While everyone's been buzzing about AI agents and automation, AMD and Johns Hopkins University have been working on improving how humans and AI collaborate in research. Their new open-source framework, Agent Laboratory, is a complete reimagining of how scientific research can be accelerated through human-AI teamwork.
After looking at numerous AI research frameworks, Agent Laboratory stands out for its practical approach. Instead of trying to replace human researchers (like many existing solutions), it focuses on supercharging their capabilities by handling the time-consuming aspects of research while keeping humans in the driver's seat.
The core innovation here is simple but powerful: Rather than pursuing fully autonomous research (which often leads to questionable results), Agent Laboratory creates a virtual lab where multiple specialized AI agents work together, each handling different aspects of the research process while staying anchored to human guidance.
Breaking Down the Virtual Lab
Think of Agent Laboratory as a well-orchestrated research team, but with AI agents playing specialized roles. Just like a real research lab, each agent has specific responsibilities and expertise:
- A PhD agent tackles literature reviews and research planning
- Postdoc agents help refine experimental approaches
- ML Engineer agents handle the technical implementation
- Professor agents evaluate and score research outputs
What makes this system particularly interesting is its workflow. Unlike traditional AI tools that operate in isolation, Agent Laboratory creates a collaborative environment where these agents interact and build upon each other's work.
The process follows a natural research progression:
- Literature Review: The PhD agent scours academic papers using the arXiv API, gathering and organizing relevant research
- Plan Formulation: PhD and postdoc agents team up to create detailed research plans
- Implementation: ML Engineer agents write and test code
- Analysis & Documentation: The team works together to interpret results and generate comprehensive reports
But here's where it gets really practical: The framework is compute-flexible, meaning researchers can allocate resources based on their access to computing power and budget constraints. This makes it a tool designed for real-world research environments.
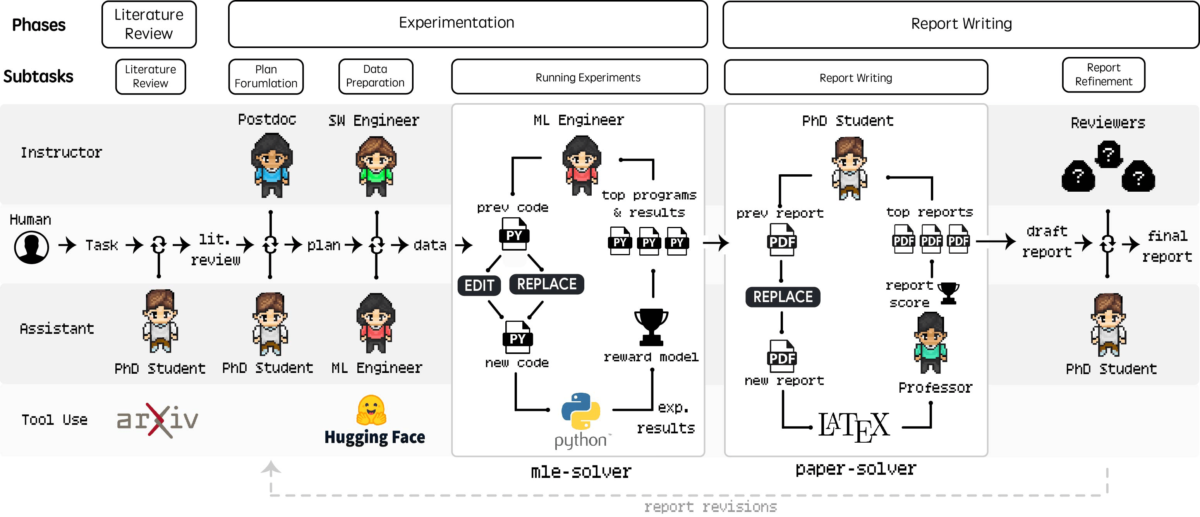
Schmidgall et al.
The Human Factor: Where AI Meets Expertise
While Agent Laboratory packs impressive automation capabilities, the real magic happens in what they call “co-pilot mode.” In this setup, researchers can provide feedback at each stage of the process, creating a genuine collaboration between human expertise and AI assistance.
