Despite the proliferation of information and data in business environments, employees and stakeholders often find themselves searching for information and struggling to get their questions answered quickly and efficiently. This can lead to productivity losses, frustration, and delays in decision-making.
A generative AI Slack chat assistant can help address these challenges by providing a readily available, intelligent interface for users to interact with and obtain the information they need. By using the natural language processing and generation capabilities of generative AI, the chat assistant can understand user queries, retrieve relevant information from various data sources, and provide tailored, contextual responses.
By harnessing the power of generative AI and Amazon Web Services (AWS) services Amazon Bedrock, Amazon Kendra, and Amazon Lex, this solution provides a sample architecture to build an intelligent Slack chat assistant that can streamline information access, enhance user experiences, and drive productivity and efficiency within organizations.
Why use Amazon Kendra for building a RAG application?
Amazon Kendra is a fully managed service that provides out-of-the-box semantic search capabilities for state-of-the-art ranking of documents and passages. You can use Amazon Kendra to quickly build high-accuracy generative AI applications on enterprise data and source the most relevant content and documents to maximize the quality of your Retrieval Augmented Generation (RAG) payload, yielding better large language model (LLM) responses than using conventional or keyword-based search solutions. Amazon Kendra offers simple-to-use deep learning search models that are pre-trained on 14 domains and don’t require machine learning (ML) expertise. Amazon Kendra can index content from a wide range of sources, including databases, content management systems, file shares, and web pages.
Further, the FAQ feature in Amazon Kendra complements the broader retrieval capabilities of the service, allowing the RAG system to seamlessly switch between providing prewritten FAQ responses and dynamically generating responses by querying the larger knowledge base. This makes it well-suited for powering the retrieval component of a RAG system, allowing the model to access a broad knowledge base when generating responses. By integrating the FAQ capabilities of Amazon Kendra into a RAG system, the model can use a curated set of high-quality, authoritative answers for commonly asked questions. This can improve the overall response quality and user experience, while also reducing the burden on the language model to generate these basic responses from scratch.
This solution balances retaining customizations in terms of model selection, prompt engineering, and adding FAQs with not having to deal with word embeddings, document chunking, and other lower-level complexities typically required for RAG implementations.
Solution overview
The chat assistant is designed to assist users by answering their questions and providing information on a variety of topics. The purpose of the chat assistant is to be an internal-facing Slack tool that can help employees and stakeholders find the information they need.
The architecture uses Amazon Lex for intent recognition, AWS Lambda for processing queries, Amazon Kendra for searching through FAQs and web content, and Amazon Bedrock for generating contextual responses powered by LLMs. By combining these services, the chat assistant can understand natural language queries, retrieve relevant information from multiple data sources, and provide humanlike responses tailored to the user’s needs. The solution showcases the power of generative AI in creating intelligent virtual assistants that can streamline workflows and enhance user experiences based on model choices, FAQs, and modifying system prompts and inference parameters.
Architecture diagram
The following diagram illustrates a RAG approach where the user sends a query through the Slack application and receives a generated response based on the data indexed in Amazon Kendra. In this post, we use Amazon Kendra Web Crawler as the data source and include FAQs stored on Amazon Simple Storage Service (Amazon S3). See Data source connectors for a list of supported data source connectors for Amazon Kendra.
The step-by-step workflow for the architecture is the following:
- The user sends a query such as
What is the AWS Well-Architected Framework?
