trained on larger datasets, challenging the assumption that broader coverage always leads to better reasoning.
Several Key Takeaways from the Research include the following:
- DeepSeek-V3-Base and Qwen2.5 models exhibit reasoning capabilities even before RL, indicating strong pretraining effects.Dr. GRPO eliminates biases in GRPO by removing length and reward normalization terms, improving token efficiency.The Qwen2.5-Math-7B model, trained with Dr. GRPO, achieved:
- 43.3% on AIME 2024 62.7% on OlympiadBench 45.8% on Minerva Math 40.9% on MATH500 The average score across all benchmarks: 40.3%
In conclusion, the study reveals critical insights into how RL affects large language model behavior. Researchers found that pretraining plays a substantial role in determining baseline capabilities. They also demonstrated that optimization biases in popular RL algorithms can mislead training and evaluation. The introduction of Dr. GRPO corrected these issues, leading to more interpretable and efficient model training. With only 27 hours of training, their model reached state-of-the-art results on major math reasoning benchmarks. These findings reshape how the community should evaluate RL-enhanced LLMs, focusing more on method transparency and base model characteristics than on mere performance metrics.
Check out the Paper and GitHub Page. All credit for this research goes to the researchers of this project. Also, feel free to follow us on Twitter and don’t forget to join our 85k+ ML SubReddit.
The post Sea AI Lab Researchers Introduce Dr. GRPO: A Bias-Free Reinforcement Learning Method that Enhances Math Reasoning Accuracy in Large Language Models Without Inflating Responses appeared first on MarkTechPost.