This month, I am covering three new papers related to instruction finetuning and parameter-efficient finetuning with LoRA in large language models (LLMs). I work with these methods on a daily basis, so it's always exciting to see new research that provides practical insights.
This article might be a bit shorter than usual since I am currently finishing the final chapter of my book, "Build a Large Language Model From Scratch." Additionally, I am preparing for a virtual ACM Tech Talk on LLMs this Wednesday. The talk is free and open to all, and you are very welcome to join if you're interested!
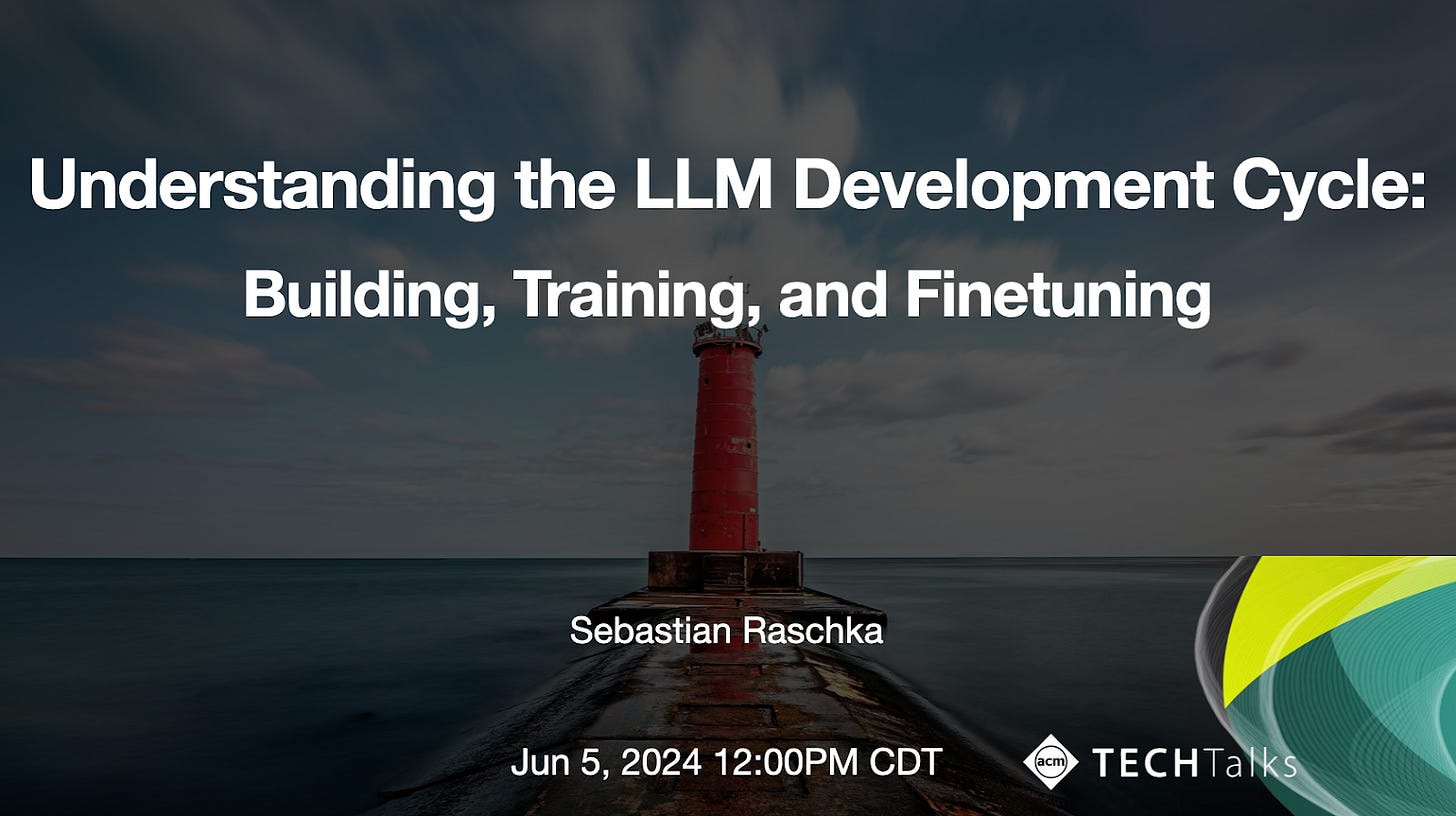
1. Instruction Tuning With Loss Over Instructions
One paper that caught my eye this month is Instruction Tuning With Loss Over Instructions.
In this paper, the authors question a widely accepted practice in instruction finetuning: masking the instruction when calculating the loss. But before we discuss the findings, let's begin with a general overview.
1.1 Instruction Masking During Instruction Finetuning
Instruction finetuning (or instruction tuning for short) is the task of improving the responses of a pretrained LLM to follow instructions ("Summarize this article," "Translate this sentence," etc.).
When instruction finetuning LLMs, it is common to mask out the instruction itself when calculating the loss. For instance, this is done by default in our LitGPT library, and I use it also in Chapter 7 of my Build a Large Language Model From Scratch book (however, I am now considering moving the masking to a reader exercise).
In other popular LLM libraries like Axolotl, this is also done automatically via the train_on_inputs: false
default setting in the config.yaml
. In Hugging Face, this is not done by default, but one can implement this through a DataCollatorForCompletionOnlyLM
dataset collator, as detailed in their documentation.
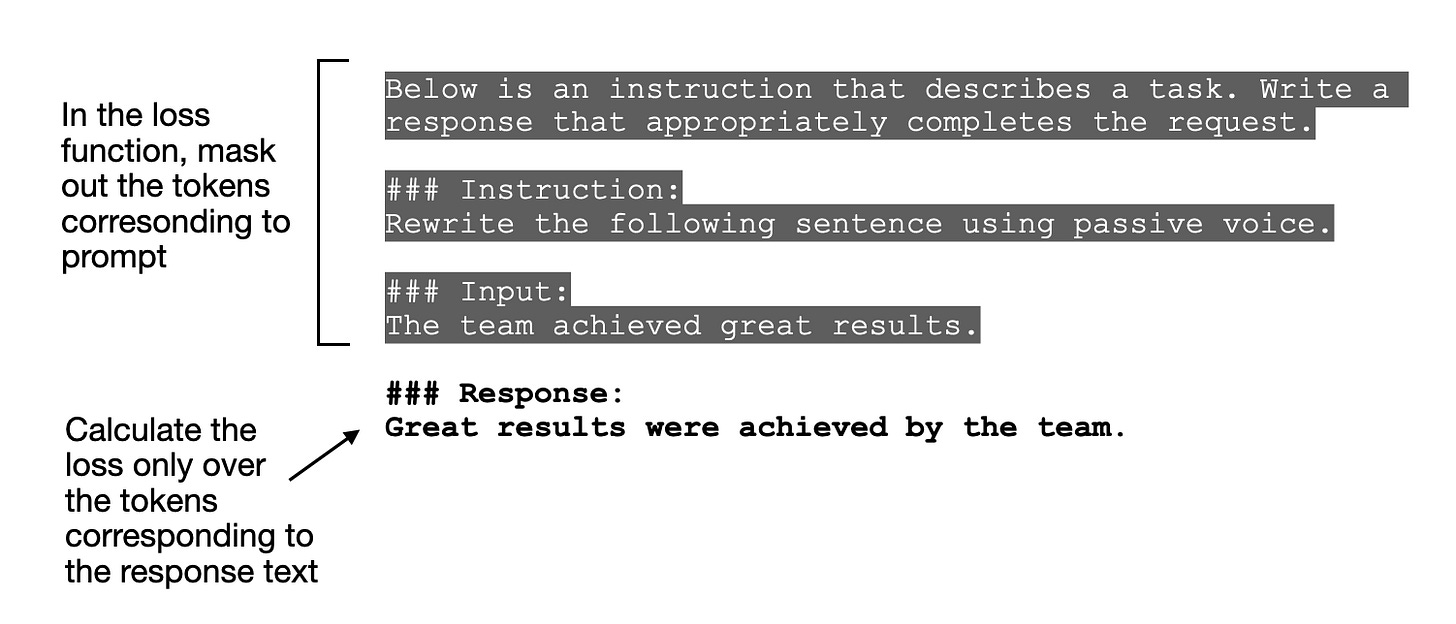
Masking the input prompts, as described above, is a routine task, and some papers may include comparisons with and without this masking. For example, the QLoRA paper included a comparison in its appendix, finding that masking performs better.
