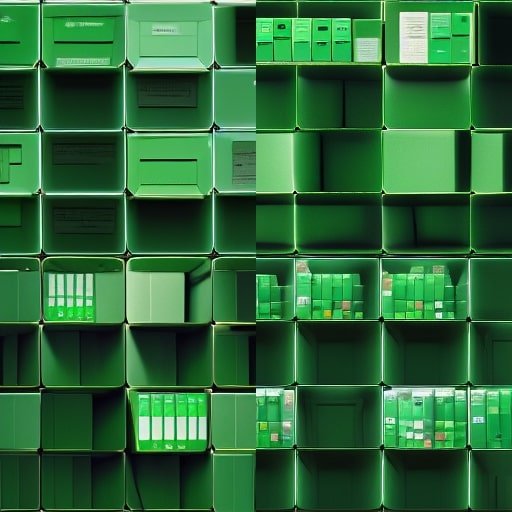
A recurrent neural network (RNN) is a type of neural network that is designed to handle sequences of data. Unlike a traditional neural network, which assumed that all inputs were independent of one another, an RNN is able to maintain a "memory" of sorts, making it well-suited for tasks such as language modeling and machine translation. RNNs are typically constructed using a series of hidden layers, each of which contains a set of units known as neurons. When processing an input sequence, the neurons in the first hidden layer will each receive a separate piece of the input. They will then pass this information on to the neurons in the second hidden layer, and so on until the final output is produced. It is this ability to make use of previous inputs that gives RNNs their power. However, training an RNN can be difficult due to the vanishing gradient problem. This occurs when the gradient signal becomes too weak to propagate back through the network, making it difficult for the network to learn from error signals. Nevertheless, recent advances in deep learning have shown that RNNs are capable of producing state-of-the-art results on a variety of tasks.
MIT Introduction to Deep Learning 6.S191: Lecture 2
Recurrent Neural Networks
Lecturer: Ava Soleimany
A recurrent neural network (RNN) is a type of artificial neural network where connections between nodes form a directed graph along a temporal sequence. This allows it to exhibit temporal dynamic behavior. Unlike feedforward neural networks, RNNs can use their internal state (memory) to process sequences of inputs. This makes them applicable to tasks such as unsegmented, connected handwriting recognition or speech recognition. RNNs were created in the 1980s and were originally called connectionist temporal classification (CTC) models. The first working RNN was published by Jürgen Schmidhuber in 1991. Many different types of RNNs have been created, including long short-term memory (LSTM) networks and GRU networks. RNNs can be used for many tasks, including machine translation, image captioning, and text classification.
ELI5: Explain recurrent neural networks like I’m 5
Imagine you have a special kind of brain called a Recurrent Neural Network (RNN). This brain is different from a regular brain because it can remember things from the past and use that information to understand what's happening right now.
Let's say you're playing with building blocks, and you have a friend who loves to play with you. Every time you add a new block, your friend tells you the color of the block. But here's the cool part: your friend also tells you the color of the block you added before!
Now, with this information, your special brain starts to understand patterns. It remembers the colors of the blocks you've been adding and can predict the color of the next block based on the previous ones. So, if you had a blue block and then a red block, your brain might guess that the next block will be yellow because it remembers that the pattern was blue-red-yellow.
The RNN works similarly. It has a memory that helps it remember the information from the past. It takes in data, like words or numbers, one at a time, just like you add blocks one at a time. And at each step, it uses the current input and the information it remembers from the past to make predictions or understand what the data means.
This kind of brain is really useful when dealing with things that happen over time, like understanding the meaning of a sentence or predicting the next word in a sentence. It can also be helpful in tasks like translating languages or recognizing handwriting because it can remember the context and use that to make better predictions.
So, just like you and your friend playing with blocks, a Recurrent Neural Network has a special memory that helps it understand things that happen in a sequence and make predictions based on that sequence.