The rapid adoption of generative AI (GenAI) applications is driving a seismic shift within the SaaS application ecosystem. As enterprises leverage more GenAI, they face the formidable challenge of managing and safeguarding massive volumes of data. Artificial intelligence (AI) not only transforms workforce productivity, but it also plays a critical role in powering data security, particularly with classification. The performance and cost-effectiveness of NVIDIA’s full-stack accelerated computing provides the computational power and AI software deployment tools needed to secure vast amounts of data effectively.
Prasidh Srikanth, Senior Director of Product Management, Data Security, Palo Alto Networks said:
Today’s enterprises face increased challenges in securing their data, largely driven by the massive volume and complexity of diverse data formats. That’s why Palo Alto Networks is using NVIDIA’s groundbreaking GPU and Triton technology to improve the efficiency and accuracy of our DLP machine learning models, leading to faster response times and better customer outcomes. Together with NVIDIA, we’re providing enterprises with the best in class security and the most advanced AI technology needed to protect their modern workforce.
Chris Arangio, Cybersecurity Developer Relations Lead, NVIDIA said:
As enterprises harness the power of generative AI, the need for robust data security scales exponentially. With NVIDIA accelerated computing and AI software, cybersecurity leaders like Palo Alto Networks can safeguard vast amounts of sensitive information with unprecedented speed and accuracy, ushering in a new era of AI-driven data protection.
At Palo Alto Networks, we understand that traditional data loss prevention (DLP) alone is unable to keep up with the demands of today’s modern connected enterprise. That’s why we’re leveraging best-in-class NVIDIA accelerated computing, which includes the NVIDIA AI Enterprise platform for the development and deployment of enterprise co-pilots and other GenAI apps. This powers our AI models for enhanced data discovery and classification for superior customer outcomes. As we like to say, we’re Securing AI by Design.
Challenges in Data Discovery and Classification
Traditional DLP methods are increasingly outpaced by the complexities of today’s digital environment. The modern landscape is marked by an explosion of new data types, formats and sources, alongside an unprecedented volume of sensitive information, all of which pose significant challenges to effective data security. Data sprawl can encompass a vast array of formats, including image files, documents with intricate layouts, proprietary datasets and dynamic web content.
One of the most daunting challenges in modern data protection is detecting sensitive data within complex data types. Traditional detection methods fall short in capturing the subtle and nuanced natural language contexts in which sensitive information resides. Sensitive data embedded within visual formats, such as scanned documents or images, can prove evasive due to the inherent complexities of visual data processing.
False positives further compound these challenges. Traditional techniques frequently misidentify benign data as sensitive, resulting in unnecessary alerts and wasted resources. This issue can lead to alert fatigue, where critical notifications are overlooked and can disrupt business operations by inadvertently blocking legitimate data transfers. Addressing these challenges requires a more sophisticated approach that leverages context-aware detection technologies to enhance accuracy and reduce false positives.
Enhancing Detection Capabilities with Machine Learning
At Palo Alto Networks, we employ advanced AI and machine learning (ML) models to address the aforementioned challenges through a sophisticated, three-phased approach:
- Augmenting existing detection capabilities with AI and ML.Utilizing generative AI for synthetic data creation.Increasing accuracy with LLM and context-aware detections.
Augmenting Existing Detection Capabilities with AI and ML
Regulated industries, like healthcare, often require the detection of unique documents that contain sensitive patient information. To meet these requirements, we provide trainable classifiers that excel in identifying specific data types. Classifiers can be specifically trained with labels to accurately recognize and categorize patient records, like personally identifiable information (PII), patient profiles, medical diagnosis reports and prescription data.
Palo Alto Networks ML-based detections also include optical character recognition (OCR). OCR technology is pivotal for identifying sensitive information embedded within image files, such as scanned documents like driver's licenses, passports and other forms of PII. Machine learning enhances OCR by training algorithms on extensive image datasets to improve text detection and recognition. This process involves preprocessing images to enhance its detection quality using deep learning models to accurately identify and interpret characters and words, while applying postprocessing techniques to further refine the output.