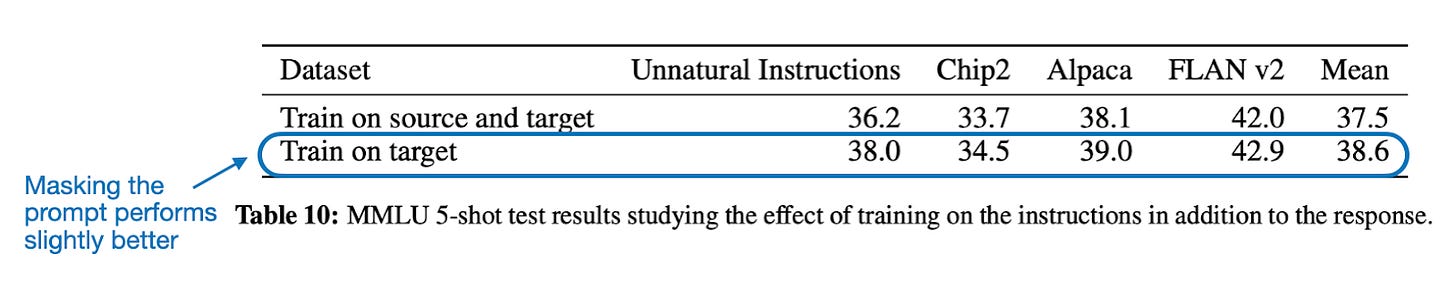
Note that MMLU is a benchmark focused on measuring the performance on multiple-choice questions, and the authors did not measure or study how it affects conversational performance when the finetuned model is used as a chatbot.
1.2 Instruction Modeling
After the brief introduction to the topic in the previous section, let's examine the Instruction Tuning With Loss Over Instructions paper. In this study, the authors systematically investigate the differences in LLM performance when instructions are masked versus unmasked.
Approach number 1 in the figure above is the default approach when implementing an LLM because it does not require any extra work or modification of the loss function. It's the method that the authors refer to in the paper as "instruction modeling." (In the paper, they additionally mask special prompt tokens like <|user|>
, <|assistant|>
, and <|system|>
that may occur in non-Alpaca prompt templates.)
Approach number 2 is currently the most common approach in practice, where everything except for the response is masked when computing the loss. In the paper, they refer to this approach as "instruction tuning" (the naming is a bit unfortunate in this context, because we are not "tuning" the instruction but excluding the instructions from the loss, compared to approach 1).
While drawing the figure above, I thought of an interesting third approach that is not explored in the paper: Masking prompt-specific boilerplate text. For instance, in Alpaca-style prompts, all examples begin with "Below is an instruction...". Compared to the actual instruction and input, this text is constant and could thus be excluded from the loss. This is not studied in the paper but might be an interesting additional experiment, which I am planning to add as another reader exercise (plus solution) to my LLMs from Scratch book.
It turns out that instruction modeling (that is, not masking the instructions) seems to outperform the masking approach, as shown in the figure below.
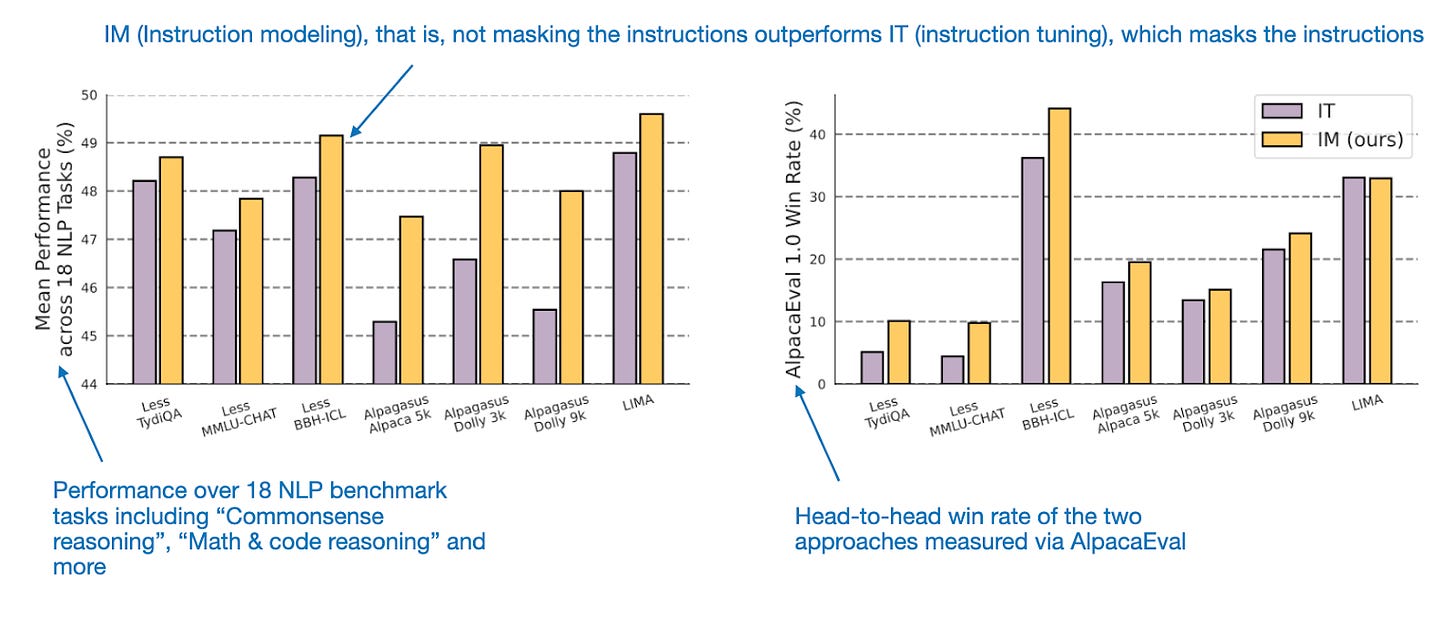
However, the performance of "instruction modeling" depends on (a) the ratio between the instruction and response length and (b) the size of the dataset (in terms of the number of training examples).
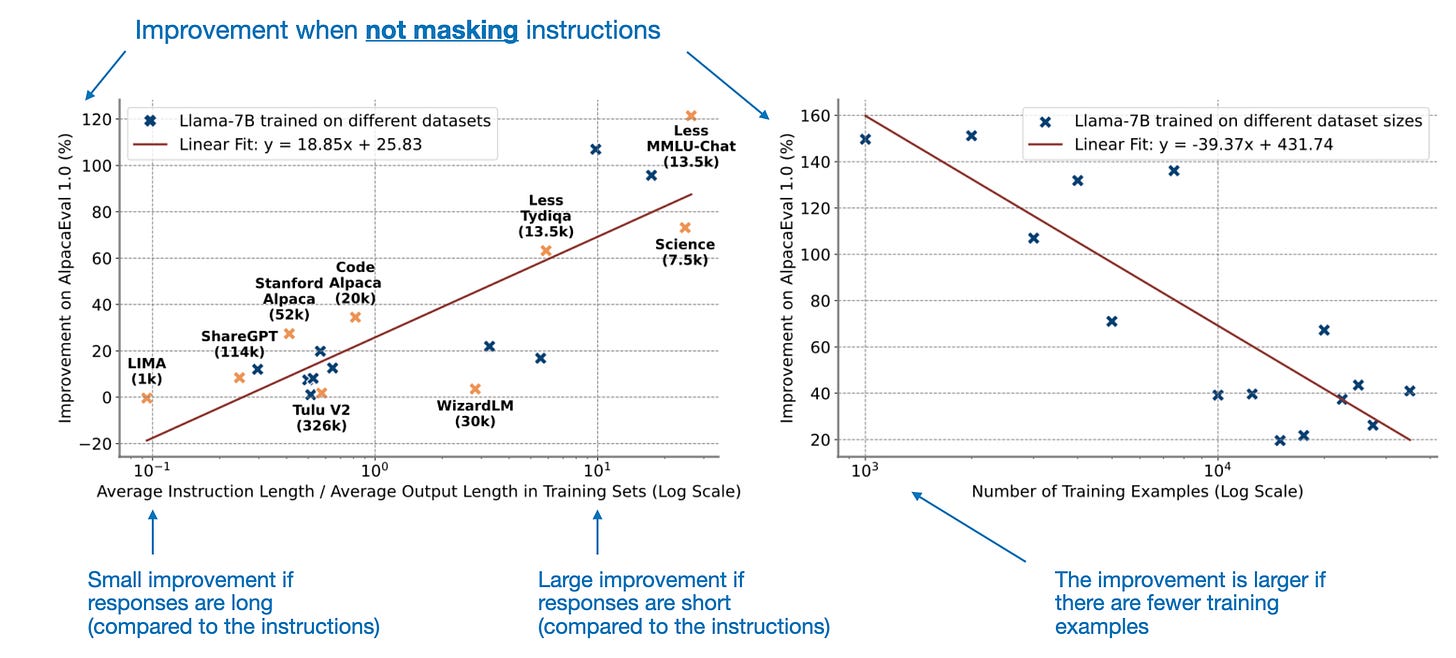
A plausible explanation for the dataset length and size dependence shown above is that if the responses are short and there are only a few training examples, it becomes easier for the model to memorize the responses, so including the instruction modeling, that is, modeling more of the model output in the loss, can help reduce overfitting.