through the Slack app. The query goes to Amazon Lex, which identifies the intent. Currently two intents are configured in Amazon Lex (Welcome
and FallbackIntent
). The welcome intent is configured to respond with a greeting when a user enters a greeting such as “hi” or “hello.” The assistant responds with “Hello! I can help you with queries based on the documents provided. Ask me a question.” The fallback intent is fulfilled with a Lambda function. - The Lambda function searches Amazon Kendra FAQs through the
search_Kendra_FAQ
method by taking the user query and Amazon Kendra index ID as inputs. If there’s a match with a high confidence score, the answer from the FAQ is returned to the user. If there isn’t a match with a high enough confidence score, relevant documents from Amazon Kendra with a high confidence score are retrieved through the kendra_retrieve_document
method and sent to Amazon Bedrock to generate a response as the context. The response is generated from Amazon Bedrock with the invokeLLM
method. The following is a snippet of the invokeLLM
method within the fulfillment function. Read more on inference parameters and system prompts to modify parameters that are passed into the Amazon Bedrock invoke model request. Finally, the response generated from Amazon Bedrock along with the relevant referenced URLs are returned to the end user. When selecting websites to index, adhere to the AWS Acceptable Use Policy and other AWS terms. Remember that you can only use Amazon Kendra Web Crawler to index your own web pages or web pages that you have authorization to index. Visit the Amazon Kendra Web Crawler data source guide to learn more about using the web crawler as a data source. Using Amazon Kendra Web Crawler to aggressively crawl websites or web pages you don’t own is not considered acceptable use.
Supported features
The chat assistant supports the following features:
- Support for the following Anthropic’s models on Amazon Bedrock:
claude-v2
claude-3-haiku-20240307-v1:0
claude-instant-v1
claude-3-sonnet-20240229-v1:0
VERY_HIGH
Retrieves only documents from Amazon Kendra that have a HIGH
or VERY_HIGH
confidence score If documents with a high confidence score aren’t found, the chat assistant returns “No relevant documents found” Prerequisites
To perform the solution, you need to have following prerequisites:
- Basic knowledge of AWS An AWS account with access to Amazon S3 and Amazon Kendra An S3 bucket to store your documents. For more information, see Step 1: Create your first S3 bucket and the Amazon S3 User Guide. A Slack workspace to integrate the chat assistant Permission to install Slack apps in your Slack workspace Seed URLs for the Amazon Kendra Web Crawler data source
- You’ll need authorization to crawl and index any websites provided
Build a generative AI Slack chat assistant
To build a Slack application, use the following steps:
- Request model access on Amazon Bedrock for all Anthropic models Create an S3 bucket in the
us-east-1
(N. Virginia) AWS Region. Upload the AIBot-LexJson.zip and SampleFAQ.csv files to the S3 bucket Launch the CloudFormation stack in the us-east-1
(N. Virginia) AWS Region.
SampleFAQs
uploaded to the S3 bucket in Step 3 For S3LexBotKey, enter the name of the Amazon Lex .zip file uploaded to the S3 bucket in Step 3 For SeedUrls, enter up to 10 URLs for the web crawler as a comma delimited list. In the example in this post, we give the publicly available Amazon Bedrock service page as the seed URL Leave the rest as defaults. Choose Next. Choose Next again on the Configure stack options Acknowledge by selecting the box and choose Submit, as shown in the following screenshot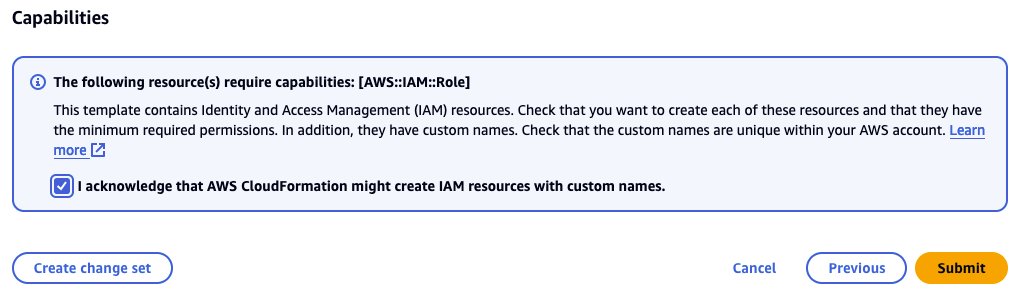
- On the Amazon Lex console, choose your chat assistant
${YourStackName}-AIBot
Choose Intents Choose Version 1 and choose Test, as shown in the following screenshot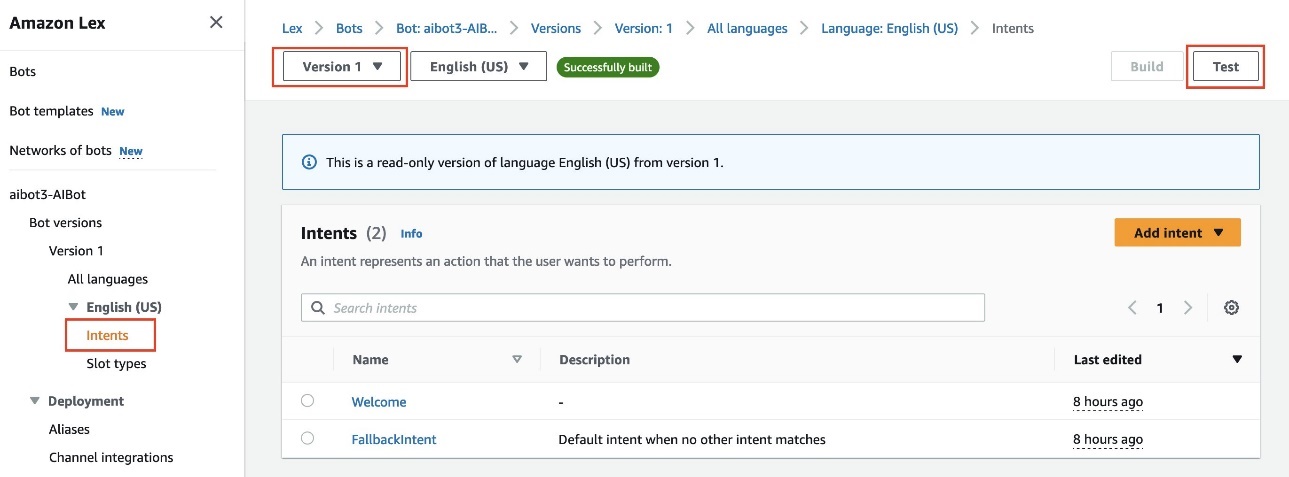
AIBotProdAlias
. Learn more about Versioning and Aliases.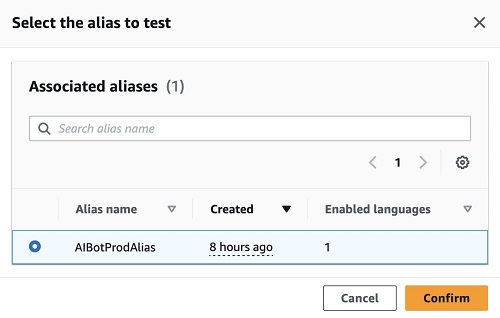
_question | _answer | _source_uri |
Which AWS service has 11 nines of durability? | Amazon S3 | https://aws.amazon.com/s3/ |
What is the AWS Well-Architected Framework? | The AWS Well-Architected Framework enables customers and partners to review their architectures using a consistent approach and provides guidance to improve designs over time. | https://aws.amazon.com/architecture/well-architected/ |
In what Regions is Amazon Kendra available? | Amazon Kendra is currently available in the following AWS Regions: Northern Virginia, Oregon, and Ireland | https://aws.amazon.com/about-aws/global-infrastructure/regional-product-services/ |
“Which AWS service has 11 nines of durability?”