Utilizing Generative AI for Synthetic Data Creation
Large language models (LLMs), such as OpenAI o1 or GPT-4o, have demonstrated remarkable abilities to understand and generate text. With just a few examples, LLMs can create diverse datasets that can be used to help build robust ML models. This is particularly useful for datasets that historically contain very limited samples, such as EU driver's licenses and national IDs. The ability to generate synthetic data has proven to be highly effective in training our AI/ML detection models.
Increasing Accuracy with LLM and Context-Aware Detections
Palo Alto Networks Data Security addresses the issue of false positives that have often plagued data security administrators. Regular expression patterns and keyword-based methods are inherently prone to generating false positives, which can overwhelm security teams and dilute the effectiveness of data protection efforts. To mitigate this, we leverage LLM-powered ML models to provide context of the detected sensitive data and establish ground truth.
In the example below, a regular expression might mistakenly flag any 9-digit number as a Social Security number. However, by using context-aware ML detection, we significantly enhance the signal-to-noise ratio. LLM-powered improvements have led to a remarkable reduction in potential false positives by over 90%. This is achieved through advanced pattern recognition that understands the context and semantics of data. With advanced ML, we can accurately differentiate between true positives and benign data, empowering InfoSec teams to respond to real incidents.
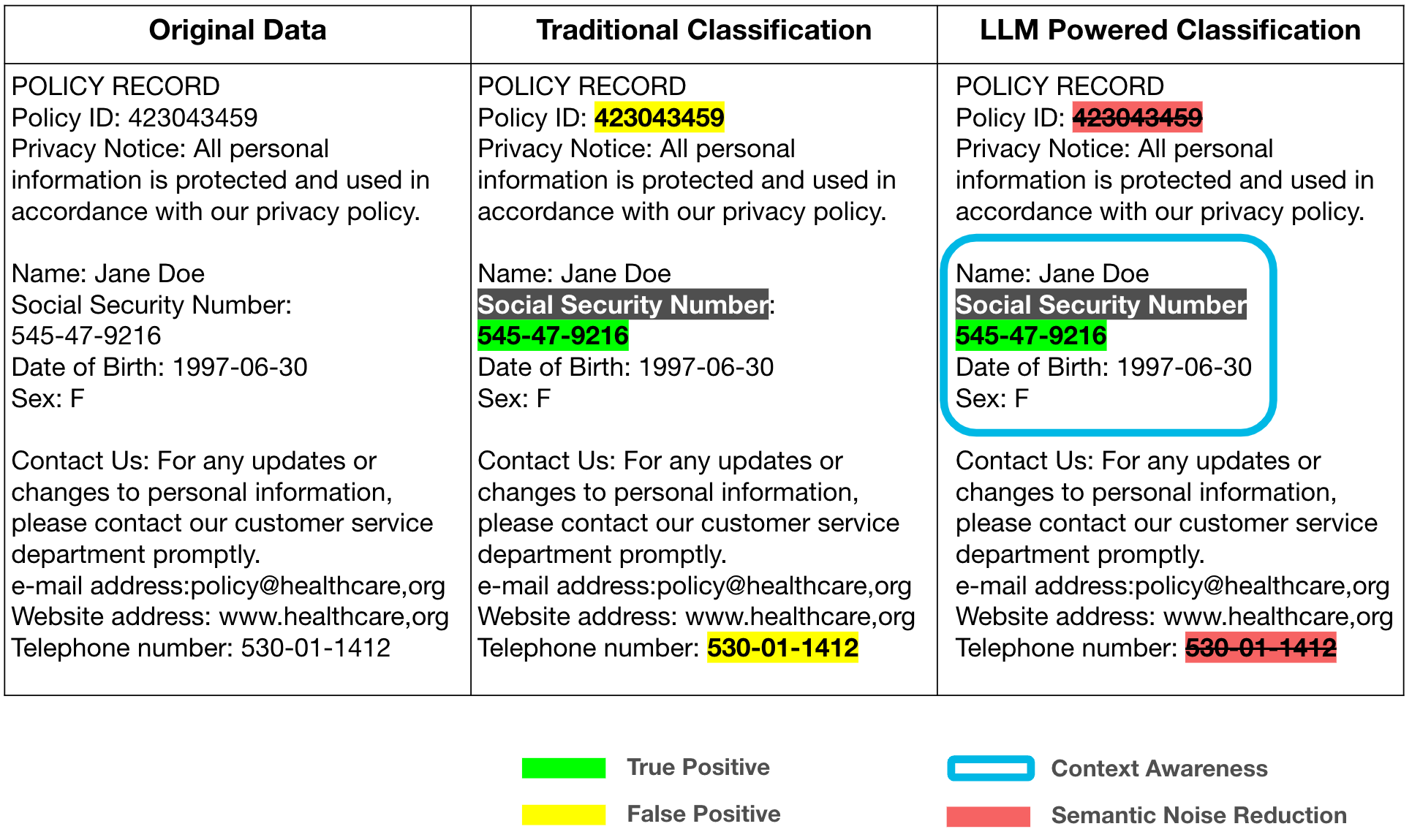
The Role of NVIDIA AI in Machine Learning
Computational power is essential to effectively train and deploy deep learning models. Two critical factors in deciding whether to host on a GPU are cost and response time. We have consistently found that transitioning to GPUs, especially for more computationally intensive models, not only reduces costs but significantly decreases response times compared to hosting the same models on CPUs with alternative inferencing hardware.