1.3 Conclusion
In short, the authors found that going back to the basics, not masking the instructions, can benefit model performance.
It's positively surprising that the simpler approach of not masking the instruction (except for mask special prompt tokens like <|user|>, <|assistant|>, and <|system|>) performs better.
In my personal experience, when I tried both approaches in the past and didn't see a clear winner, I usually didn't experiment with masking much, though, and focused more on changing other settings if things didn't work well. In hindsight, it may make sense to consider experimenting with the (not) masking approach a bit more, as it seems to depend on the dataset size and length.
2. LoRA learns less and forgets less
LoRA Learns Less and Forgets Less is a comprehensive empirical study of low-rank adaptation (LoRA) for finetuning LLMs, comparing LoRA to full finetuning across two target domains: programming and mathematics. In addition to these domains, the comparison extends to two target tasks: instruction finetuning and continued pretraining.
If you are looking for a LoRA refresher before reading on, I recently covered in "Improving LoRA: Implementing Weight-Decomposed Low-Rank Adaptation (DoRA) from Scratch":
2.1 LoRA Learns Less
Based on the first set of results, LoRA learns significantly less than full finetuning, as shown in the figure below. This is expected, in my view, because updating fewer parameters limits learning capacity. Learning new knowledge generally requires more capacity than converting a pretrained base model into an instruction-following model.
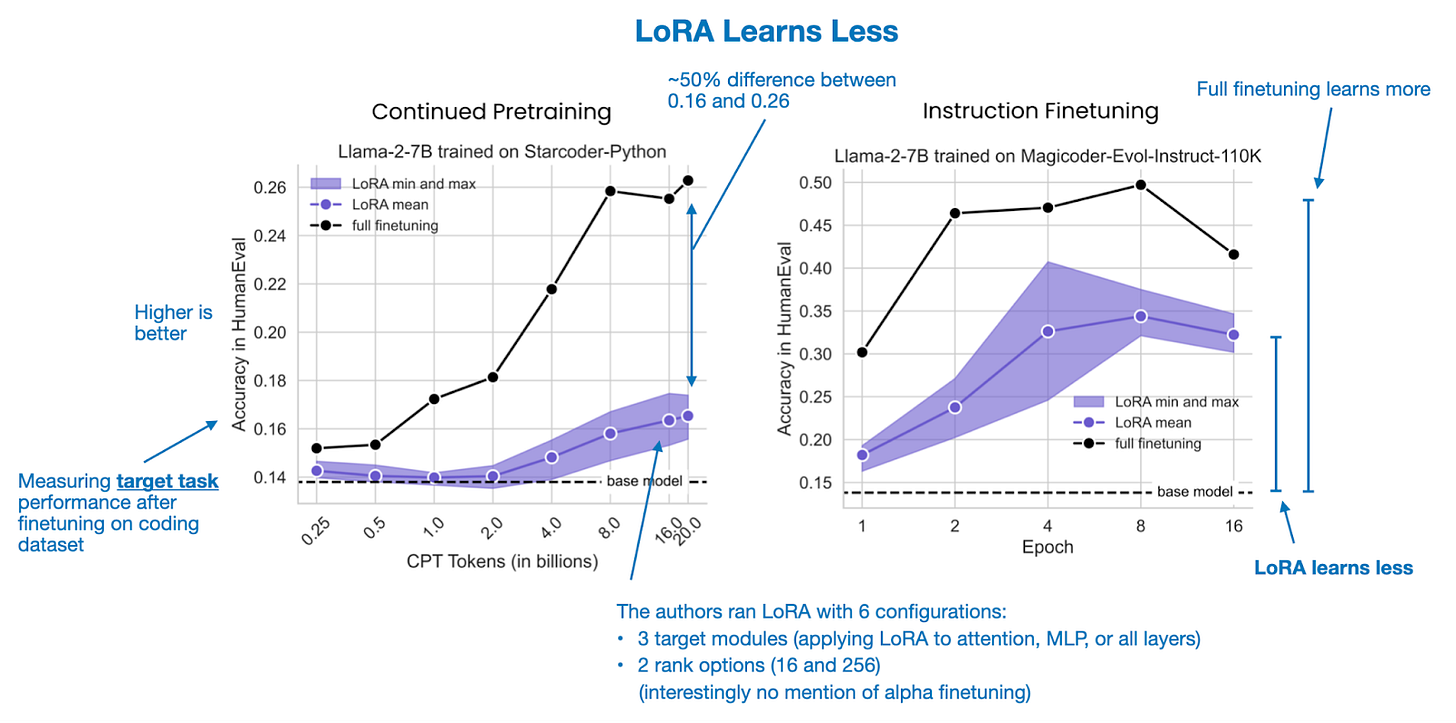
In the figure above, it is also interesting to note that the gap between LoRA and full finetuning is larger for continued pretraining than for instruction finetuning. This is consistent with the common intuition that pretraining primarily teaches an LLM new knowledge, whereas instruction finetuning primarily changes the behavior of an LLM.
Next, examining the same set of experiments in the domain of math instead of coding, we observe that the gap between full finetuning and LoRA shrinks, as illustrated in the figure below.
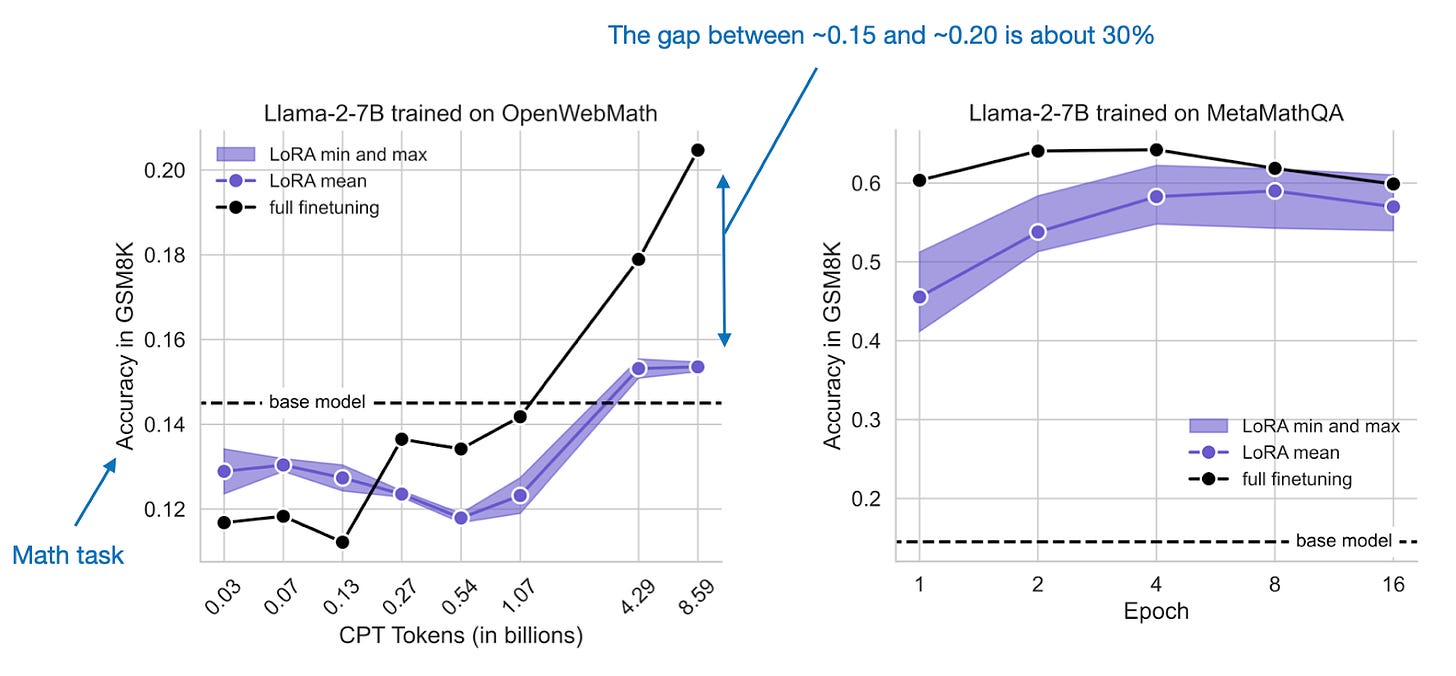
One could argue that solving math problems is more closely related to the source domain of the LLM than coding is. In other words, the LLM may have encountered more math problems during pretraining than programming tasks. Furthermore, math problems are usually described in words, whereas programming requires an entirely new set of terms.