and its response. You can observe that the response is the same as in the FAQ file and includes a link.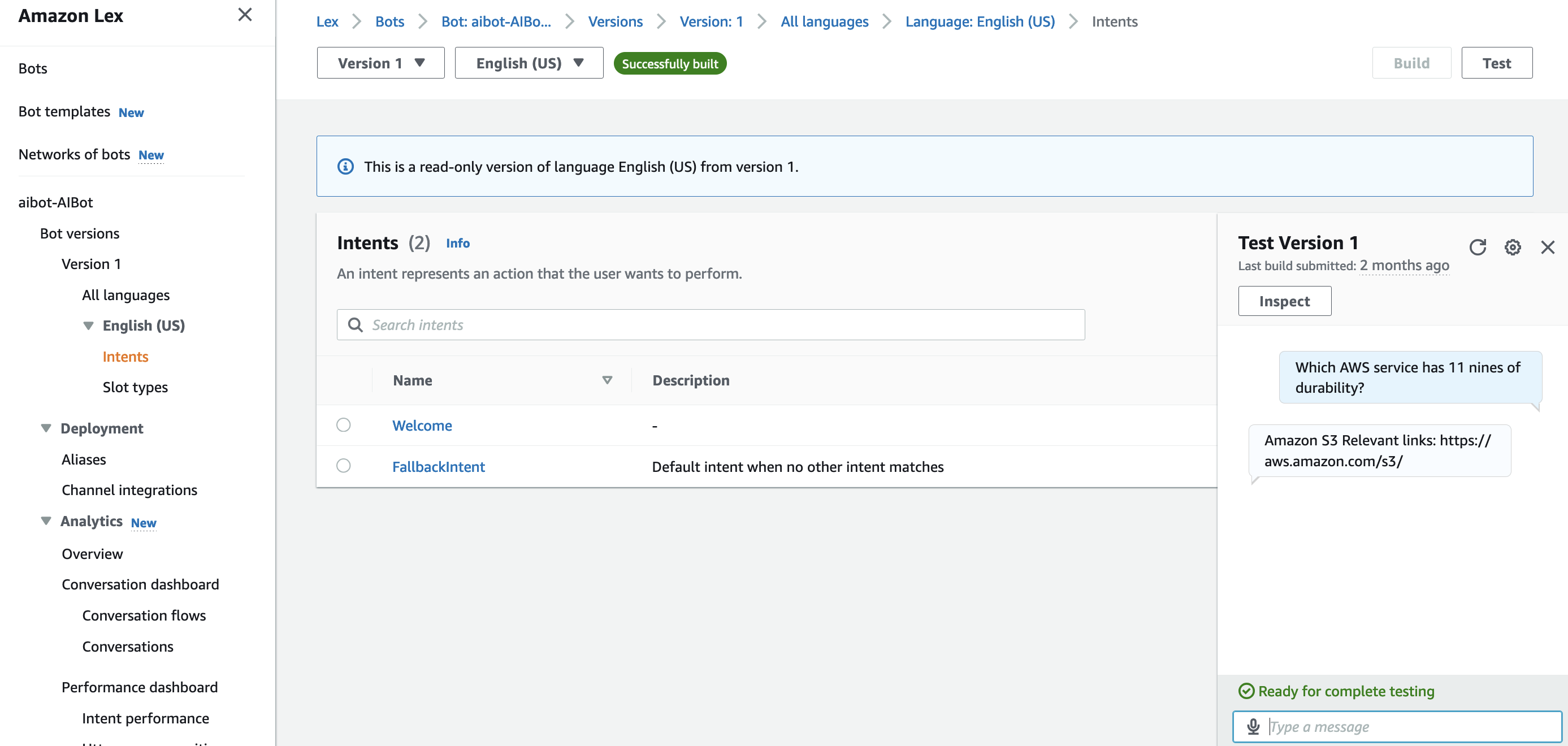
“What are agents in Amazon Bedrock?”
