Protein docking, the process of predicting the structure of protein-protein complexes, remains a complex challenge in computational biology. While advances like AlphaFold have transformed sequence-to-structure prediction, accurately modeling protein interactions is often complicated by conformational flexibility, where proteins undergo structural changes upon binding. For example, AlphaFold-multimer (AFm), an extension of AlphaFold, achieves a success rate of only 43% in modeling complex interactions, particularly for targets requiring significant structural adjustments. These challenges are especially evident in highly flexible targets, such as antibody-antigen complexes, which are further complicated by sparse evolutionary data. Conventional physics-based docking tools like ReplicaDock 2.0 address some aspects of these issues but often struggle with efficiency and adaptability, highlighting the need for approaches that combine multiple strengths.
Researchers at Johns Hopkins have introduced AlphaRED, a docking pipeline that integrates AlphaFold’s predictive capabilities with ReplicaDock 2.0’s physics-based sampling methods. AlphaRED is designed to address the specific challenges of conformational flexibility and binding site prediction. By leveraging AlphaFold-multimer’s confidence metrics, such as the predicted Local Distance Difference Test (pLDDT), the pipeline identifies flexible protein regions and refines docking predictions for improved accuracy. For challenging cases like antibody-antigen targets, AlphaRED demonstrates a success rate of 43%, doubling AlphaFold-multimer’s performance. Additionally, it generates CAPRI acceptable-quality models for 63% of benchmark targets, compared to AlphaFold’s 43%. This approach effectively combines the strengths of deep learning and physics-based methods to improve protein complex prediction.

Technical Details and Benefits
AlphaRED begins by using AlphaFold-multimer to generate structural templates, which are then evaluated based on interface-specific pLDDT scores. When predictions show low interface confidence, the pipeline employs ReplicaDock 2.0 for global docking simulations, using replica exchange Monte Carlo to explore diverse conformations. For high-confidence models, AlphaRED performs localized refinements, focusing on backbone flexibility in regions indicated by low per-residue pLDDT scores. This targeted approach captures binding-induced conformational changes and improves prediction accuracy. By combining the complementary strengths of machine learning and physics-based sampling, AlphaRED addresses scenarios involving high flexibility or limited evolutionary data more effectively than either approach alone.
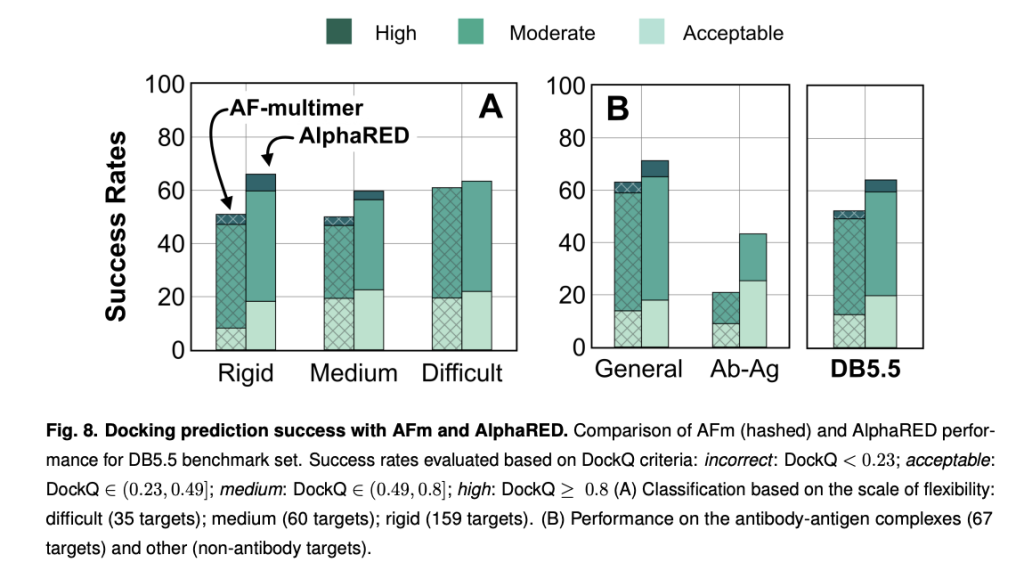
Results and Insights
AlphaRED was tested on a curated dataset of 254 targets, including rigid, medium, and highly flexible protein complexes. It showed significant improvements across all categories, with notable success in antibody-antigen docking. For instance, AlphaRED’s DockQ scores exceeded 0.23 for 63% of the dataset, compared to 43% for AlphaFold-multimer. In blind evaluations like CASP15, AlphaRED excelled, particularly in nanobody-antigen complexes where AlphaFold struggled due to limited co-evolutionary information. Additionally, AlphaRED significantly reduced interface root mean square deviations (RMSDs), refining initial AlphaFold predictions into models closer to native structures. These results suggest that AlphaRED holds promise for applications in therapeutic antibody design and structural biology.
Conclusion
AlphaRED offers a thoughtful integration of AlphaFold’s deep learning capabilities with the adaptive sampling techniques of ReplicaDock 2.0. This pipeline enhances docking accuracy while providing a practical solution for complex cases involving conformational flexibility. Its demonstrated success in challenging docking scenarios, such as antibody-antigen complexes and blind evaluations, makes it a valuable tool for advancing structural biology and drug discovery. By effectively combining the strengths of machine learning and physics-based approaches, AlphaRED represents an important step forward in reliable protein complex prediction and opens new possibilities for research in computational biology.
Check out the Paper and GitHub Page. All credit for this research goes to the researchers of this project. Also, don’t forget to follow us on Twitter and join our Telegram Channel and LinkedIn Group. Don’t Forget to join our 60k+ ML SubReddit.
FREE UPCOMING AI WEBINAR (JAN 15, 2025): Boost LLM Accuracy with Synthetic Data and Evaluation Intelligence–Join this webinar to gain actionable insights into boosting LLM model performance and accuracy while safeguarding data privacy.
The post Enhancing Protein Docking with AlphaRED: A Balanced Approach to Protein Complex Prediction appeared first on MarkTechPost.