As a takeaway, so far we can conclude that the further the new task deviates from the pretraining data, the more advantageous it is to use full finetuning over LoRA when it comes to acquiring new knowledge, such as through continued pretraining.
2.2 LoRA Forgets Less
Above, we examined how LoRA compares to full finetuning in terms of knowledge updates. The next set of experiments assesses these two approaches in terms of forgetting information after additional training, via continued pretraining and instruction finetuning. The difference to the previous results is that here they measure the performance on the original source tasks.
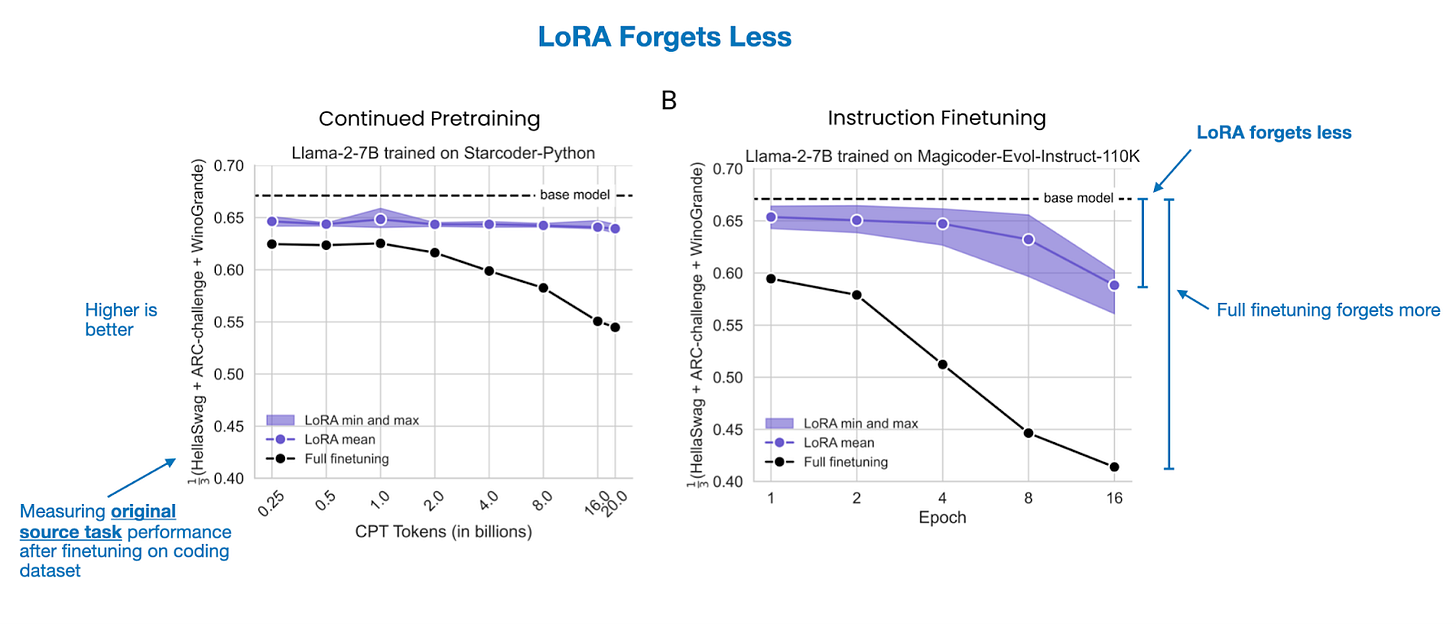
As we can see from the figure above, full finetuning forgets much more knowledge than LoRA when these methods are applied to datasets that are farther from the source domain (here, coding). The gaps are smaller for the math dataset, as shown below.
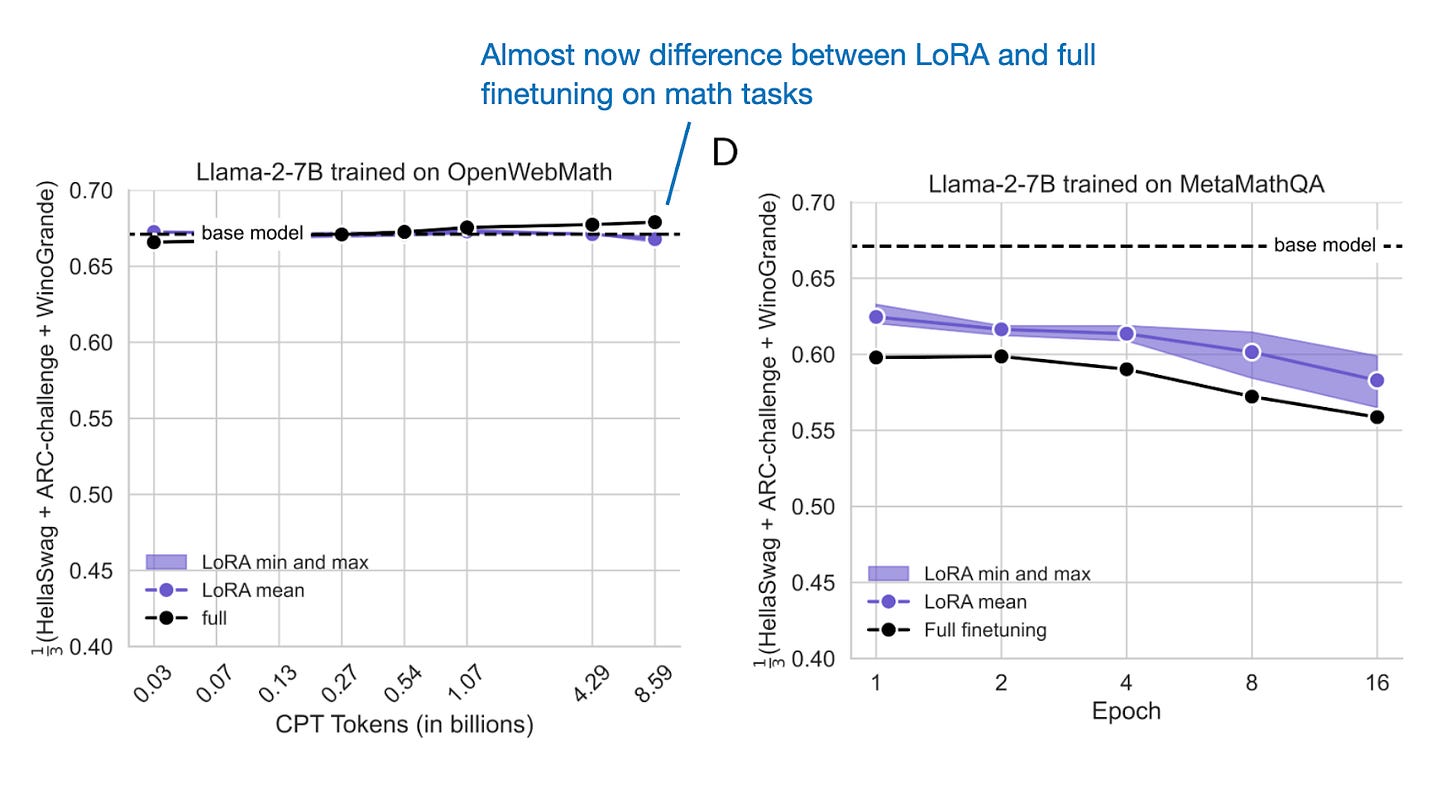
2.3 Conclusion
LoRA versus full finetuning? Perhaps as expected, it all boils down to a learning-forgetting trade-off. Full finetuning results in stronger performance in the new target domain, whereas LoRA maintains better performance in the original source domain *.
Intuitively, I suspect this is merely a side effect of LoRA changing fewer parameters in the model—the goal of LoRA, as its name suggests, is low-rank adaptation, which means not substantially modifying all model parameters.
Moreover, in practice, it often isn't a question of whether to use full finetuning or LoRA, as the latter might be the only feasible option due to its memory savings and lower storage footprint.
Nonetheless, it's very interesting to see this thoroughly laid out and analyzed in great experimental detail. (The experiments were conducted with 7B and 13B Llama 2 models).
* (One caveat that Mariano Kamp pointed out to me was that they did not update the embedding layers in the LoRA experiments, which is crucial when adapting a model to a new task.)
3. MoRA: High-Rank Updating for Parameter-Efficient Finetuning
It's always exciting when a new paper with a LoRA-like method for efficient LLM finetuning is published. In MoRA: High-Rank Updating for Parameter-Efficient Finetuning the authors take a related yet opposite approach to low-rank adaptation by replacing the LoRA adapters with a square matrix.