and and a generated response that includes relevant links.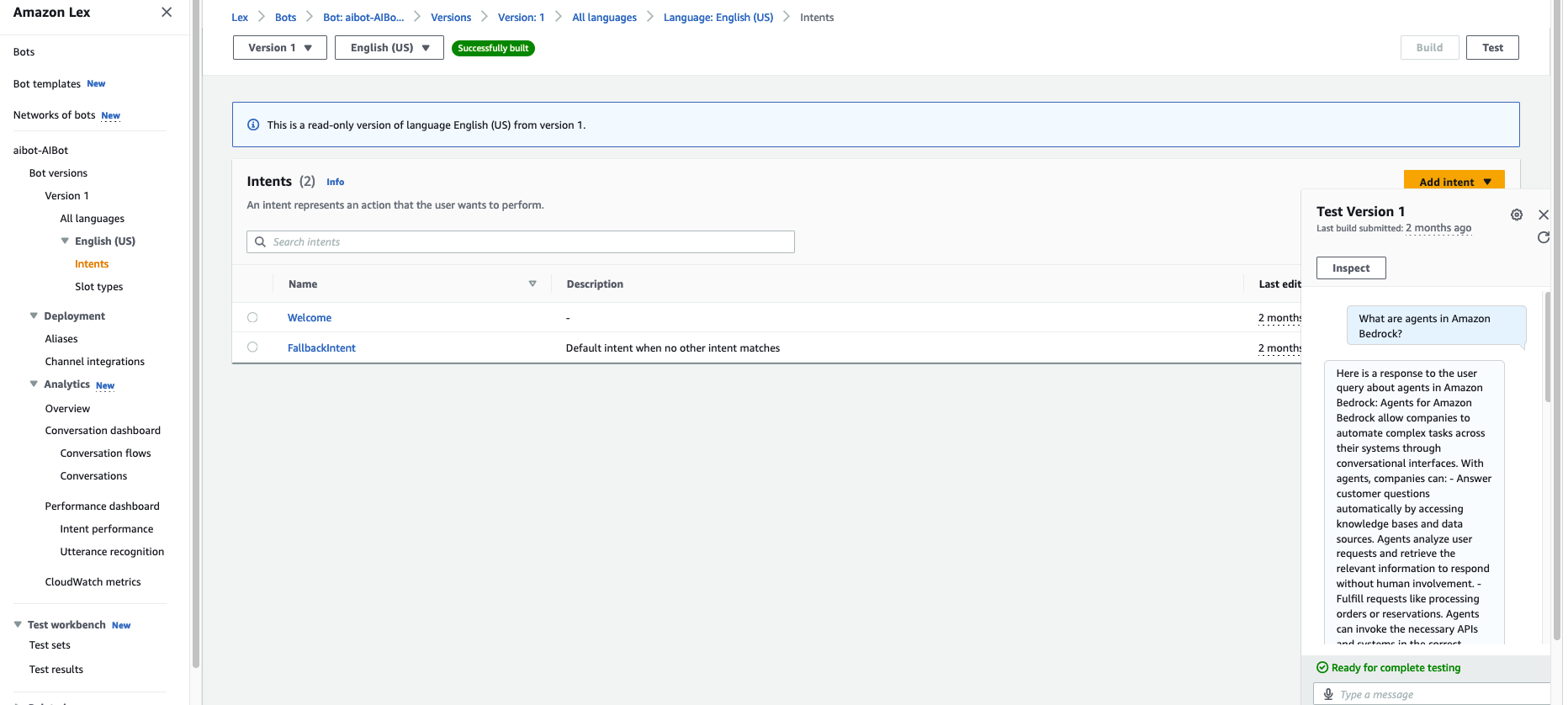
- For integration of the Amazon Lex chat assistant with Slack, see Integrating an Amazon Lex V2 bot with Slack. Choose the AIBotProdAlias under Alias in the Channel Integrations
Run sample queries to test the solution
- In Slack, go to the Apps section. In the dropdown menu, choose Manage and select Browse apps.
