This post is co-written with Bar Fingerman from Bria.
We are thrilled to announce that Bria 2.3, 2.2 HD, and 2.3 Fast text-to-image foundation models (FMs) from Bria AI are now available in Amazon SageMaker JumpStart. Bria models are trained exclusively on commercial-grade licensed data, providing high standards of safety and compliance with full legal indemnity.
These advanced models from Bria AI generate high-quality and contextually relevant visual content that is ready to use in marketing, design, and image generation use cases across industries from ecommerce, media and entertainment, and gaming to consumer-packaged goods and retail.
In this post, we discuss Bria’s family of models, explain the Amazon SageMaker platform, and walk through how to discover, deploy, and run inference on a Bria 2.3 model using SageMaker JumpStart.
Overview of Bria 2.3, Bria 2.2 HD, and Bria 2.3 Fast
Bria AI offers a family of high-quality visual content models. These advanced models represent the cutting edge of generative AI technology for image creation:
- Bria 2.3 – The core model delivers high-quality visual content with exceptional photorealism and detail, capable of generating stunning images with complex concepts in various art styles, including photorealism. Bria 2.2 HD – Optimized for high-definition, Bria 2.2 HD offers high-definition visual content that meets the demanding needs of high-resolution applications, making sure every detail is crisp and clear. Bria 2.3 Fast – Optimized for speed, Bria 2.3 Fast generates high-quality visuals at a faster rate, perfect for applications requiring quick turnaround times without compromising on quality. Using the model on SageMaker g5 instance types gives fast latency and throughput (compared to Bria 2.3 and Bria 2.2 HD), and the p4d instance type provides twice the latency from the g5 instance.
Overview of SageMaker JumpStart
With SageMaker JumpStart, you can choose from a broad selection of publicly available FMs. ML practitioners can deploy FMs to dedicated SageMaker instances from a network-isolated environment and customize models using SageMaker for model training and deployment. You can now discover and deploy Bria models in Amazon SageMaker Studio or programmatically through the SageMaker Python SDK. Doing so enables you to derive model performance and machine learning operations (MLOps) controls with SageMaker features such as Amazon SageMaker Pipelines, Amazon SageMaker Debugger, or container logs.
The model is deployed in an AWS secure environment and under your virtual private cloud (VPC) controls, helping provide data security. Bria models are available today for deployment and inferencing in SageMaker Studio in 22 AWS Regions where SageMaker JumpStart is available. Bria models will require g5 and p4 instances.
Prerequisites
To try out the Bria models using SageMaker JumpStart, you need the following prerequisites:
- An AWS account that will contain all your AWS resources. An AWS Identity and Access Management (IAM) role to access SageMaker. To learn more about how IAM works with SageMaker, refer to Identity and Access Management for Amazon SageMaker. Access to AWS Marketplace. Access to SageMaker Studio, a SageMaker notebook instance, or an integrated development environment (IDE) such as PyCharm or Visual Studio Code. We recommend using SageMaker Studio for straightforward deployment and inference.
Discover Bria models in SageMaker JumpStart
You can access the FMs through SageMaker JumpStart in the SageMaker Studio UI and the SageMaker Python SDK. In this section, we show how to discover the models in SageMaker Studio.
SageMaker Studio is an IDE that provides a single web-based visual interface where you can access purpose-built tools to perform all ML development steps, from preparing data to building, training, and deploying your ML models. For more details on how to get started and set up SageMaker Studio, refer to Amazon SageMaker Studio.
In SageMaker Studio, you can access SageMaker JumpStart by choosing JumpStart in the navigation pane or by choosing JumpStart on the Home page.
On the SageMaker JumpStart landing page, you can find pre-trained models from popular model hubs. You can search for Bria, and the search results will list all the Bria model variants available. For this post, we use the Bria 2.3 Commercial Text-to-image model.
You can choose the model card to view details about the model such as license, data used to train, and how to use the model. You also have two options, Deploy and Preview notebooks, to deploy the model and create an endpoint.
Subscribe to Bria models in AWS Marketplace
When you choose Deploy, if the model wasn’t already subscribed, you first have to subscribe before you can deploy the model. We demonstrate the subscription process for the Bria 2.3 Commercial Text-to-image model. You can repeat the same steps for subscribing to other Bria models.
After you choose Subscribe, you’re redirected to the model overview page, where you can read the model details, pricing, usage, and other information. Choose Continue to Subscribe and accept the offer on the following page to complete the subscription.
Configure and deploy Bria models using AWS Marketplace
The configuration page gives three different launch methods to choose from. For this post, we showcase how you can use SageMaker console:
- For Available launch method, select SageMaker console. For Region, choose your preferred Region. Choose View in Amazon SageMaker.