Also, Appendix E of my Build a Large Language Model From Scratch book implements LoRA from scratch for a GPT model trained to classify spam messages.
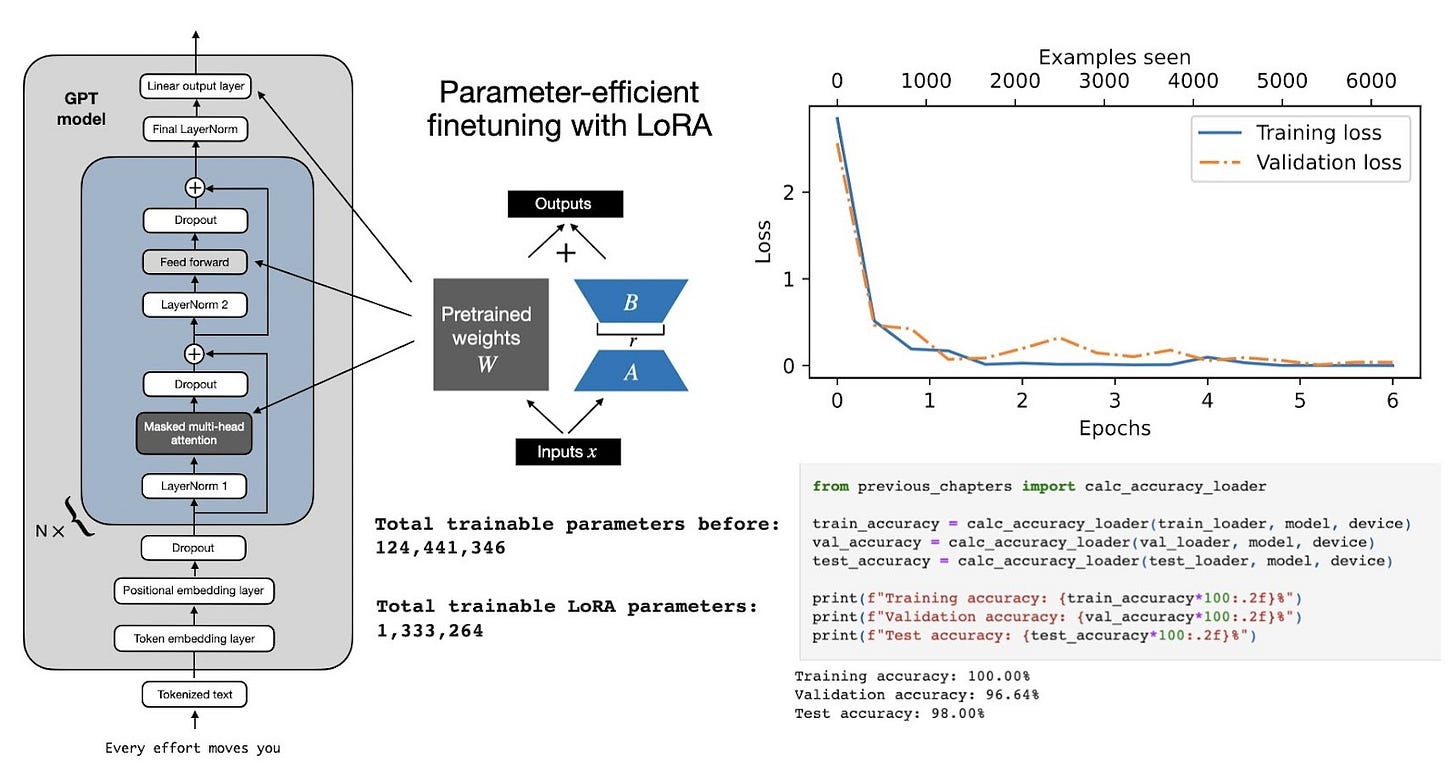
3.1 Low Vs High Ranks
As shown in the figure below, MoRA uses a small square matrix (M) instead of the two small LoRA matrices, A and B. We will return to discussing how this works in the next section.
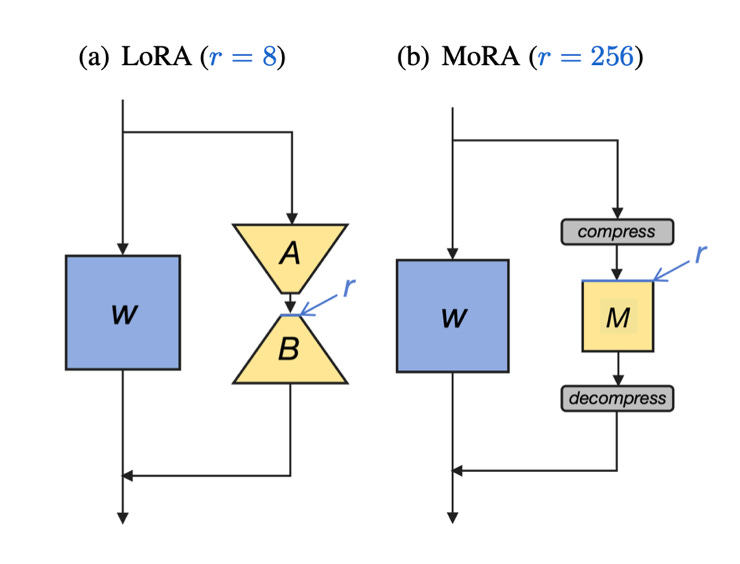
Why introduce another LoRA alternative, now with "high" ranks? The reason is that LoRA updates the original weights in a relatively limited way. In my view, this is by design, because we don't want to perturb or change the original model capabilities too much when we finetune a model with LoRA. However, while this low-rank updating is effective and sufficient for tasks like instruction finetuning, one downside of the low-rank updating is that it is relatively ineffective for incorporating new knowledge, such as via continued pretraining (also referred to as finetuning on regular text data, not instruction finetuning).
In the MoRA paper, the authors seek to develop a parameter-efficient finetuning method that can perform well for both instruction finetuning and absorbing new knowledge in continued pretraining.
Below is a side-by-side comparison between LoRA, MoRA, and regular full finetuning (FFT) based on a synthetic dataset that requires the LLM to memorize specific identifier codes.
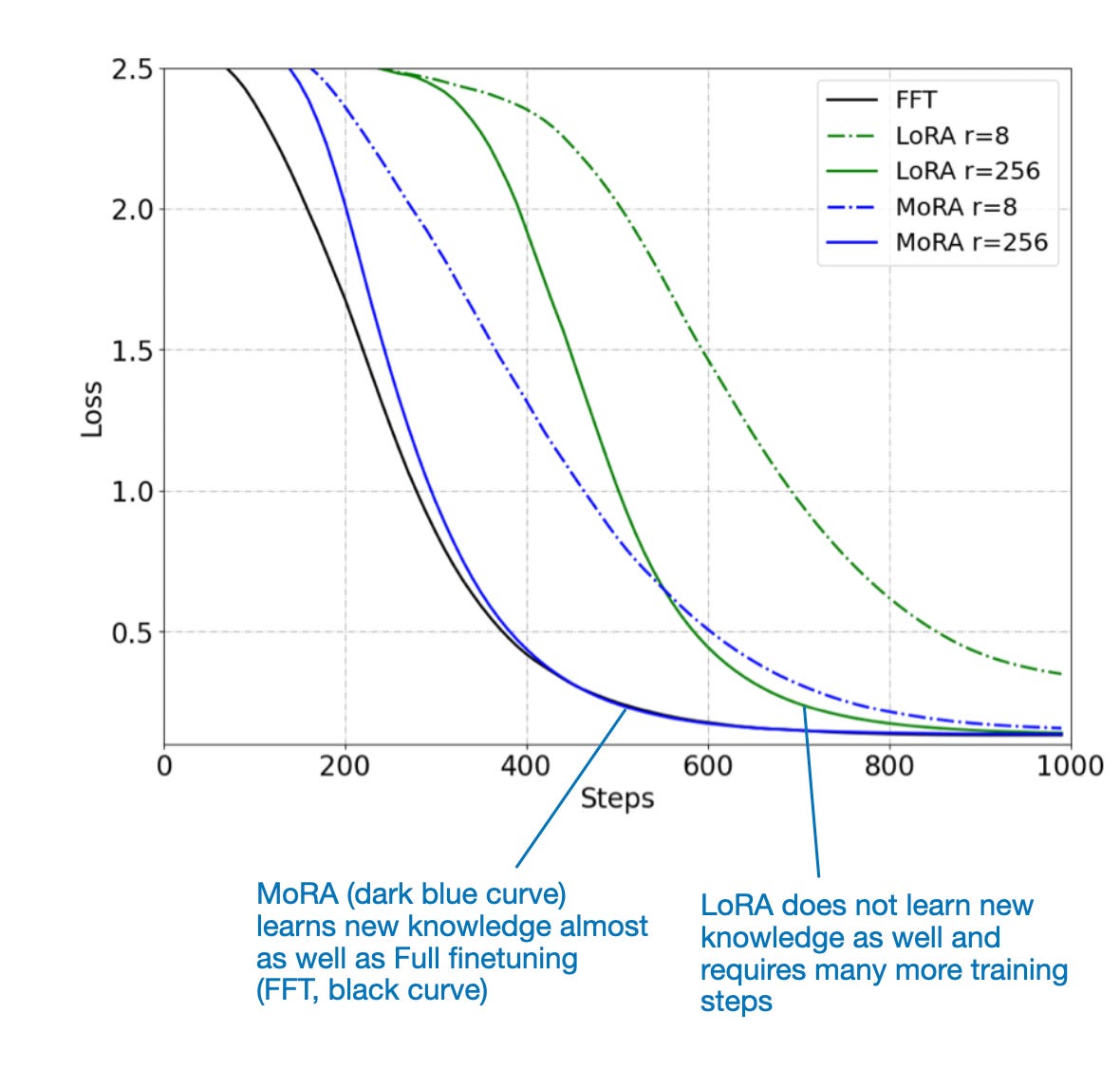
In the synthetic benchmark shown in the figure above, MoRA has similar knowledge-uptake (or memorization) capabilities as full fine-tuning. LoRA with high ranks (r=256) can eventually memorize the synthetic identifier codes as well, but it requires more steps. LoRA with a small rank (r=8) fails to memorize.