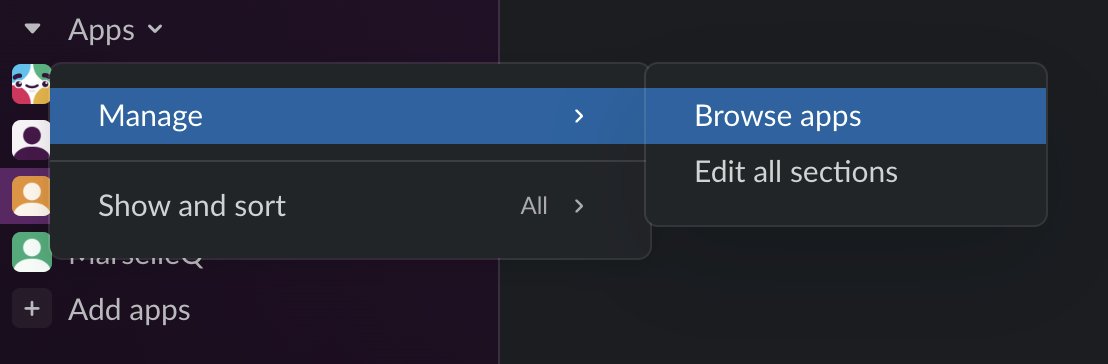
${AIBot}
in App Directory and choose the chat assistant. This will add the chat assistant to the Apps section in Slack. You can now start asking questions in the chat. The following screenshot shows the question “Which AWS service has 11 nines of durability?”
and its response. You can observe that the response is the same as in the FAQ file and includes a link.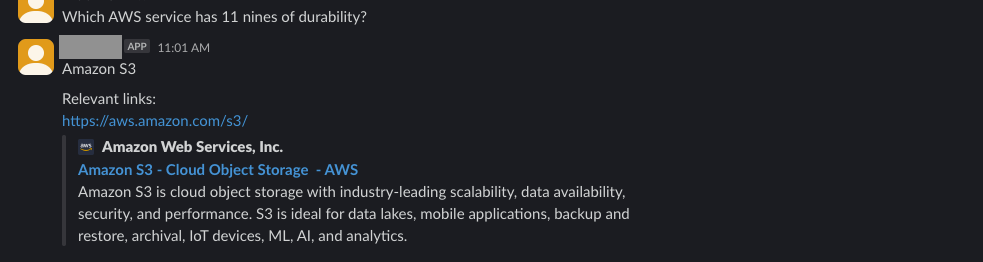
“What is the AWS Well-Architected Framework?”
and its response.
“What are agents in Amazon Bedrock?”
and and a generated response that includes relevant links.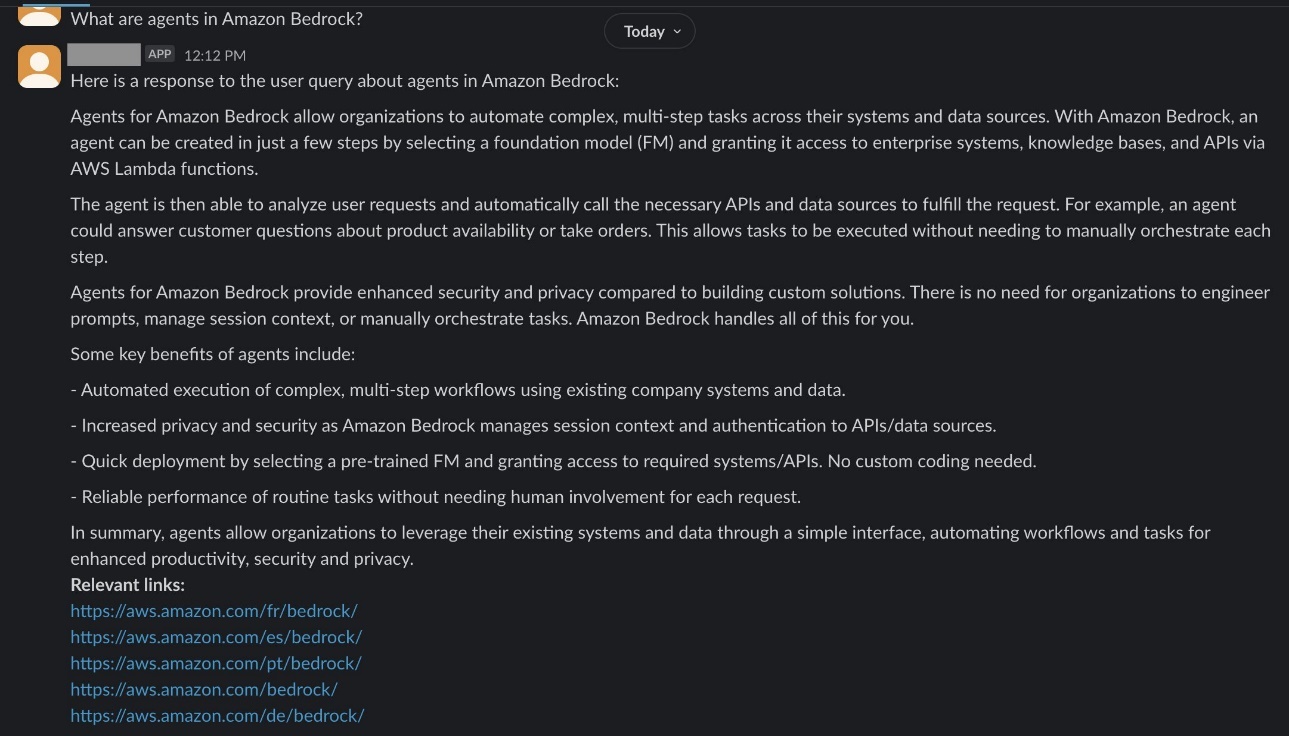
“What is amazon polly?”
Because there is no Amazon Polly documentation indexed, the chat assistant responds with “No relevant documents found,” as expected.
These examples show how the chat assistant retrieves documents from Amazon Kendra and provides answers based on the documents retrieved. If no relevant documents are found, the chat assistant responds with “No relevant documents found.”
Clean up
To clean up the resources created by this solution:
- Delete the CloudFormation stack by navigating to the CloudFormation console Select the stack you created for this solution and choose Delete Confirm the deletion by entering the stack name in the provided field. This will remove all the resources created by the CloudFormation template, including the Amazon Kendra index, Amazon Lex chat assistant, Lambda function, and other related resources.
Conclusion