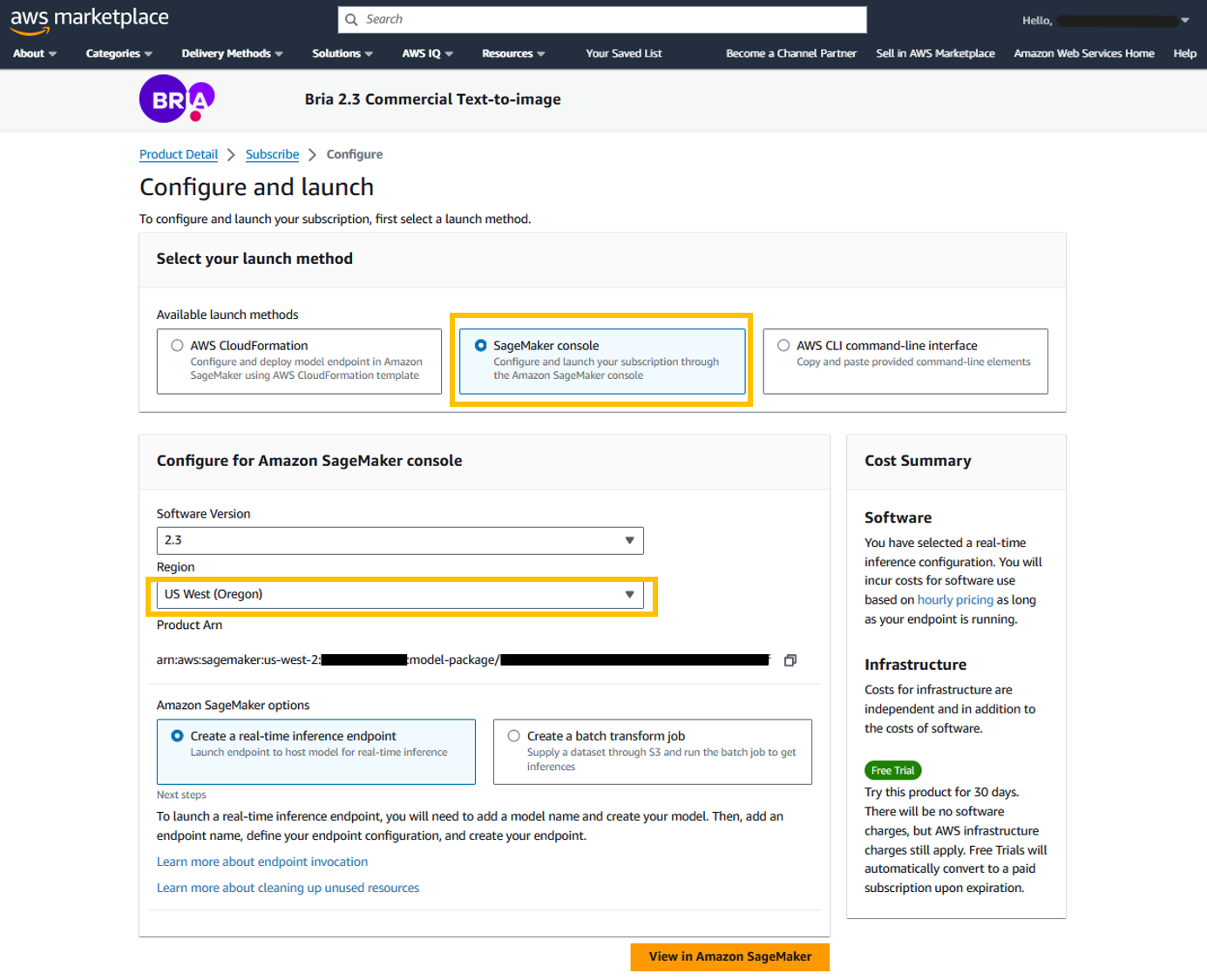
Model-Bria-v2-3
). For IAM role, choose an existing IAM role or create a new role that has the SageMaker full access IAM policy attached. Choose Next.