Our GPU deployment models have been aided by NVIDIA Triton Inference Server, part of NVIDIA AI Enterprise, providing a dynamic interface and allowing for the hosting of various kinds of models.
Performance Enhancement: Triton boosts throughput by 20% for both CPU and GPU hosting. This is achieved through dynamic batch processing and optimal utilization of GPU cores, leading to faster response times and more efficient use of computational resources.
- Dynamic Batch Processing: Triton intelligently manages batch processing based on server load, enabling more efficient handling of multiple requests simultaneously.Optimal GPU Utilization: Triton maximizes the usage of GPU resources, ensuring higher overall GPU efficiency by dynamically allocating GPU resources to handle various tasks in parallel, leading to reduced response times and increased processing speed.
Below is a performance table comparing the model inferencing key performance metrics across instances using CPU with Inferentia, NVIDIA GPU and NVIDIA GPU with Triton. The data demonstrates a substantial reduction in response time with GPU and Triton-based instances, showcasing the efficiency gains achieved through advanced GPU hosting and optimization with Triton.
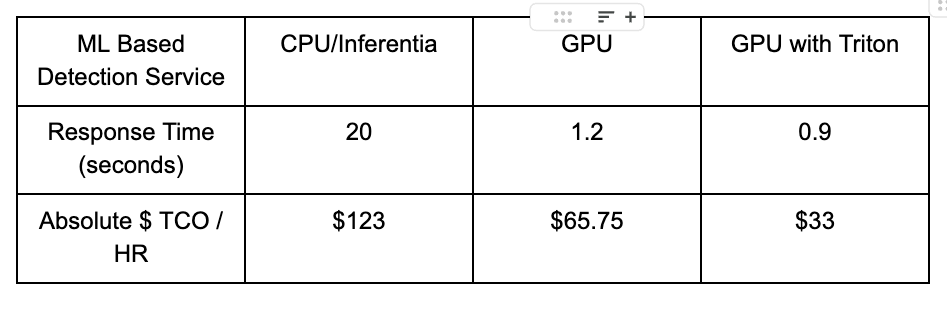
Cost Efficiency: Triton improves performance and drives down operational costs. By optimizing GPU usage and repurposing excess CPU resources, Triton enables substantial cost savings, making it a highly cost-efficient solution for deploying and managing machine learning models.
The chart below illustrates both the compute cost per hour and the response time (adjusted to deciseconds for clarity). The key insights are:
- CPU with Inferentia Service: The response time is notably high and running parallel computations on the CPU is costly.GPU without Optimization: Switching to a GPU significantly reduces the response time compared to the CPU.GPU with Triton Optimization: Both response time and cost are optimized, bringing the compute cost down to $33 per hour.
