Aligning large language models (LLMs) with human preferences is an essential task in artificial intelligence research. However, current reinforcement learning (RL) methods face notable challenges. Proximal Policy Optimization (PPO) and similar techniques often demand extensive online sampling, which can lead to high computational costs and instability. Offline RL methods like Direct Preference Optimization (DPO) avoid these issues but face difficulties with tasks requiring multi-step reasoning, such as solving mathematical problems or generating complex code. These methods frequently treat the generation process as a single-step problem, neglecting the long-horizon dependencies intrinsic to many reasoning tasks. Additionally, sparse reward functions, which provide feedback only at the conclusion of a reasoning sequence, make intermediate step guidance challenging.
Researchers from ByteDance and UCLA have introduced Direct Q-function Optimization (DQO) to address these challenges. DQO frames the response generation process as a Markov Decision Process (MDP) and utilizes the Soft Actor-Critic (SAC) framework. By parameterizing the Q-function directly through the language model, DQO shifts the LLM alignment problem into a structured, step-by-step learning process. Unlike bandit-based methods, DQO incorporates process rewards—intermediate feedback signals—to support multi-step reasoning more effectively.
A key feature of DQO is its ability to identify and optimize correct reasoning steps even within partially correct responses. For example, in mathematical problem-solving, DQO assigns higher value to accurate steps and penalizes errors, enabling incremental improvement in reasoning. This makes DQO particularly suitable for tasks requiring detailed, long-horizon decision-making.
Technical Implementation and Practical Advantages
DQO’s approach is centered on parameterizing the Q-function using the language model, thereby integrating policy and value functions. The model updates its Q-function and value function based on the Soft Bellman Equation. KL-regularization ensures stable learning and helps prevent overfitting to specific samples.
To handle challenges such as high bias in temporal difference errors, DQO employs λ-return, a mechanism that balances short-term and long-term rewards for more stable training. Importance sampling further enhances DQO’s offline learning capabilities by reducing distributional shifts between the training data and the model’s policy.
DQO offers several practical advantages. It eliminates the need for online sampling, reducing computational costs. Moreover, it can learn from unbalanced and negative samples, enhancing its robustness across various scenarios. The use of process rewards helps refine reasoning capabilities while improving alignment with task requirements.

Results and Insights
Experimental evaluations of DQO on mathematical reasoning datasets—GSM8K and MATH—demonstrate its effectiveness. On the GSM8K dataset, DQO improved performance from a baseline of 59.06% to 87.26% for greedy generation and from 53.30% to 84.69% for sampling-based generation. These results surpass other baseline methods, including DPO and DRO. Similarly, on the MATH dataset, DQO outperformed baselines, achieving improvements of 1.18% in sampling and 1.40% in greedy generation.
Enhancing DQO with process rewards further boosted performance, suggesting its potential to incorporate additional supervisory signals. These results underscore DQO’s capability to handle multi-step reasoning tasks effectively and align LLMs with complex objectives.
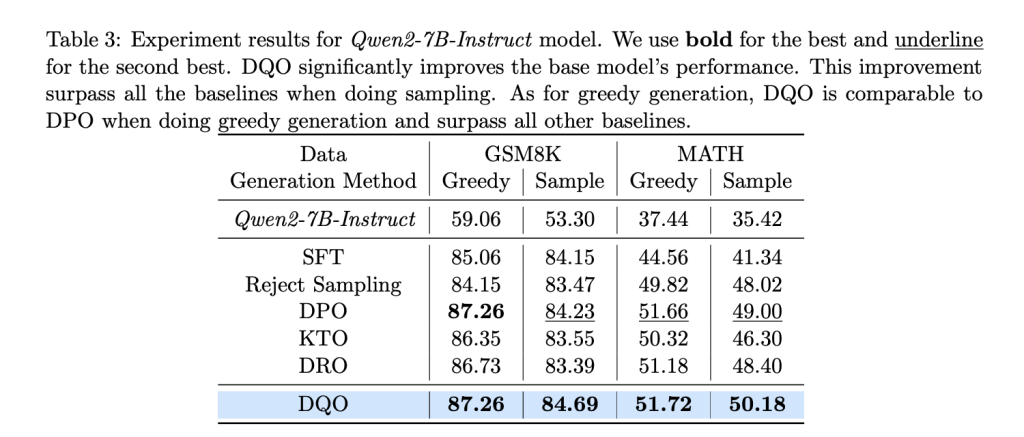
Conclusion
Direct Q-function Optimization (DQO) offers a thoughtful approach to reinforcement learning for LLM alignment. By framing response generation as an MDP and utilizing the SAC framework, DQO addresses the limitations of existing methods. Its ability to integrate process rewards, handle unbalanced data, and stabilize training through λ-return and importance sampling makes it a practical solution for tasks involving multi-step reasoning.
Future research could explore applying DQO to other domains, such as code generation and dialogue systems, where long-horizon decision-making is critical. As AI systems evolve to tackle increasingly complex challenges, methods like DQO will play an important role in enhancing the alignment and performance of language models.
Check out the Paper. All credit for this research goes to the researchers of this project. Also, don’t forget to follow us on Twitter and join our Telegram Channel and LinkedIn Group. Don’t Forget to join our 60k+ ML SubReddit.
The post Revolutionizing LLM Alignment: A Deep Dive into Direct Q-Function Optimization appeared first on MarkTechPost.