Note that memorization in LLMs is not necessarily a good thing (except for historical dates and facts, of course). However, the fact that LoRA does not tend to easily memorize can also be advantageous in reducing the tendency to overfit the training data. That being said, this benchmark primarily probes whether LoRA adds enough capacity to learn new knowledge. Think of it as similar to batch overfitting, a popular debugging technique where we try to get the model to overfit on a small portion of the training set to ensure that we have implemented the architecture correctly. (We will look at a benchmark on real data in the next section.)
3.2 MoRA in a Nutshell
So, how is MoRA implemented?
Here, the authors use a trainable square matrix that is applied to the original weights W instead of the matrices AB in LoRA. This square matrix is usually much smaller than the original weight matrix. In fact, the number of trainable parameters in LoRA and MoRA can even be the same.
For example, if the original weight layer has 4096 × 4096 = 16,777,216 parameters, LoRA with r=8 has 4096×8 + 8×4096 = 65,536 parameters. With MoRA, we can match the number of parameters with r=256, i.e., 256 × 256 = 65,536.
How do you apply this 256×256 matrix to the original 1024×1024 weight matrix? They define several non-parametric compression and decompression methods (the details are a bit out of scope for this post, but I tried to summarize them with PyTorch code in the figure).
Left: Visualization of the matrix dimensions for LoRA and DoRA when the total number of parameters is the same. Right: Example code illustrating the compression and decompression of the MoRA matrix.
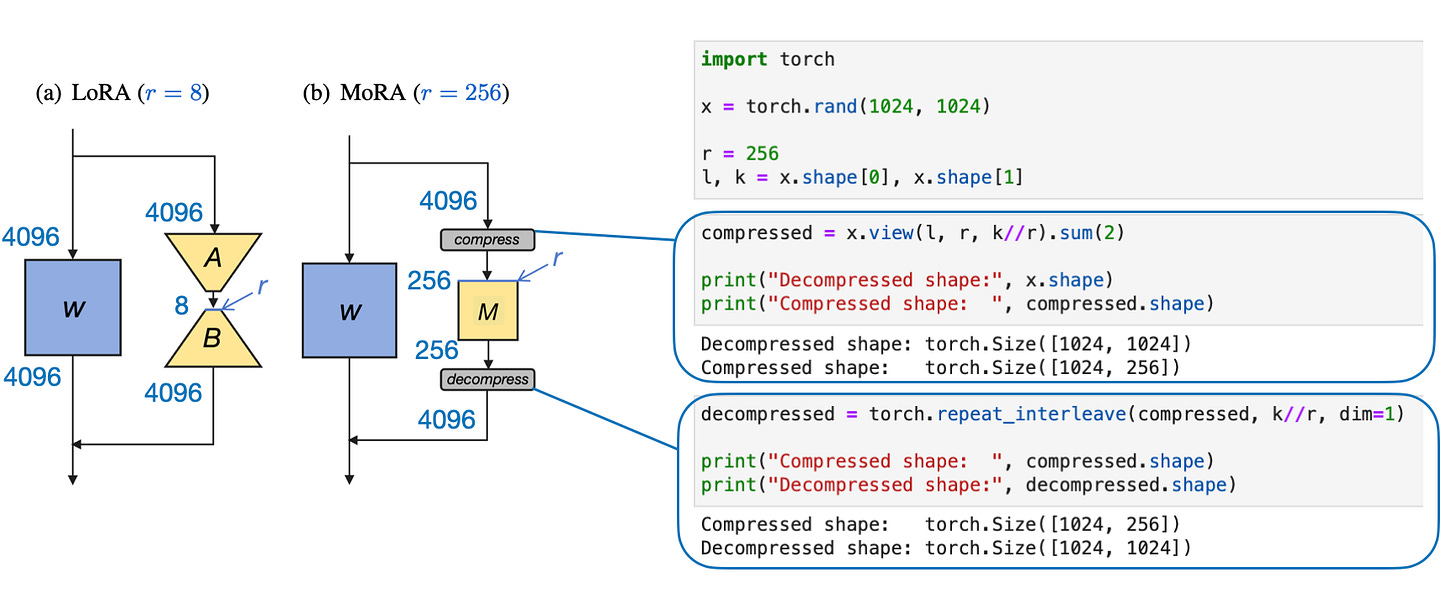
How do LoRA and MoRA compare?
Looking at real dataset benchmarks (see the table below), MoRA and LoRA are in relatively similar ballparks. However, in the case of continued pretraining on biomedical and finance data, MoRA outperforms all LoRA variants; only full fine-tuning (FFT) performs better. Additionally, an interesting observation is that LoRA ties with or outperforms DoRA, a LoRA variant I covered a few months ago in "Improving LoRA: Implementing Weight-Decomposed Low-Rank Adaptation (DoRA) from Scratch":
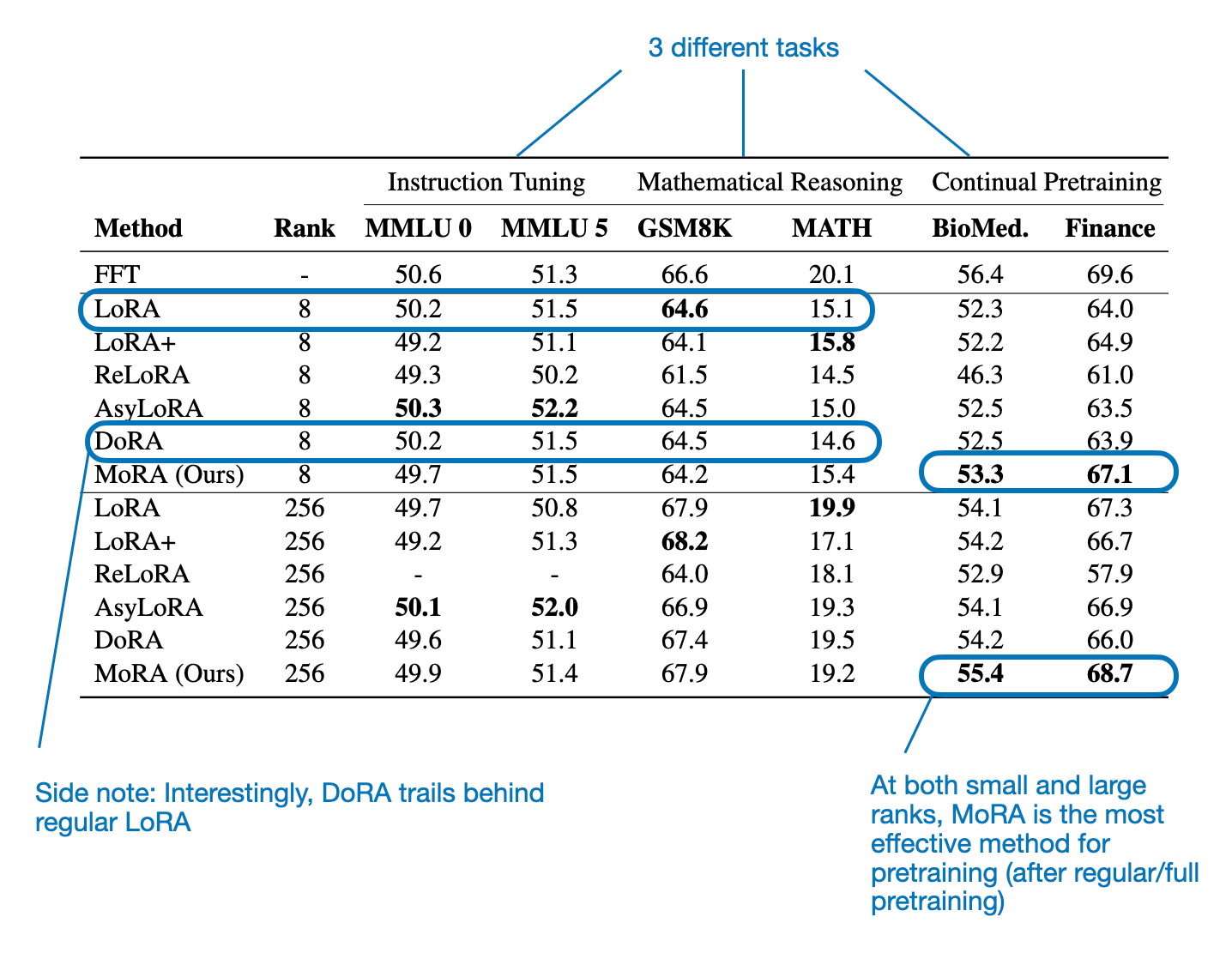
3.3 Conclusion
It turns out that the relatively simple MoRA method works surprisingly well. And it indeed slightly outperforms LoRA in continued pretraining. However, it does lag behind LoRA a bit in instruction fine-tuning and mathematical reasoning with small ranks. For me, the results are not compelling enough to replace LoRA with MoRA. Nonetheless, the paper presents an interesting method with an interesting set of experiments.