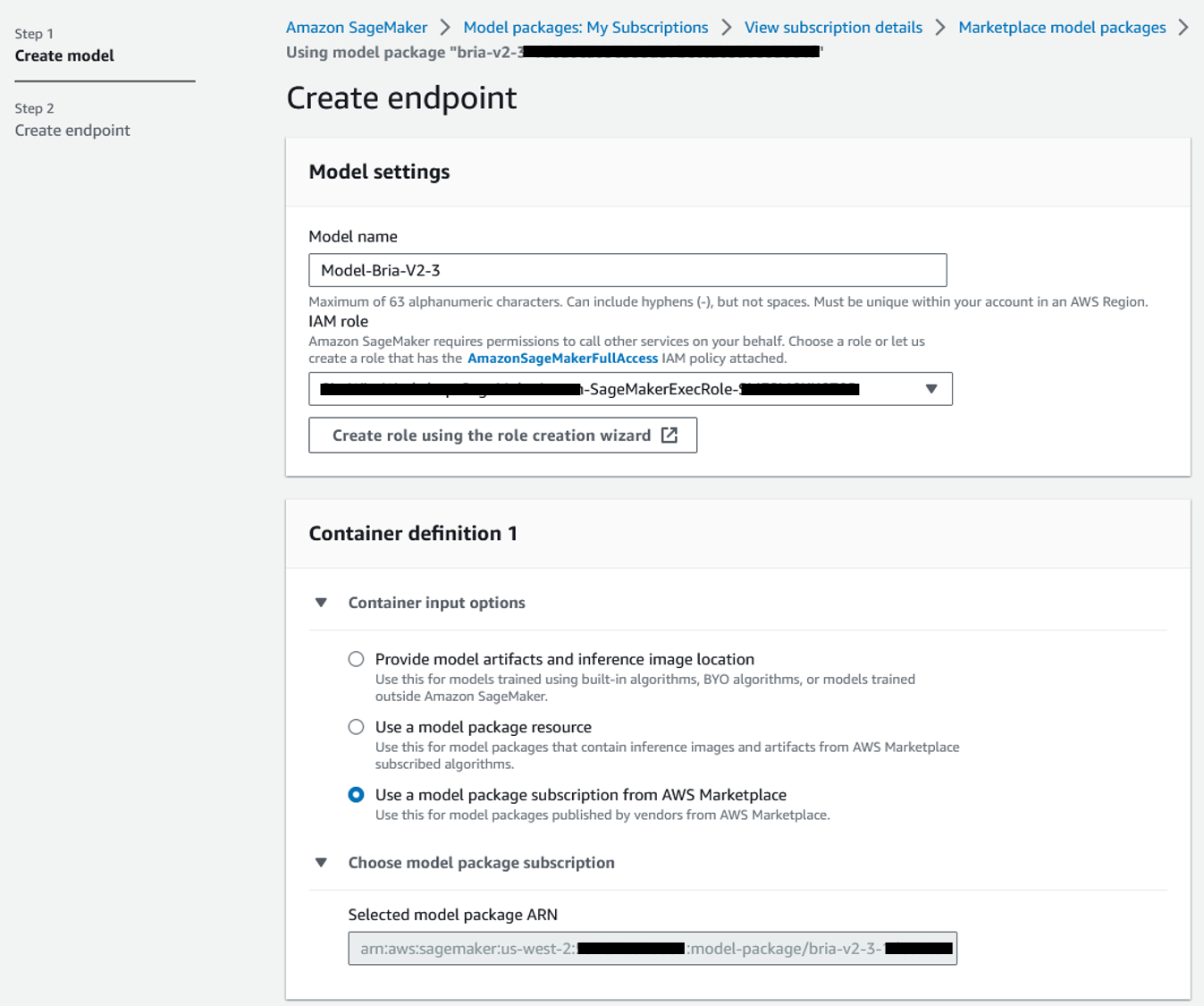
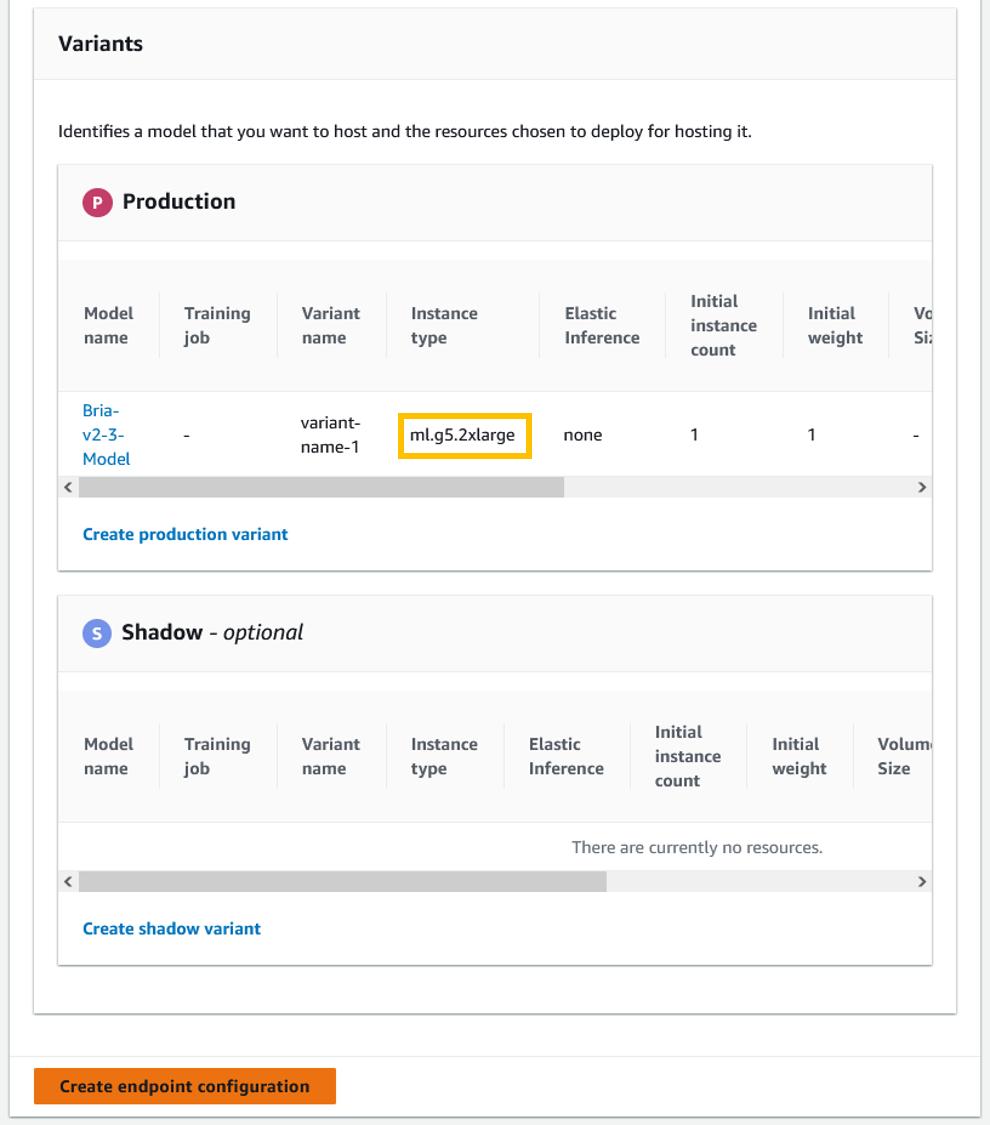
A success message should appear after the endpoint configuration is successfully created. Choose Next to create an endpoint.