This post describes the development of a generative AI Slack application powered by Amazon Bedrock and Amazon Kendra. This is designed to be an internal-facing Slack chat assistant that helps answer questions related to the indexed content. The solution architecture includes Amazon Lex for intent identification, a Lambda function for fulfilling the fallback intent, Amazon Kendra for FAQ searches and indexing crawled web pages, and Amazon Bedrock for generating responses. The post walks through the deployment of the solution using a CloudFormation template, provides instructions for running sample queries, and discusses the steps for cleaning up the resources. Overall, this post demonstrates how to use various AWS services to build a powerful generative AI–powered chat assistant application.
This solution demonstrates the power of generative AI in building intelligent chat assistants and search assistants. Explore the generative AI Slack chat assistant: Invite your teams to a Slack workspace and start getting answers to your indexed content and FAQs. Experiment with different use cases and see how you can harness the capabilities of services like Amazon Bedrock and Amazon Kendra to enhance your business operations. For more information about using Amazon Bedrock with Slack, refer to Deploy a Slack gateway for Amazon Bedrock.
About the authors
Kruthi Jayasimha Rao is a Partner Solutions Architect with a focus on AI and ML. She provides technical guidance to AWS Partners in following best practices to build secure, resilient, and highly available solutions in the AWS Cloud.
Mohamed Mohamud is a Partner Solutions Architect with a focus on Data Analytics. He specializes in streaming analytics, helping partners build real-time data pipelines and analytics solutions on AWS. With expertise in services like Amazon Kinesis, Amazon MSK, and Amazon EMR, Mohamed enables data-driven decision-making through streaming analytics.