4. Other Interesting Research Papers In May
Below is a selection of other interesting papers I stumbled upon this month. Given the length of this list, I highlighted those 10 I found particularly interesting with an asterisk (*). However, please note that this list and its annotations are purely based on my interests and relevance to my own projects.
Contextual Position Encoding: Learning to Count What's Important by Golovneva, Wang, Weston, and Sukhbaatar (29 May), https://arxiv.org/abs/2405.18719
The research introduces Contextual Position Encoding (CoPE), a new position encoding method for LLMs that adapts to context, allowing for more abstract position-based attention.
LLaMA-NAS: Efficient Neural Architecture Search for Large Language Models by Sarah, Sridhar, Szanking, and Sundaresan (28 May), https://arxiv.org/abs/2405.18377
This study introduces a method using one-shot NAS and genetic algorithms to optimize the LLaMA2-7B model, achieving a 1.5x reduction in size and 1.3x speedup in throughput with minimal accuracy loss, outperforming traditional pruning and sparsification techniques.
VeLoRA: Memory Efficient Training using Rank-1 Sub-Token Projections by Miles, Reddy, Elezi, and Deng (28 May), https://arxiv.org/abs/2405.17991
This paper introduces a memory-efficient algorithm for training and finetuning LLMs that compresses intermediate activations without performance loss, divides tokens into sub-tokens, and projects them onto a fixed 1D subspace.
* gzip Predicts Data-dependent Scaling Laws by Pandey (26 May), https://arxiv.org/abs/2405.16684
This study challenges the notion that scaling laws for neural language models are data-agnostic by demonstrating that model performance scaling is sensitive to training data complexity, proposing a new data-dependent scaling law that correlates a model's compute-optimal resource allocation with the gzip-compressibility of the training data.
Offline Regularised Reinforcement Learning for Large Language Models Alignment by Yao, Wu, Yang, et al. (22 May), https://arxiv.org/abs/2405.13800
This paper introduces Direct Reward Optimization (DRO), a new framework for aligning large language models using more abundant single-trajectory datasets—consisting of a prompt, a response, and a user's feedback—eliminating the need for pairwise preference data like in Direct Preference Optimization (DPO).
Trans-LoRA: Towards Data-free Transferable Parameter Efficient Finetuning, by Wang, Ghosh, Cox, et al. (27 May), https://arxiv.org/abs/2405.17258
Trans-LoRA enables nearly data-free, lossless transfer of low-rank adapters (LoRA) between different base models using synthetic data.
Stacking Your Transformers: A Closer Look at Model Growth for Efficient LLM Pre-Training (26 May) by Du, Luo, Qiu, et al., https://arxiv.org/abs/2405.15319
This paper studies model growth methods, specifically a depthwise stacking operator called Gstack, in pretraining LLMs, to accelerate training and improves performance.
The Road Less Scheduled, Defazio, Yang, Mehta, et al. (24 May), https://arxiv.org/abs/2405.15682
The study introduces a schedule-free optimization approach that outperforms traditional learning rate schedules without requiring a predefined stopping time, all without adding extra hyper-parameters beyond those in standard momentum-based optimizers.
Instruction Tuning With Loss Over Instructions by Shi, Yang, Wu, et al. (23 May), https://arxiv.org/abs/2405.14394
This paper compares the performance of LLMs that are instruction finetuned with and without masking the instruction and finds that not masking tends to perform better.
SimPO: Simple Preference Optimization with a Reference-Free Reward by Meng, Xia, and Chen (23 May), https://arxiv.org/abs/2405.14734
Direct preference optimization (DPO) simplifies reinforcement learning with human feedback learning for LLMs, and this paper further simplifies DPO by averaging the log-probas and removing the need for a reference model.
AlignGPT: Multi-modal Large Language Models with Adaptive Alignment Capability by Fei Zhao, Taotian Pang, and Chunhui Li (23 May), https://arxiv.org/abs/2405.14129
AlignGPT is a new multimodal large language model that improves cross-modal alignment by differentiating the alignment capabilities of image-text pairs during pretraining and adaptively tuning these capabilities during instruction finetuning.
Dense Connector for MLLMs by Yao, Wu, Yang, et al. (22 May), https://arxiv.org/abs/2405.13800
This paper introduces the Dense Connector, a vision-language connector that improves Multimodal Large Language Models (MLLMs) by integrating multi-layer visual features.
Attention as an RNN by Feng, Tung, Hajimirsadeghi, et al. (22 May), https://arxiv.org/abs/2405.13956
This study reinterprets Transformers as a variant of RNNs and introduces Aaren, a new attention-based module that combines the parallel training capabilities of Transformers with the efficient, constant-memory updating of traditional RNNs.
* MoRA: High-Rank Updating for Parameter-Efficient Fine-Tuning by Jiang, Huang, Luo, et al. (20 May), https://arxiv.org/abs/2405.12130
This paper analyzes the limitations of low-rank updating in LLMs and introduces MoRA, a method using a square matrix for high-rank updating, which matches LoRA's parameter efficiency and outperforms it on memory-intensive tasks while maintaining comparable performance on others.
SLAB: Efficient Transformers with Simplified Linear Attention and Progressive Re-parameterized Batch Normalization by Guo, Chen, Tang, and Wang (19 May), https://arxiv.org/abs/2405.11582
This paper presents an approach to progressively replace LayerNorm with re-parameterized BatchNorm in transformer-based models, alongside a simplified linear attention (SLA) module.
Towards Modular LLMs by Building and Reusing a Library of LoRAs by Ostapenko, Su, Ponti, et al. (17 May), https://arxiv.org/abs/2405.11157
This study explores the reuse of trained adapters from a base LLMs for new tasks, introducing a method for building a library of adapters using model-based clustering (MBC) and a zero-shot routing mechanism to improve task generalization without retraining.
Chameleon: Mixed-Modal Early-Fusion Foundation Models by unknown authors at Meta AI (16 May), https://arxiv.org/abs/2405.09818
Chameleon is an early-fusion, token-based mixed-modal model that excels in processing and generating both images and text in any sequence.
Xmodel-VLM: A Simple Baseline for Multimodal Vision Language Model by Xu, Liu, He, et al. (15 May), https://arxiv.org/abs/2405.09215
This paper introduces Xmodel-VLM, a 1B-scale, efficient multimodal vision language model designed for deployment on consumer GPUs.
* LoRA Learns Less and Forgets Less by Biderman, Ortiz, Portes, et al. (15 May), https://arxiv.org/abs/2405.09673
This study compares Low-Rank Adaptation (LoRA), the parameter-efficient finetuning method, with full finetuning on large language models across programming and mathematics domains, finding that while LoRA often underperforms, it better preserves base model capabilities outside targeted domains and provides stronger regularization.
RLHF Workflow: From Reward Modeling to Online RLHF by Dong, Xiong, Pang, et al. (13 May), https://arxiv.org/abs/2405.07863
This report outlines an Online Iterative RLHF method for LLMs that uses proxy models to simulate human feedback, which outperforms offline methods and leading to the high-performing SFR-Iterative-DPO-LLaMA-3-8B-R model.