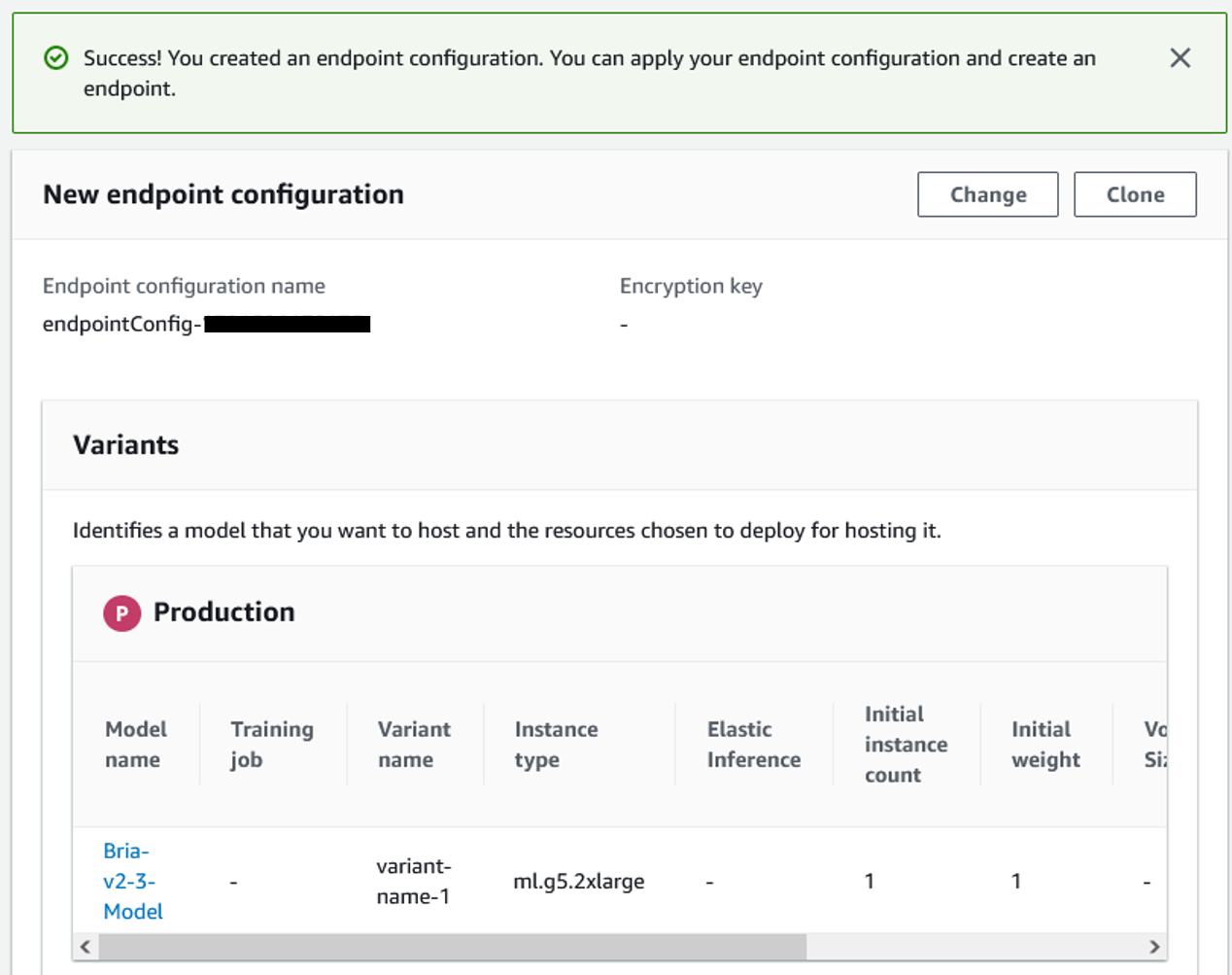
Endpoint-Bria-v2-3-Model
) and choose Submit.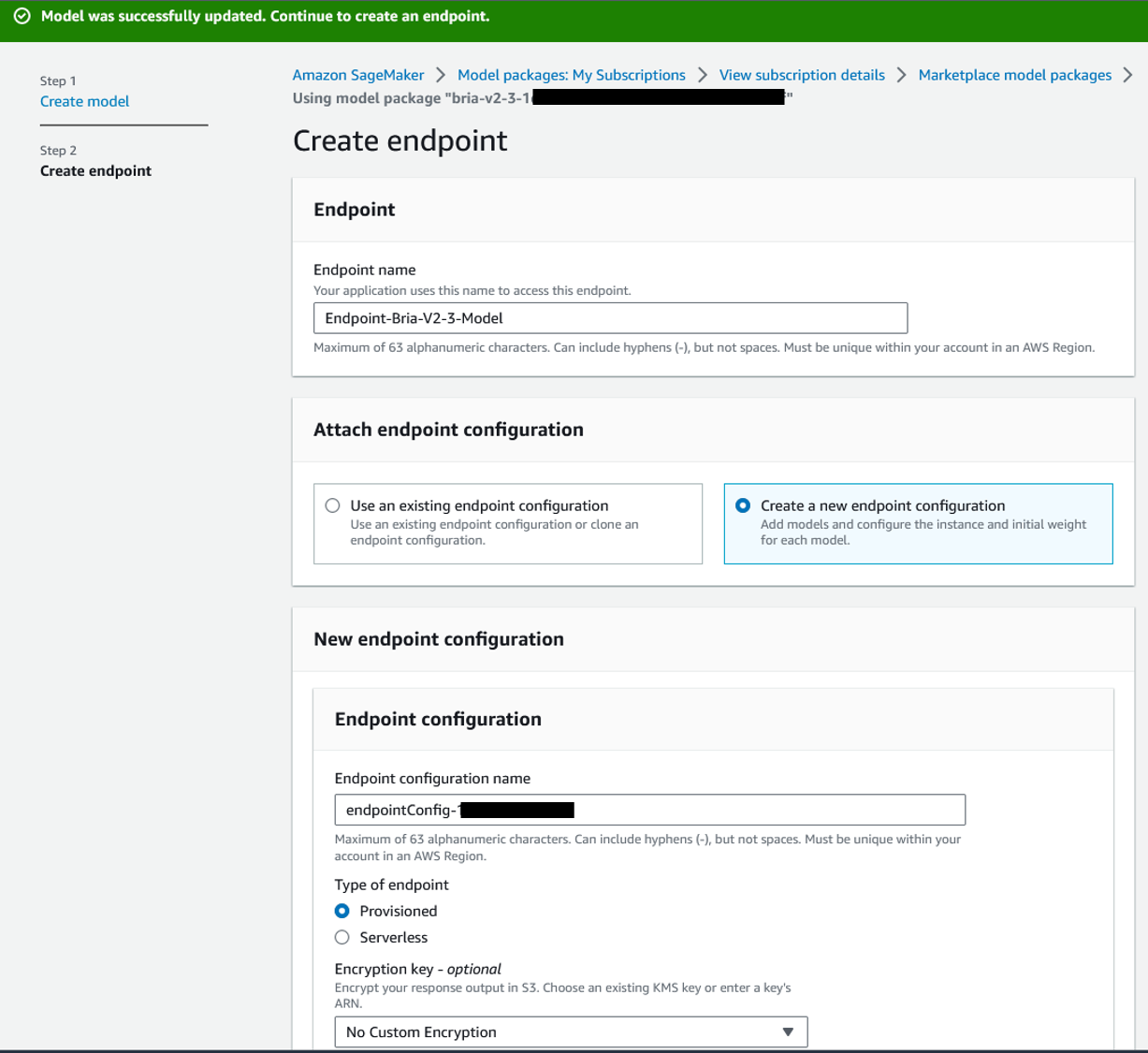
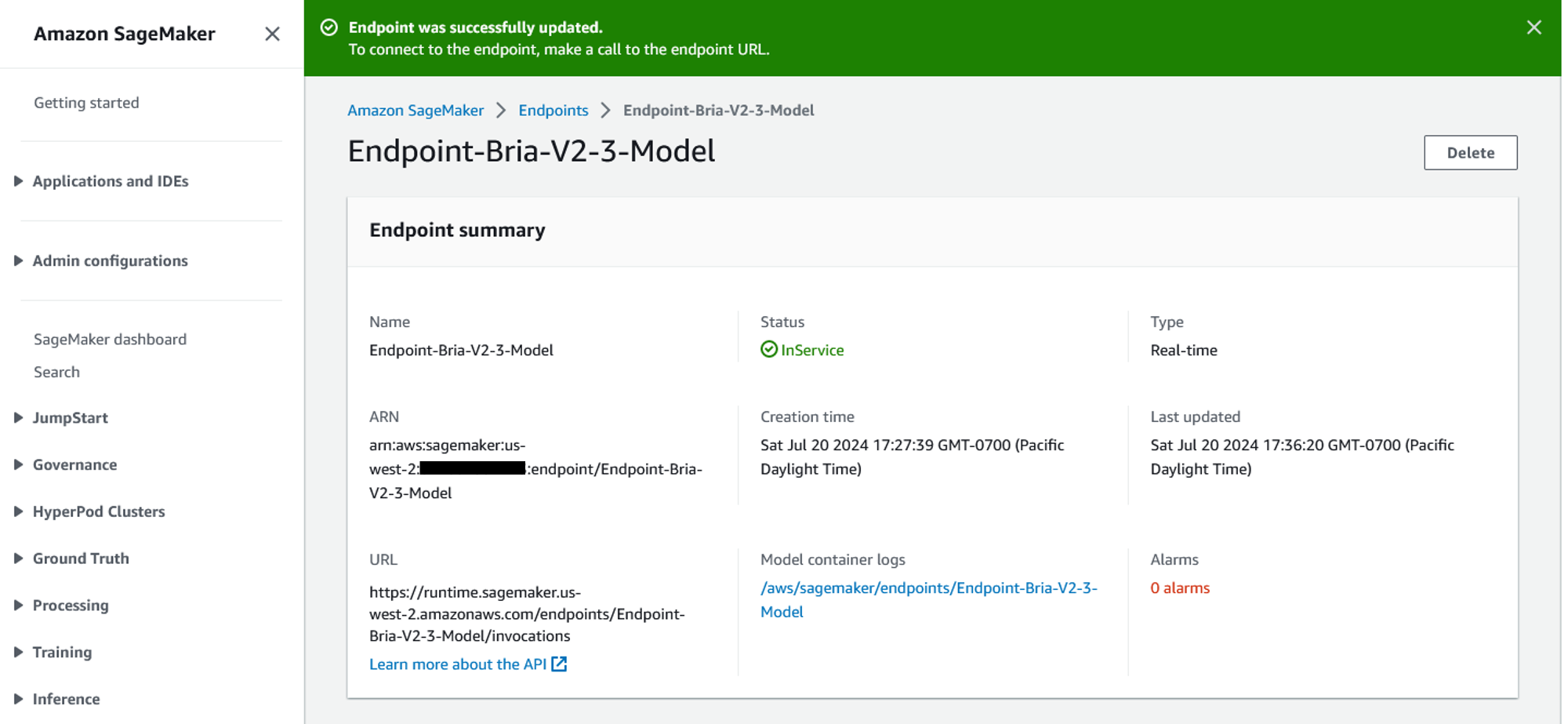
Configure and deploy Bria models using SageMaker JumpStart
If the Bria models are already subscribed in AWS Marketplace, you can choose Deploy in the model card page to configure the endpoint.
On the endpoint configuration page, SageMaker pre-populates the endpoint name, recommended instance type, instance count, and other details for you. You can modify them based on your requirements and then choose Deploy to create an endpoint.
After you successfully create the endpoint, the status will show as In service.
Run inference in SageMaker Studio
You can test the endpoint by passing a sample inference request payload in SageMaker Studio, or you can use SageMaker notebook. In this section, we demonstrate using SageMaker Studio:
- In SageMaker Studio, in the navigation pane, choose Endpoints under Deployments. Choose the Bria endpoint you just created.