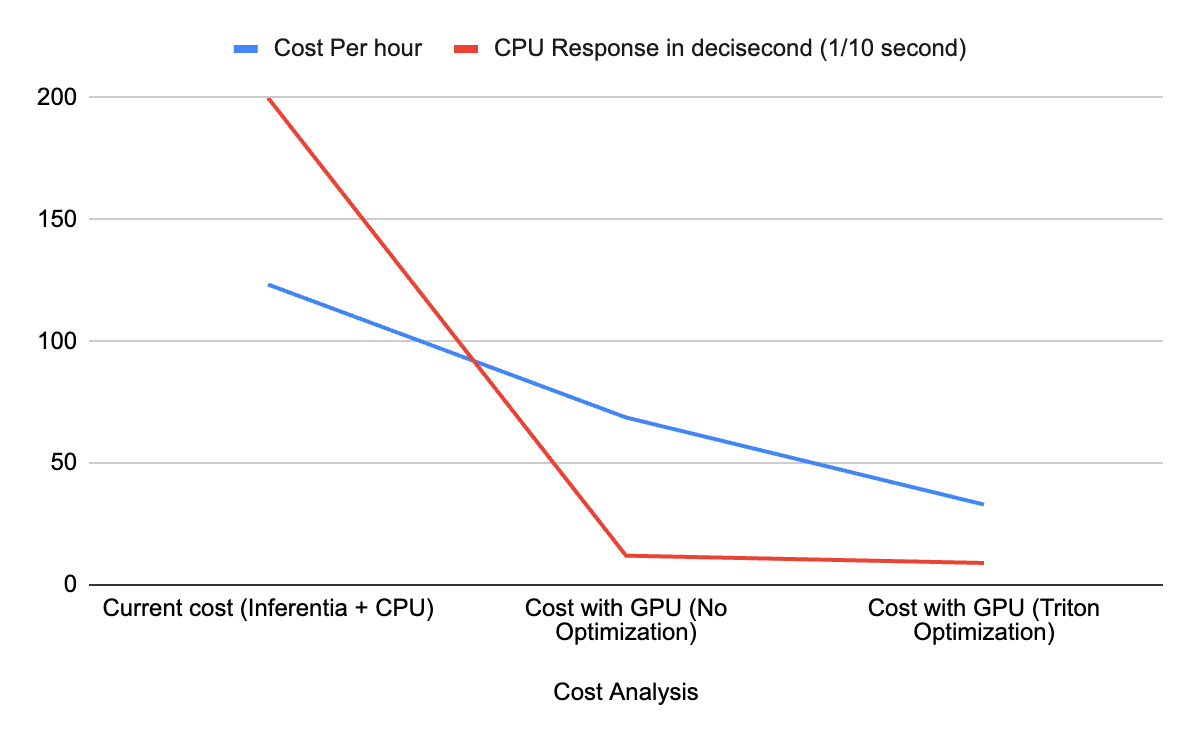
Conclusion
The integration of NVIDIA Triton Inference Server and GPU technology into Palo Alto Networks Data Security marks a significant advancement in our ability to handle complex data security challenges. By leveraging the exceptional computational power of NVIDIA accelerated computing, we have dramatically improved the efficiency and accuracy of our ML models, leading to faster response times and a substantial reduction in operational costs. Triton Inference Server optimizes GPU utilization and enhances throughput, enabling us to scale our services more effectively while maintaining cost-efficiency. As we continue to expand our capabilities, the use of NVIDIA AI and accelerated computing will play a pivotal role in driving the future of AI-powered data protection, ensuring our solutions remain at the cutting edge of innovation.
Contact your Palo Alto Networks representative to explore how our integrated data security solution can empower your business to thrive in today’s dynamic digital landscape.
The post Palo Alto Networks Prevents Data Loss at Enterprise Scale with NVIDIA appeared first on Palo Alto Networks Blog.