The co-pilot feedback data reveals some compelling insights. In the autonomous mode, Agent Laboratory-generated papers scored an average of 3.8/10 in human evaluations. But when researchers engaged in co-pilot mode, those scores jumped to 4.38/10. What is particularly interesting is where these improvements showed up – papers scored significantly higher in clarity (+0.23) and presentation (+0.33).
But here is the reality check: even with human involvement, these papers still scored about 1.45 points below the average accepted NeurIPS paper (which sits at 5.85). This is not a failure, but it is a crucial learning about how AI and human expertise need to complement each other.
The evaluation revealed something else fascinating: AI reviewers consistently rated papers about 2.3 points higher than human reviewers. This gap highlights why human oversight remains crucial in research evaluation.
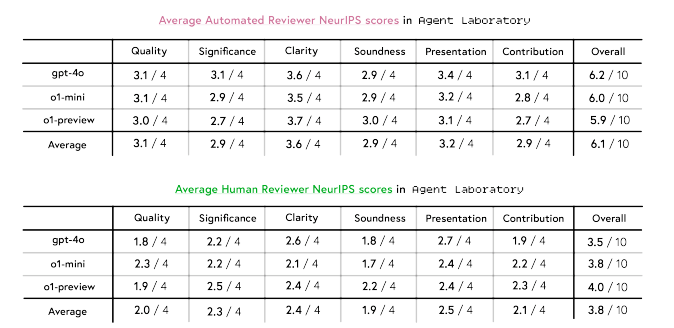
Schmidgall et al.
Breaking Down the Numbers
What really matters in a research environment? The cost and performance. Agent Laboratory's approach to model comparison reveals some surprising efficiency gains in this regard.
GPT-4o emerged as the speed champion, completing the entire workflow in just 1,165.4 seconds – that's 3.2x faster than o1-mini and 5.3x faster than o1-preview. But what is even more important is that it only costs $2.33 per paper. Compared to previous autonomous research methods that cost around $15, we are looking at an 84% cost reduction.
Looking at model performance:
- o1-preview scored highest in usefulness and clarity
- o1-mini achieved the best experimental quality scores
- GPT-4o lagged in metrics but led in cost-efficiency
The real-world implications here are significant.
Researchers can now choose their approach based on their specific needs:
- Need rapid prototyping? GPT-4o offers speed and cost efficiency
- Prioritizing experimental quality? o1-mini might be your best bet
- Looking for the most polished output? o1-preview shows promise
This flexibility means research teams can adapt the framework to their resources and requirements, rather than being locked into a one-size-fits-all solution.
A New Chapter in Research
After looking into Agent Laboratory's capabilities and results, I am convinced that we are looking at a significant shift in how research will be conducted. But it is not the narrative of replacement that often dominates headlines – it is something far more nuanced and powerful.
While Agent Laboratory's papers are not yet hitting top conference standards on their own, they are creating a new paradigm for research acceleration. Think of it like having a team of AI research assistants who never sleep, each specializing in different aspects of the scientific process.
The implications for researchers are profound:
- Time spent on literature reviews and basic coding could be redirected to creative ideation
- Research ideas that might have been shelved due to resource constraints become viable
- The ability to rapidly prototype and test hypotheses could lead to faster breakthroughs
Current limitations, like the gap between AI and human review scores, are opportunities. Each iteration of these systems brings us closer to more sophisticated research collaboration between humans and AI.
Looking ahead, I see three key developments that could reshape scientific discovery:
- More sophisticated human-AI collaboration patterns will emerge as researchers learn to leverage these tools effectively
- The cost and time savings could democratize research, allowing smaller labs and institutions to pursue more ambitious projects
- The rapid prototyping capabilities could lead to more experimental approaches in research
The key to maximizing this potential? Understanding that Agent Laboratory and similar frameworks are tools for amplification, not automation. The future of research isn't about choosing between human expertise and AI capabilities – it's about finding innovative ways to combine them.
The post Agent Laboratory: A Virtual Research Team by AMD and Johns Hopkins appeared first on Unite.AI.