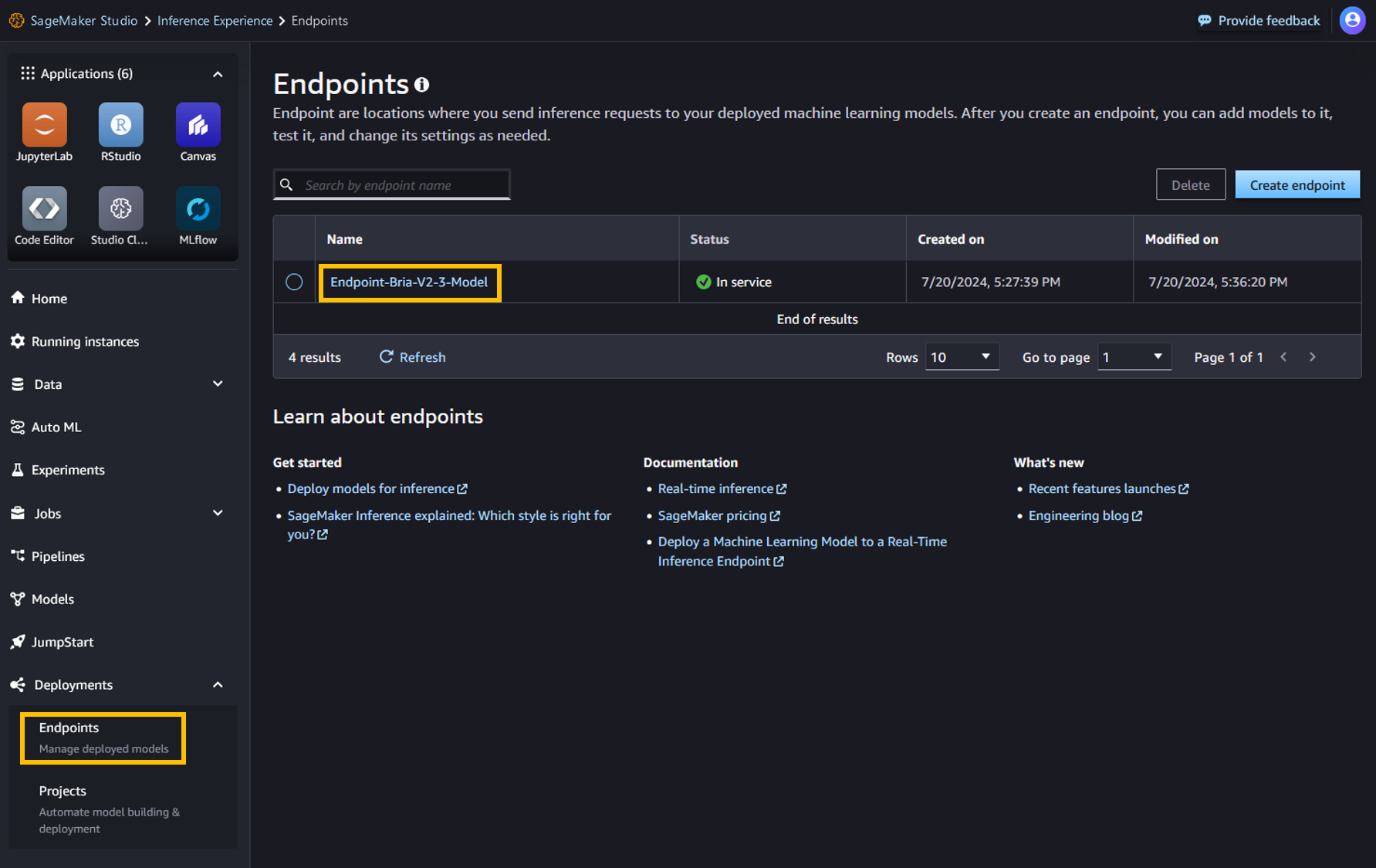
You can see the response on the same page, as shown in the following screenshot.
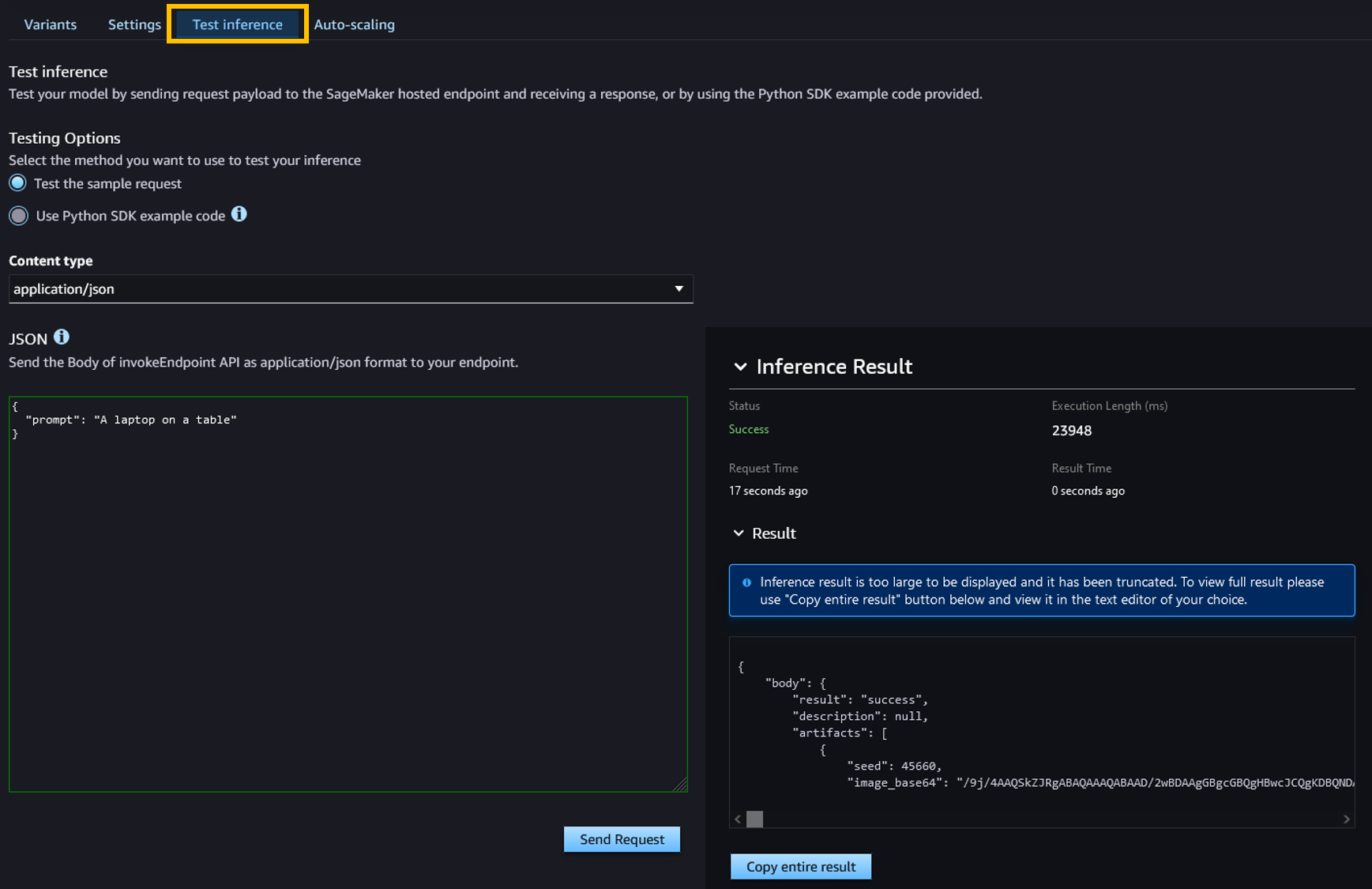
Text-to-image generation using a SageMaker notebook
You can also use a SageMaker notebook to run inference against the deployed endpoint using the SageMaker Python SDK.
The following code initiates the endpoint you created using SageMaker JumpStart:
The model responses are in base64 encoded format. The following function helps decode the base64 encoded image and displays it as an image:
The following is a sample payload with a text prompt to generate an image using the Bria model:
Example prompts
You can interact with the Bria 2.3 text-to-image model like any standard image generation model, where the model processes an input sequence and outputs response. In this section, we provide some example prompts and sample output.
We use the following prompts:
- Photography, dynamic, in the city, professional mail skateboarder, sunglasses, teal and orange hue Young woman with flowing curly hair stands on a subway platform, illuminated by the vibrant lights of a speeding train, purple and cyan colors Close up of vibrant blue and green parrot perched on a wooden branch inside a cozy, well-lit room Light speed motion with blue and purple neon colors and building in the background
The model generates the following images.
The following is an example prompt for generating an image using the preceding text prompt:
Clean up
After you’re done running the notebook, delete all resources that you created in the process so your billing is stopped. Use the following code:
Conclusion
With the availability of Bria 2.3, 2.2 HD, and 2.3 Fast in SageMaker JumpStart and AWS Marketplace, enterprises can now use advanced generative AI capabilities to enhance their visual content creation processes. These models provide a balance of quality, speed, and compliance, making them an invaluable asset for any organization looking to stay ahead in the competitive landscape.
Bria’s commitment to responsible AI and the robust security framework of SageMaker provide enterprises with the full package for data privacy, regulatory compliance, and responsible AI models for commercial use. In addition, the integrated experience takes advantage of the capabilities of both platforms to simplify MLOps, data storage, and real-time processing.