PHUDGE: Phi-3 as Scalable Judge by Deshwal and Chawla (12 May), https://arxiv.org/abs/2405.08029
This report introduces PHUDGE, a Phi3 model that surpasses ChatGPT's GPT 4 in speed and effectiveness for grading tasks.
Value Augmented Sampling for Language Model Alignment and Personalization by Han, Shenfeld, Srivastava, et al. (10 May), https://arxiv.org/abs/2405.06639
This study introduces Value Augmented Sampling (VAS), a new reward optimization framework that aligns LLMs efficiently without modifying their weights or co-training policy and value functions and enables adaptation of API-only LLMs like ChatGPT.
* Does Fine-Tuning LLMs on New Knowledge Encourage Hallucinations? by Gekhman, Yona, Aharoni, et al. (9 May), https://arxiv.org/abs/2405.05904
This study explores the impact of introducing new factual information during the supervised finetuning of large language models, finding that while these models struggle to incorporate new facts and learn them slower than familiar information, the eventual learning of these facts linearly increases the model’s tendency to generate factually incorrect responses.
* Fishing for Magikarp: Automatically Detecting Under-trained Tokens in Large Language Models by Land and Bartolo (8 May), https://arxiv.org/abs/2405.05417
This paper presents a thorough analysis of tokenizer issues in LLMs and suggests a method to automatically detect 'glitch tokens'—tokens present in the tokenizer but nearly or entirely absent in training data.
* DeepSeek-V2: A Strong, Economical, and Efficient Mixture-of-Experts Language Model by Liu, Feng, Wang, et al. (8 May), https://arxiv.org/abs/2405.04434
DeepSeek-V2 is a 236B parameter Mixture-of-Experts language model (it utilizes only 21B activated parameters per token) that introduces Multi-head Latent Attention (MLA), which is designed to compress the Key-Value (KV) cache into a latent vector, which improves inference efficiency and reduces memory requirements.
You Only Cache Once: Decoder-Decoder Architectures for Language Models by Sun, Dong, Zhu, et al. (8 May), https://arxiv.org/abs/2405.05254
The study introduces YOCO, a decoder-decoder architecture for large language models that features a self-decoder for encoding global key-value caches used by a cross-decoder, substantially reducing GPU memory requirements and enhancing prefill performance.
* xLSTM: Extended Long Short-Term Memory by Beck, Poeppel, Spanring, et al. (7 May), https://arxiv.org/abs/2405.04517
This study explores the potential of scaling LSTMs to billions of parameters with new modifications like exponential gating and enhanced memory structures, integrating these into xLSTM architectures that perform comparably to state-of-the-art transformer- and state space- based LLMs.
vAttention: Dynamic Memory Management for Serving LLMs without PagedAttention by Prabhu, Nayak, Mohan et al. (7 May), https://arxiv.org/abs/2405.04437
This paper introduces vAttention, a method for managing GPU memory in LLMs that maintains KV-cache in contiguous virtual memory and uses existing low-level system support for demand paging to dynamically allocate physical memory.
* Is Flash Attention Stable? by Golden, Hsia, Sun, et al. (5 May), https://arxiv.org/abs/2405.02803
This study develops a framework to analyze the effects of numeric deviation on large-scale machine learning model training, finding that the widely-used Flash Attention optimization experiences significant numeric deviations, though these are less impactful than those from low-precision training
* What Matters When Building Vision-Language Models? by Laurencon, Tronchon, Cord, and Sanh (3 May), https://arxiv.org/abs/2405.02246
This paper highlights the lack of justification for critical design choices in vision-language models (VLMs) and presents Idefics2, a new 8-billion parameter VLM that achieves state-of-the-art performance by rigorously evaluating different architectures, data, and training methods, with its models and datasets made publicly available.
Prometheus 2: An Open Source Language Model Specialized in Evaluating Other Language Models by (2 May), https://arxiv.org/abs/2405.01535
This paper introduces Prometheus 2, an open-source evaluator LLM that addresses the limitations of previous models by closely aligning with human and GPT-4 judgments and offering flexibility in assessment formats and criteria.
* A Careful Examination of Large Language Model Performance on Grade School Arithmetic by Zhang, Da, Lee, et al. (1 May), https://arxiv.org/abs/2405.00332
This study introduces GSM1k, a new benchmark mirroring the established GSM8k, to test LLMs and finds that performance drops significantly, suggesting that previous successes may stem more from memorizing training data rather than genuine mathematical reasoning. (Note: GSM8k is a benchmark designed to evaluate the mathematical reasoning abilities of large language models by presenting problems typical of grade school level complexity.)
Self-Play Preference Optimization for Language Model Alignment by Wu, Sun, Yuan, et al. (1 May), https://arxiv.org/abs/2405.00675
This paper introduces the Self-play Probabilistic Preference Optimization (SPPO) method for language model alignment, which uses a self-play framework to approximate Nash equilibrium through iterative policy updates.
Is Bigger Edit Batch Size Always Better? An Empirical Study on Model Editing with Llama-3 by Yoon, Gupta, and Anumanchipalli (1 May), https://arxiv.org/abs/2405.00664
This study examines the effectiveness of model editing techniques on Llama-3 by testing sequential, batch, and a novel sequential-batch editing across various layers, concluding that smaller, sequential edits perform better than larger batch edits in improving model performance. (Note: Edit batch size refers to the number of changes made to a model’s parameters simultaneously in one round of editing.)
This magazine is a personal passion project that does not offer direct compensation. However, for those who wish to support me, please consider purchasing a copy of one of my books. If you find them insightful and beneficial, please feel free to recommend them to your friends and colleagues. (Sharing your feedback with others via a book review on Amazon helps a lot, too!)
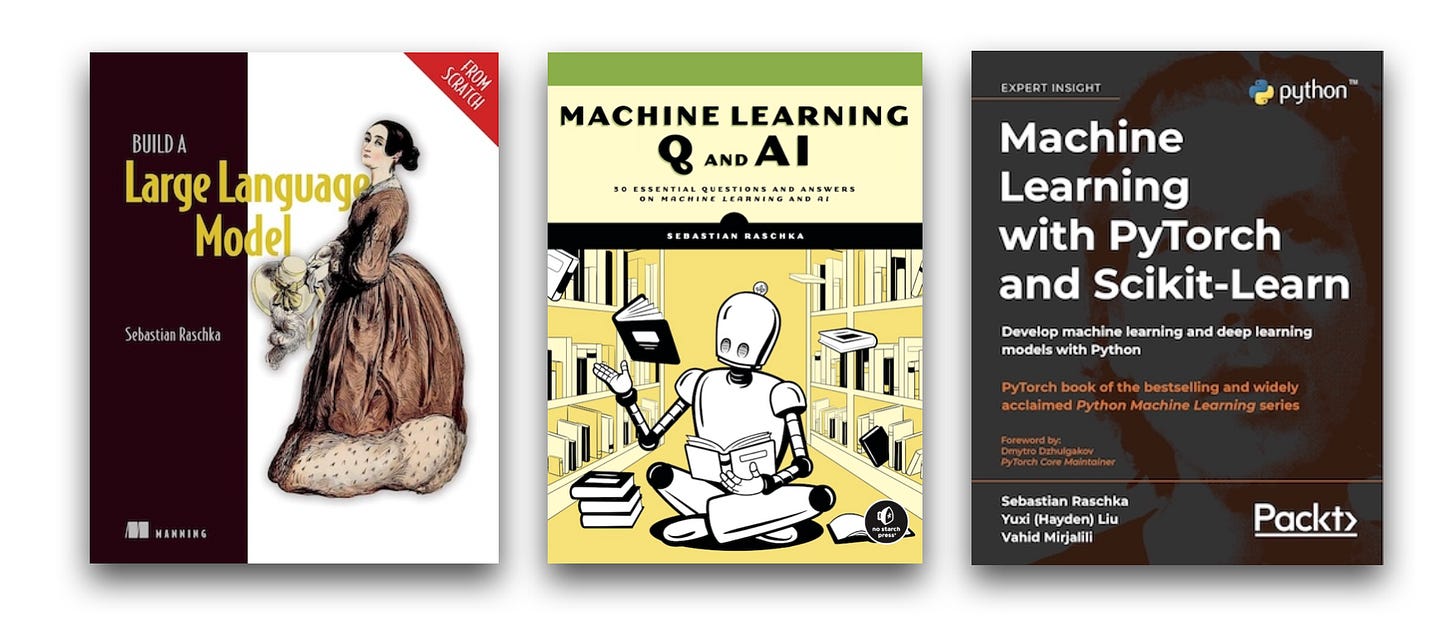
Your support means a great deal! Thank you!