For more information about using FMs in SageMaker JumpStart, refer to Train, deploy, and evaluate pretrained models with SageMaker JumpStart, JumpStart Foundation Models, and Getting started with Amazon SageMaker JumpStart.
Explore Bria models in SageMaker JumpStart today and revolutionize your visual content creation process!
About the Authors
Bar Fingerman is the Head of AI/ML Engineering at Bria. He leads the development and optimization of core infrastructure, enabling the company to scale cutting-edge generative AI technologies. With a focus on designing high-performance supercomputers for large-scale AI training, Bar leads the engineering group in deploying, managing, and securing scalable AI/ML cloud solutions. He works closely with leadership and cross-functional teams to align business goals while driving innovation and cost-efficiency.
Supriya Puragundla is a Senior Solutions Architect at AWS. She has over 15 years of IT experience in software development, design, and architecture. She helps key customer accounts on their data, generative AI, and AI/ML journeys. She is passionate about data-driven AI and the area of depth in ML and generative AI.
Rodrigo Merino is a Generative AI Solutions Architect Manager at AWS. With over a decade of experience deploying emerging technologies, ranging from generative AI to IoT, Rodrigo guides customers across various industries to accelerate their AI/ML and generative AI journeys. He specializes in helping organizations train and build models on AWS, as well as operationalize end-to-end ML solutions. Rodrigo’s expertise lies in bridging the gap between cutting-edge technology and practical business applications, enabling companies to harness the full potential of AI and drive innovation in their respective fields.
Eliad Maimon is a Senior Startup Solutions Architect at AWS, focusing on generative AI startups. He helps startups accelerate and scale their AI/ML journeys by guiding them through deep-learning model training and deployment on AWS. With a passion for AI and entrepreneurship, Eliad is committed to driving innovation and growth in the startup ecosystem.